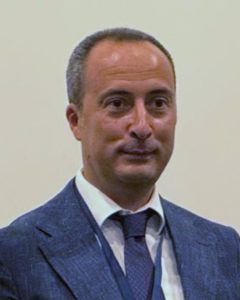
Informazioni
+39 080 596 3052
ti.ab1738079269ilop@1738079269ilrac1738079269.elea1738079269ffar1738079269
Pagina Istituzionale
Pagina Google Scholar
Pagina Scopus
Curriculum Vitae
Pubblicazioni
Raffaele CARLI
Ricercatore
Raffaele Carli ha conseguito la Laurea in Ingegneria Elettronica con lode nel 2002 e il Dottorato di Ricerca in Ingegneria Elettrica e dell’Informazione nel 2016, entrambi presso il Politecnico di Bari, Italia. Dal 2003 al 2004 ha prestato servizio come Ufficiale di Complemento nella Marina Militare Italiana. Dal 2004 al 2012 ha lavorato come System and Control Engineer and Technical Manager per una multinazionale nel settore spaziale e della difesa.
Attualmente, il Dr. Carli è Ricercatore (RTD-b) in Automatica presso il Politecnico di Bari, dove è responsabile tecnico del Decision and Control Laboratory (coordinato dalla prof.ssa Mariagrazia Dotoli) all’interno del Dipartimento di Ingegneria Elettrica e dell’Informazione (http://dclab.poliba.it/). Ha conseguito l’abilitazione nazionale come Professore Associato nel 2021 e come Professore Ordinario nel 2023. Dal 2022 è Vice-Coordinatore del Dottorato Nazionale in Sistemi Autonomi (http://dausy.poliba.it/phd/).
Il Dr. Carli fa parte del comitato editoriale delle riviste IEEE (Institute of Electrical and Electronics Engineers) come Associate Editor per IEEE Transactions on Automation Science and Engineering (premiato come Best Associate Editor nel 2023) e IEEE Transactions on Systems, Man, and Cybernetics. È inoltre un membro attivo dei comitati editoriali di conferenze per vari eventi sponsorizzati da IFAC (International Federation of Automatic Control) e IEEE, inclusi quelli promossi dalla IEEE Control Systems Society (CSS), dalla IEEE Robotics and Automation Society (RAS) e dalla IEEE Systems, Man, and Cybernetics Society (SMCS). I suoi ruoli organizzativi comprendono la partecipazione ai comitati di diverse conferenze IEEE, tra cui Young Career Chair per la IEEE International Conference on Automation Science and Engineering del 2017, Publication Co-chair per la IEEE International Conference on Automation Science and Engineering del 2015, Tutorial Co-chair per la IEEE International Conference on Systems, Man and Cybernetics del 2023, e Finance Chair e Special Session Chair per la IEEE International Conference on Automation Science and Engineering del 2024. Riveste inoltre il ruolo di General Chair per il prossimo IFAC Workshop on Smart Energy Systems for Efficient and Sustainable Smart Grids and Smart Cities (SENSYS 2025).
Membro Senior IEEE dal 2022, il Dr. Carli attualmente ricopre il ruolo di Mentorship Subcommittee Chair del Membership and Student Activities Committee all’interno della IEEE SMCS per per i bienni 2023-2024 e 2025-2026. Inoltre, è Segretario del Chapter CS23 della Control Systems Society (CSS) dell’IEEE Italy Section per il mandato 2025-2026.
Il Dr. Carli è autore di oltre 110 pubblicazioni internazionali (profilo Google Scholar: https://scholar.google.it/citations?user=OvXT8Y0AAAAJ&hl=en). La sua ricerca si concentra sullo sviluppo di tecniche di decisione e controllo per la modellazione, ottimizzazione, gestione e controllo di sistemi complessi su larga scala, in particolare in ambiti intelligenti come l’industria e l’energia. Ha ricevuto il premio IEEE Italy Section SMCS Chapter Award for Excellence in Early Career Research nel 2024.
Pubblicazioni
2024
- Scarabaggio, P., Carli, R., Grammatico, S. & Dotoli, M. (2024) Local Generalized Nash Equilibria with Nonconvex Coupling Constraints. IN IEEE Transactions on Automatic Control, .. doi:10.1109/TAC.2024.3462553
[BibTeX] [Abstract] [Download PDF]We address a class of Nash games with nonconvex coupling constraints for which we define a novel notion of local equilibrium, here named local generalized Nash equilibrium (LGNE). Our first technical contribution is to show the stability in the game theoretic sense of these equilibria on a specific local subset of the original feasible set. Remarkably, we show that the proposed notion of local equilibrium can be equivalently formulated as the solution of a quasi-variational inequality with equal Lagrange multipliers. Next, under the additional proximal smoothness assumption of the coupled feasible set, we define conditions for the existence and local uniqueness of a LGNE. To compute such an equilibrium, we propose two discrete-time dynamics, or fixed-point iterations implemented in a centralized fashion. Our third technical contribution is to prove convergence under (strongly) monotone assumptions on the pseudo- gradient mapping of the game and proximal smoothness of the coupled feasible set. Finally, we apply our theoretical results to a noncooperative version of the optimal power flow control problem. © 1963-2012 IEEE.
@ARTICLE{Scarabaggio2024, author = {Scarabaggio, Paolo and Carli, Raffaele and Grammatico, Sergio and Dotoli, Mariagrazia}, title = {Local Generalized Nash Equilibria with Nonconvex Coupling Constraints}, year = {2024}, journal = {IEEE Transactions on Automatic Control}, doi = {10.1109/TAC.2024.3462553}, url = {https://www.scopus.com/inward/record.uri?eid=2-s2.0-85204423506&doi=10.1109%2fTAC.2024.3462553&partnerID=40&md5=a06e7fdba1764046b6ce415201f6be5e}, abstract = {We address a class of Nash games with nonconvex coupling constraints for which we define a novel notion of local equilibrium, here named local generalized Nash equilibrium (LGNE). Our first technical contribution is to show the stability in the game theoretic sense of these equilibria on a specific local subset of the original feasible set. Remarkably, we show that the proposed notion of local equilibrium can be equivalently formulated as the solution of a quasi-variational inequality with equal Lagrange multipliers. Next, under the additional proximal smoothness assumption of the coupled feasible set, we define conditions for the existence and local uniqueness of a LGNE. To compute such an equilibrium, we propose two discrete-time dynamics, or fixed-point iterations implemented in a centralized fashion. Our third technical contribution is to prove convergence under (strongly) monotone assumptions on the pseudo- gradient mapping of the game and proximal smoothness of the coupled feasible set. Finally, we apply our theoretical results to a noncooperative version of the optimal power flow control problem. © 1963-2012 IEEE.}, author_keywords = {Generalized nash equilibrium; multi-agent systems; non convex generalized games; variational inequalities}, keywords = {Game theory; Coupling constraints; Feasible set; Generalized Nash equilibrium; Local equilibrium; Multiagent systems (MASs); Nash games; Non convex generalized game; Nonconvex; Technical contribution; Variational inequalities; Lagrange multipliers}, type = {Article}, publication_stage = {Article in press}, source = {Scopus}, note = {Cited by: 0; All Open Access, Hybrid Gold Open Access} }
- Dammacco, L., Carli, R., Lazazzera, V., Fiorentino, M. & Dotoli, M. (2024) Virtual Design of Complex Manufacturing Systems by Digital Technologies: The Case of an Italian Automotive Company. IN Lecture Notes in Mechanical Engineering, .383 – 390. doi:10.1007/978-3-031-58094-9_42
[BibTeX] [Abstract] [Download PDF]In recent years, many industrial companies have embraced new technologies for Industry 4.0, the fourth industrial revolution. This global manufacturing trend connects real-life industry with the virtual world. Digitalization tools play a crucial role in allowing companies to decrease design and production costs, as well as accelerate the product development process. Regarding an actual industrial case study of an Italian automotive company, this work focuses on the use of digital technologies in designing complex manufacturing systems, showing the benefits that can be obtained with respect to traditional methods. Digitalization tools have aided in the design of intricate manufacturing systems that demand advanced automation to optimize the arrangement, workflow, and equipment configuration within a manufacturing facility or production line. After examining the features of the virtual approach employed by the considered company, the virtual commissioning of a screwing station for an electric axle assembly line is analyzed in detail to show the efficiency and effectiveness of digitalization. © The Author(s), under exclusive license to Springer Nature Switzerland AG 2024.
@ARTICLE{Dammacco2024383, author = {Dammacco, Lucilla and Carli, Raffaele and Lazazzera, Vito and Fiorentino, Michele and Dotoli, Mariagrazia}, title = {Virtual Design of Complex Manufacturing Systems by Digital Technologies: The Case of an Italian Automotive Company}, year = {2024}, journal = {Lecture Notes in Mechanical Engineering}, pages = {383 – 390}, doi = {10.1007/978-3-031-58094-9_42}, url = {https://www.scopus.com/inward/record.uri?eid=2-s2.0-85193606990&doi=10.1007%2f978-3-031-58094-9_42&partnerID=40&md5=f032f88abf2548dcd8fabb0be4cd138a}, abstract = {In recent years, many industrial companies have embraced new technologies for Industry 4.0, the fourth industrial revolution. This global manufacturing trend connects real-life industry with the virtual world. Digitalization tools play a crucial role in allowing companies to decrease design and production costs, as well as accelerate the product development process. Regarding an actual industrial case study of an Italian automotive company, this work focuses on the use of digital technologies in designing complex manufacturing systems, showing the benefits that can be obtained with respect to traditional methods. Digitalization tools have aided in the design of intricate manufacturing systems that demand advanced automation to optimize the arrangement, workflow, and equipment configuration within a manufacturing facility or production line. After examining the features of the virtual approach employed by the considered company, the virtual commissioning of a screwing station for an electric axle assembly line is analyzed in detail to show the efficiency and effectiveness of digitalization. © The Author(s), under exclusive license to Springer Nature Switzerland AG 2024.}, author_keywords = {Automotive; Complex Manufacturing Systems; Digital Technologies; Industry 4.0; Virtual Commissioning}, keywords = {Costs; Electric lines; Product design; Virtual reality; Automotive companies; Automotives; Complex manufacturing systems; Digital technologies; Global manufacturing; Industrial companies; Industrial revolutions; Virtual commissioning; Virtual design; Virtual worlds; Industry 4.0}, type = {Conference paper}, publication_stage = {Final}, source = {Scopus}, note = {Cited by: 0} }
- Mignoni, N., Carli, R. & Dotoli, M. (2024) Optimal Decision Strategies for the Generalized Cuckoo Card Game. IN IEEE Transactions on Games, 16.185 – 194. doi:10.1109/TG.2023.3239795
[BibTeX] [Abstract] [Download PDF]Cuckoo is a popular card game, which originated in France during the 15th century, and then, spread throughout Europe, where it is currently well-known under distinct names and with different variants. Cuckoo is an imperfect information game-of-chance, which makes the research regarding its optimal strategies determination interesting. The rules are simple: each player receives a covered card from the dealer; starting from the player at the dealer’s left, each player looks at its own card and decides whether to exchange it with the player to their left, or keep it; the dealer plays at last and, if it decides to exchange card, it draws a random one from the remaining deck; the player(s) with the lowest valued card lose(s) the round. We formulate the gameplay mathematically and provide an analysis of the optimal decision policies. Different card decks can be used for this game, e.g., the standard 52-card deck or the Italian 40-card deck. We generalize the decision model for an arbitrary number of decks’ cards, suites, and players. Finally, through numerical simulations, we compare the determined optimal decision strategy against different benchmarks, showing that the strategy outperforms the random and naive policies and approaches the performance of the ideal oracle. © 2018 IEEE.
@ARTICLE{Mignoni2024185, author = {Mignoni, Nicola and Carli, Raffaele and Dotoli, Mariagrazia}, title = {Optimal Decision Strategies for the Generalized Cuckoo Card Game}, year = {2024}, journal = {IEEE Transactions on Games}, volume = {16}, number = {1}, pages = {185 – 194}, doi = {10.1109/TG.2023.3239795}, url = {https://www.scopus.com/inward/record.uri?eid=2-s2.0-85147283813&doi=10.1109%2fTG.2023.3239795&partnerID=40&md5=3913f77d7fa5cb1c10485170111b23d2}, abstract = {Cuckoo is a popular card game, which originated in France during the 15th century, and then, spread throughout Europe, where it is currently well-known under distinct names and with different variants. Cuckoo is an imperfect information game-of-chance, which makes the research regarding its optimal strategies determination interesting. The rules are simple: each player receives a covered card from the dealer; starting from the player at the dealer's left, each player looks at its own card and decides whether to exchange it with the player to their left, or keep it; the dealer plays at last and, if it decides to exchange card, it draws a random one from the remaining deck; the player(s) with the lowest valued card lose(s) the round. We formulate the gameplay mathematically and provide an analysis of the optimal decision policies. Different card decks can be used for this game, e.g., the standard 52-card deck or the Italian 40-card deck. We generalize the decision model for an arbitrary number of decks' cards, suites, and players. Finally, through numerical simulations, we compare the determined optimal decision strategy against different benchmarks, showing that the strategy outperforms the random and naive policies and approaches the performance of the ideal oracle. © 2018 IEEE.}, author_keywords = {Card games; games of chance; optimal decision strategy; optimization}, keywords = {Artificial intelligence; Benchmarking; Emotion Recognition; 15th century; Card games; Computational modelling; Cultural difference; Emotion recognition; Europe; Game; Games of chance; Optimal decision strategy; Optimisations; Numerical models}, type = {Article}, publication_stage = {Final}, source = {Scopus}, note = {Cited by: 1; All Open Access, Hybrid Gold Open Access} }
- Vlacic, L., Huang, H., Dotoli, M., Wang, Y., Ioannou, P. A., Fan, L., Wang, X., Carli, R., Lv, C., Li, L., Na, X., Han, Q. & Wang, F. (2024) Automation 5.0: The Key to Systems Intelligence and Industry 5.0. IN IEEE/CAA Journal of Automatica Sinica, 11.1723 – 1727. doi:10.1109/JAS.2024.124635
[BibTeX] [Abstract] [Download PDF]Automation has come a long way since the early days of mechanization, i.e., the process of working exclusively by hand or using animals to work with machinery. The rise of steam engines and water wheels represented the first generation of industry, which is now called Industry 1.0. Subsequently, Industry 2.0 witnessed the development of electric power and assembly lines. Later on, programmable logic controllers and Human Machine Interfaces (HMI) were the new productivity tools in Industry 3.0, which enabled precise and consistent production. In recent years, Industry 4.0 absorbed the latest technologies of Internet of Things (IoT), Artificial Intelligence (AI), and big data, making production processes integrated, interconnected, and smart. Nowadays, Industry 5.0 has been proposed, which emphasizes human-centric automation. Specifically, the new concept of automation in Industry 5.0, named Automation 5.0, is no longer about how to create machinery to replace humans. Instead, it aims to reach organic interactions and cooperation between humans and machines, meeting the goal of ‘6S’-Safety, Security, Sustainability, Sensitivity, Service, and Smartness [1]-[4]-and the overall objective of deploying automation for the better, human-friendly, and smarter industry. © 2014 Chinese Association of Automation.
@ARTICLE{Vlacic20241723, author = {Vlacic, Ljubo and Huang, Hailong and Dotoli, Mariagrazia and Wang, Yutong and Ioannou, Petros A. and Fan, Lili and Wang, Xingxia and Carli, Raffaele and Lv, Chen and Li, Lingxi and Na, Xiaoxiang and Han, Qing-Long and Wang, Fei-Yue}, title = {Automation 5.0: The Key to Systems Intelligence and Industry 5.0}, year = {2024}, journal = {IEEE/CAA Journal of Automatica Sinica}, volume = {11}, number = {8}, pages = {1723 – 1727}, doi = {10.1109/JAS.2024.124635}, url = {https://www.scopus.com/inward/record.uri?eid=2-s2.0-85199527866&doi=10.1109%2fJAS.2024.124635&partnerID=40&md5=bdd9d87d98cc8da08156c45367eb9eb2}, abstract = {Automation has come a long way since the early days of mechanization, i.e., the process of working exclusively by hand or using animals to work with machinery. The rise of steam engines and water wheels represented the first generation of industry, which is now called Industry 1.0. Subsequently, Industry 2.0 witnessed the development of electric power and assembly lines. Later on, programmable logic controllers and Human Machine Interfaces (HMI) were the new productivity tools in Industry 3.0, which enabled precise and consistent production. In recent years, Industry 4.0 absorbed the latest technologies of Internet of Things (IoT), Artificial Intelligence (AI), and big data, making production processes integrated, interconnected, and smart. Nowadays, Industry 5.0 has been proposed, which emphasizes human-centric automation. Specifically, the new concept of automation in Industry 5.0, named Automation 5.0, is no longer about how to create machinery to replace humans. Instead, it aims to reach organic interactions and cooperation between humans and machines, meeting the goal of '6S'-Safety, Security, Sustainability, Sensitivity, Service, and Smartness [1]-[4]-and the overall objective of deploying automation for the better, human-friendly, and smarter industry. © 2014 Chinese Association of Automation.}, keywords = {Accident prevention; Electric lines; Industry 4.0; Internet of things; Programmable logic controllers; Assembly line; Electric power lines; Human Machine Interface; Human-centric; Latest technology; Mechanisation; Production process; Productivity tools; System intelligence; Water wheel; Automation}, type = {Article}, publication_stage = {Final}, source = {Scopus}, note = {Cited by: 2} }
- Mignoni, N., Martinez-Piazuelo, J., Carli, R., Ocampo-Martinez, C., Quijano, N. & Dotoli, M. (2024) A Game-Theoretical Control Framework for Transactive Energy Trading in Energy Communities IN 2024 European Control Conference, ECC 2024., 786 – 791. doi:10.23919/ECC64448.2024.10591157
[BibTeX] [Abstract] [Download PDF]Under the umbrella of non-cooperative game theory, we formulate a transactive energy framework to model and control energy communities comprised of heterogeneous agents including (yet not limited to) prosumers, energy storage systems, and energy retailers. The underlying control task is defined as a generalized Nash equilibrium problem (GNEP), which must be solved in a distributed fashion. To solve the GNEP, we formulate a Gauss-Seidel-type alternating direction method of multipliers algorithm, which is guaranteed to converge under strongly monotone pseudo-gradient mappings. As such, we provide sufficient conditions on the private cost and energy pricing functions of the community members, so that the strong monotonicity of the overall pseudo-gradient is ensured. Finally, the proposed framework and the effectiveness of the solution method are illustrated through a numerical simulation. © 2024 EUCA.
@CONFERENCE{Mignoni2024786, author = {Mignoni, Nicola and Martinez-Piazuelo, Juan and Carli, Raffaele and Ocampo-Martinez, Carlos and Quijano, Nicanor and Dotoli, Mariagrazia}, title = {A Game-Theoretical Control Framework for Transactive Energy Trading in Energy Communities}, year = {2024}, journal = {2024 European Control Conference, ECC 2024}, pages = {786 – 791}, doi = {10.23919/ECC64448.2024.10591157}, url = {https://www.scopus.com/inward/record.uri?eid=2-s2.0-85200567031&doi=10.23919%2fECC64448.2024.10591157&partnerID=40&md5=940a89b8b7a2b8a90d241be8bd443e4b}, abstract = {Under the umbrella of non-cooperative game theory, we formulate a transactive energy framework to model and control energy communities comprised of heterogeneous agents including (yet not limited to) prosumers, energy storage systems, and energy retailers. The underlying control task is defined as a generalized Nash equilibrium problem (GNEP), which must be solved in a distributed fashion. To solve the GNEP, we formulate a Gauss-Seidel-type alternating direction method of multipliers algorithm, which is guaranteed to converge under strongly monotone pseudo-gradient mappings. As such, we provide sufficient conditions on the private cost and energy pricing functions of the community members, so that the strong monotonicity of the overall pseudo-gradient is ensured. Finally, the proposed framework and the effectiveness of the solution method are illustrated through a numerical simulation. © 2024 EUCA.}, keywords = {Game theory; Power markets; Control energy; Control framework; Energy; Energy trading; Generalized Nash equilibrium problems; Heterogeneous agents; Modelling and controls; Non-cooperative game theory; Pseudo gradients; Storage energy; Numerical methods}, type = {Conference paper}, publication_stage = {Final}, source = {Scopus}, note = {Cited by: 0} }
- Zhukovskii, K., Ovsiannikova, P., Jhunjhunwala, P., Scarabaggio, P., Carli, R., Dotoli, M. & Vyatkin, V. (2024) Energy Consumption Optimisation for Horticultural Facilities IN IEEE International Conference on Emerging Technologies and Factory Automation, ETFA.. doi:10.1109/ETFA61755.2024.10710757
[BibTeX] [Abstract] [Download PDF]This paper proposes a framework designed to optimise energy consumption in vertical farming. It aims to maximise cost efficiency by balancing between minimising system operations during the electricity price peaks and the ability to trade capacity on the FCR market while also fulfilling constraints on the internal growing process. We consider that the vertical farming system has distributed control with a series of actuators controlled by various spatially distributed PLCs that we refer to as agents, to underline their independence. The paper conducts two experiments for a lO-agent system with a Pareto controller and a lOO-agent system with a Lagrangian approach and shows the balance between more cost-efficient momentary energy consumption control. © 2024 IEEE.
@CONFERENCE{Zhukovskii2024, author = {Zhukovskii, Kirill and Ovsiannikova, Polina and Jhunjhunwala, Pranay and Scarabaggio, Paolo and Carli, Raffaele and Dotoli, Mariagrazia and Vyatkin, Valeriy}, title = {Energy Consumption Optimisation for Horticultural Facilities}, year = {2024}, journal = {IEEE International Conference on Emerging Technologies and Factory Automation, ETFA}, doi = {10.1109/ETFA61755.2024.10710757}, url = {https://www.scopus.com/inward/record.uri?eid=2-s2.0-85207826602&doi=10.1109%2fETFA61755.2024.10710757&partnerID=40&md5=301035e618ab242689424422b21164c4}, abstract = {This paper proposes a framework designed to optimise energy consumption in vertical farming. It aims to maximise cost efficiency by balancing between minimising system operations during the electricity price peaks and the ability to trade capacity on the FCR market while also fulfilling constraints on the internal growing process. We consider that the vertical farming system has distributed control with a series of actuators controlled by various spatially distributed PLCs that we refer to as agents, to underline their independence. The paper conducts two experiments for a lO-agent system with a Pareto controller and a lOO-agent system with a Lagrangian approach and shows the balance between more cost-efficient momentary energy consumption control. © 2024 IEEE.}, author_keywords = {energy consumption optimisation; multi-agent systems; vertical farming}, keywords = {Agent systems; Cost-efficiency; Electricity prices; Energy consumption optimization; Energy-consumption; Farming system; Growing process; Multiagent systems (MASs); Systems operation; Vertical farming}, type = {Conference paper}, publication_stage = {Final}, source = {Scopus}, note = {Cited by: 0} }
2023
- Mignoni, N., Carli, R. & Dotoli, M. (2023) Layout Optimization for Photovoltaic Panels in Solar Power Plants via a MINLP Approach. IN IEEE Transactions on Automation Science and Engineering, .1–14. doi:10.1109/TASE.2023.3322786
[BibTeX] [Abstract] [Download PDF]Photovoltaic (PV) technology is one of the most popular means of renewable generation, whose applications range from commercial and residential buildings to industrial facilities and grid infrastructures. The problem of determining a suitable layout for the PV arrays, on a given deployment region, is generally non-trivial and has a crucial importance in the planning phase of solar plants design and development. In this paper, we provide a mixed integer non-linear programming formulation of the PV arrays’ layout problem. First, we define the astronomical and geometrical models, considering crucial factors such as self-shadowing and irradiance variability, depending on the geographical position of the solar plant and yearly time window. Subsequently, we formalize the mathematical optimization problem, whose constraints’ set is characterized by non-convexities. In order to propose a computationally tractable approach, we provide a tight parametrized convex relaxation. The resulting optimization resolution procedure is tested numerically, using realistic data, and benchmarked against the traditional global resolution approach, showing that the proposed methodology yields near-optimal solutions in lower computational time.
Note to Practitioners —The paper is motivated by the need for efficient algorithmic procedures which can yield near-optimal solutions to the PV arrays layout problem. Due to the strong non-convexity of even simple instances, the existing methods heavily rely on global or stochastic solvers, which are computationally demanding, both in terms of resources and run-time. Our approach acts as a baseline, from which practitioners can derive more elaborate instances, by suitably modifying both the objective function and/or the constraints. In fact, we focus on the minimum set of necessary geometrical (e.g., arrays position model), astronomical (e.g., irradiance variation), and operational (e.g., power requirements) constraints which make the overall problem hard. The Appendices provide a guideline for suitably choosing the optimization parameters. All data and simulation code are available on a public repository at: https://github.com/nicomignoni/pvlayout.git. Authors@ARTICLE{Mignoni20231, author = {Mignoni, Nicola and Carli, Raffaele and Dotoli, Mariagrazia}, title = {Layout Optimization for Photovoltaic Panels in Solar Power Plants via a MINLP Approach}, year = {2023}, journal = {IEEE Transactions on Automation Science and Engineering}, pages = {1–14}, doi = {10.1109/TASE.2023.3322786}, url = {https://www.scopus.com/inward/record.uri?eid=2-s2.0-85181574361&doi=10.1109%2fTASE.2023.3322786&partnerID=40&md5=81a2752bb7efb922f17af8b437e4693b}, abstract = {Photovoltaic (PV) technology is one of the most popular means of renewable generation, whose applications range from commercial and residential buildings to industrial facilities and grid infrastructures. The problem of determining a suitable layout for the PV arrays, on a given deployment region, is generally non-trivial and has a crucial importance in the planning phase of solar plants design and development. In this paper, we provide a mixed integer non-linear programming formulation of the PV arrays’ layout problem. First, we define the astronomical and geometrical models, considering crucial factors such as self-shadowing and irradiance variability, depending on the geographical position of the solar plant and yearly time window. Subsequently, we formalize the mathematical optimization problem, whose constraints’ set is characterized by non-convexities. In order to propose a computationally tractable approach, we provide a tight parametrized convex relaxation. The resulting optimization resolution procedure is tested numerically, using realistic data, and benchmarked against the traditional global resolution approach, showing that the proposed methodology yields near-optimal solutions in lower computational time.
Note to Practitioners —The paper is motivated by the need for efficient algorithmic procedures which can yield near-optimal solutions to the PV arrays layout problem. Due to the strong non-convexity of even simple instances, the existing methods heavily rely on global or stochastic solvers, which are computationally demanding, both in terms of resources and run-time. Our approach acts as a baseline, from which practitioners can derive more elaborate instances, by suitably modifying both the objective function and/or the constraints. In fact, we focus on the minimum set of necessary geometrical (e.g., arrays position model), astronomical (e.g., irradiance variation), and operational (e.g., power requirements) constraints which make the overall problem hard. The Appendices provide a guideline for suitably choosing the optimization parameters. All data and simulation code are available on a public repository at: https://github.com/nicomignoni/pvlayout.git. Authors}, author_keywords = {Azimuth; convexification; Layout; Mathematical models; mixed integer non-linear programming; non-convex optimization; Observers; Optimization; parametrization; Photovoltaic; Photovoltaic systems; solar array layout; solar power plants; Sun}, keywords = {Convex optimization; Integer programming; Nonlinear programming; Optimal systems; Relaxation processes; Solar energy; Solar panels; Solar power generation; Stochastic systems; Array layout; Azimuth; Convexification; Layout; Mixed-integer nonlinear programming; Nonconvex optimization; Observer; Optimisations; Parametrizations; Photovoltaic systems; Photovoltaics; Solar array layout; Solar arrays; Solar power plants}, type = {Article}, publication_stage = {Article in press}, source = {Scopus}, note = {Cited by: 0; All Open Access, Green Open Access, Hybrid Gold Open Access} } - Mignoni, N., Carli, R. & Dotoli, M. (2023) A Noncooperative Stochastic Rolling Horizon Control Framework for V1G and V2B Scheduling in Energy Communities IN 2023 European Control Conference, ECC 2023.. doi:10.23919/ECC57647.2023.10178202
[BibTeX] [Abstract] [Download PDF]In this paper, we propose a novel control strategy for the optimal scheduling of an energy community constituted by prosumers and equipped with unidirectional vehicle-to-grid (V1G) and vehicle-to-building (V2B) capabilities. In particular, V2B services are provided by long-term parked electric vehicles (EVs) thus acting as temporary storage systems by prosumers, which in turn offer the V1G service to EVs provisionally plugged into charging stations. To tackle the stochastic nature of the framework, we assume that EVs communicate their parking and recharging time distribution to prosumers, allowing them to improve their energy allocation. Prosumers and EVs interact in a rolling horizon control framework with the aim of achieving an agreement on their operating strategies. The resulting control problem is formulated as a generalized Nash equilibrium problem, addressed through the variational inequality theory, and solved in a distributed fashion leveraging on the accelerated distributed augmented Lagrangian method (ADALM). The convergence and effectiveness of the proposed approach are validated through numerical simulations under realistic scenarios. © 2023 EUCA.
@CONFERENCE{Mignoni2023, author = {Mignoni, Nicola and Carli, Raffaele and Dotoli, Mariagrazia}, title = {A Noncooperative Stochastic Rolling Horizon Control Framework for V1G and V2B Scheduling in Energy Communities}, year = {2023}, journal = {2023 European Control Conference, ECC 2023}, doi = {10.23919/ECC57647.2023.10178202}, url = {https://www.scopus.com/inward/record.uri?eid=2-s2.0-85166479889&doi=10.23919%2fECC57647.2023.10178202&partnerID=40&md5=e34ca4551ecbe785858f9937e0c0a38c}, abstract = {In this paper, we propose a novel control strategy for the optimal scheduling of an energy community constituted by prosumers and equipped with unidirectional vehicle-to-grid (V1G) and vehicle-to-building (V2B) capabilities. In particular, V2B services are provided by long-term parked electric vehicles (EVs) thus acting as temporary storage systems by prosumers, which in turn offer the V1G service to EVs provisionally plugged into charging stations. To tackle the stochastic nature of the framework, we assume that EVs communicate their parking and recharging time distribution to prosumers, allowing them to improve their energy allocation. Prosumers and EVs interact in a rolling horizon control framework with the aim of achieving an agreement on their operating strategies. The resulting control problem is formulated as a generalized Nash equilibrium problem, addressed through the variational inequality theory, and solved in a distributed fashion leveraging on the accelerated distributed augmented Lagrangian method (ADALM). The convergence and effectiveness of the proposed approach are validated through numerical simulations under realistic scenarios. © 2023 EUCA.}, keywords = {Lagrange multipliers; Stochastic systems; Variational techniques; Vehicle-to-grid; Vehicles; Control framework; Control strategies; Energy; Horizon control; Optimal scheduling; Rolling horizon; Stochastics; Temporary storage; Unidirectional vehicles; Vehicle to grids; Constrained optimization}, type = {Conference paper}, publication_stage = {Final}, source = {Scopus}, note = {Cited by: 0} }
- Mignoni, N., Carli, R. & Dotoli, M. (2023) Distributed Noncooperative MPC for Energy Scheduling of Charging and Trading Electric Vehicles in Energy Communities. IN IEEE Transactions on Control Systems Technology, 31.2159 – 2172. doi:10.1109/TCST.2023.3291549
[BibTeX] [Abstract] [Download PDF]In this article, we propose a novel control strategy for the optimal scheduling of an energy community (EC) constituted by prosumers and equipped with unidirectional vehicle-to-grid (V1G) and vehicle-to-building (V2B) capabilities. In particular, V2B services are provided by long-term parked electric vehicles (EVs), used as temporary storage systems by prosumers, who in turn offer the V1G service to EVs provisionally plugged into charging stations. To tackle the stochastic nature of the framework, we assume that EVs communicate their parking and recharging time distribution to prosumers, allowing them to improve the energy allocation process. Acting as selfish agents, prosumers and EVs interact in a rolling horizon control framework with the aim of achieving an agreement on their operating strategies. The resulting control problem is formulated as a generalized Nash equilibrium (NE) problem, addressed through the variational inequality (VI) theory, and solved in a distributed fashion leveraging on the accelerated distributed augmented Lagrangian method (ADALM), showing sufficient conditions for guaranteeing convergence. The proposed model predictive control (MPC) approach is validated through numerical simulations under realistic scenarios. © 1993-2012 IEEE.
@ARTICLE{Mignoni20232159, author = {Mignoni, Nicola and Carli, Raffaele and Dotoli, Mariagrazia}, title = {Distributed Noncooperative MPC for Energy Scheduling of Charging and Trading Electric Vehicles in Energy Communities}, year = {2023}, journal = {IEEE Transactions on Control Systems Technology}, volume = {31}, number = {5}, pages = {2159 – 2172}, doi = {10.1109/TCST.2023.3291549}, url = {https://www.scopus.com/inward/record.uri?eid=2-s2.0-85165268827&doi=10.1109%2fTCST.2023.3291549&partnerID=40&md5=a1747e82483205009749a103f96f0f12}, abstract = {In this article, we propose a novel control strategy for the optimal scheduling of an energy community (EC) constituted by prosumers and equipped with unidirectional vehicle-to-grid (V1G) and vehicle-to-building (V2B) capabilities. In particular, V2B services are provided by long-term parked electric vehicles (EVs), used as temporary storage systems by prosumers, who in turn offer the V1G service to EVs provisionally plugged into charging stations. To tackle the stochastic nature of the framework, we assume that EVs communicate their parking and recharging time distribution to prosumers, allowing them to improve the energy allocation process. Acting as selfish agents, prosumers and EVs interact in a rolling horizon control framework with the aim of achieving an agreement on their operating strategies. The resulting control problem is formulated as a generalized Nash equilibrium (NE) problem, addressed through the variational inequality (VI) theory, and solved in a distributed fashion leveraging on the accelerated distributed augmented Lagrangian method (ADALM), showing sufficient conditions for guaranteeing convergence. The proposed model predictive control (MPC) approach is validated through numerical simulations under realistic scenarios. © 1993-2012 IEEE.}, author_keywords = {Distributed optimization; electric vehicles (EVs); energy communities (ECs); game theory; model predictive control (MPC); unilateral vehicle-to-grid (V1G); vehicle-to-building (V2B)}, keywords = {Charging (batteries); Constrained optimization; Electric vehicles; Lagrange multipliers; Model predictive control; Predictive control systems; Random processes; Secondary batteries; Stochastic control systems; Stochastic models; Stochastic systems; Variational techniques; Vehicle-to-grid; Battery; Distributed optimization; Electric vehicle; Energy; Energy community; Game; Microgrid; Model predictive control; Model-predictive control; Symmetric matrices; Uncertainty; Unilateral vehicle-to-grid (V1G); Vehicle to grids; Vehicle-to-building (V2B); Game theory}, type = {Article}, publication_stage = {Final}, source = {Scopus}, note = {Cited by: 13; All Open Access, Green Open Access, Hybrid Gold Open Access} }
- Mignoni, N., Scarabaggio, P., Carli, R. & Dotoli, M. (2023) Control frameworks for transactive energy storage services in energy communities. IN Control Engineering Practice, 130.. doi:10.1016/j.conengprac.2022.105364
[BibTeX] [Abstract] [Download PDF]Recently, the decreasing cost of storage technologies and the emergence of economy-driven mechanisms for energy exchange are contributing to the spread of energy communities. In this context, this paper aims at defining innovative transactive control frameworks for energy communities equipped with independent service-oriented energy storage systems. The addressed control problem consists in optimally scheduling the energy activities of a group of prosumers, characterized by their own demand and renewable generation, and a group of energy storage service providers, able to store the prosumers’ energy surplus and, subsequently, release it upon a fee payment. We propose two novel resolution algorithms based on a game theoretical control formulation, a coordinated and an uncoordinated one, which can be alternatively used depending on the underlying communication architecture of the grid. The two proposed approaches are validated through numerical simulations on realistic scenarios. Results show that the use of a particular framework does not alter fairness, at least at the community level, i.e., no participant in the groups of prosumers or providers can strongly benefit from changing its strategy while compromising others’ welfare. Lastly, the approaches are compared with a centralized control method showing better computational results. © 2022 Elsevier Ltd
@ARTICLE{Mignoni2023, author = {Mignoni, Nicola and Scarabaggio, Paolo and Carli, Raffaele and Dotoli, Mariagrazia}, title = {Control frameworks for transactive energy storage services in energy communities}, year = {2023}, journal = {Control Engineering Practice}, volume = {130}, doi = {10.1016/j.conengprac.2022.105364}, url = {https://www.scopus.com/inward/record.uri?eid=2-s2.0-85140312671&doi=10.1016%2fj.conengprac.2022.105364&partnerID=40&md5=c2f86f25172d9c67e24d1f09c9b54689}, abstract = {Recently, the decreasing cost of storage technologies and the emergence of economy-driven mechanisms for energy exchange are contributing to the spread of energy communities. In this context, this paper aims at defining innovative transactive control frameworks for energy communities equipped with independent service-oriented energy storage systems. The addressed control problem consists in optimally scheduling the energy activities of a group of prosumers, characterized by their own demand and renewable generation, and a group of energy storage service providers, able to store the prosumers’ energy surplus and, subsequently, release it upon a fee payment. We propose two novel resolution algorithms based on a game theoretical control formulation, a coordinated and an uncoordinated one, which can be alternatively used depending on the underlying communication architecture of the grid. The two proposed approaches are validated through numerical simulations on realistic scenarios. Results show that the use of a particular framework does not alter fairness, at least at the community level, i.e., no participant in the groups of prosumers or providers can strongly benefit from changing its strategy while compromising others’ welfare. Lastly, the approaches are compared with a centralized control method showing better computational results. © 2022 Elsevier Ltd}, author_keywords = {Distributed control; Energy communities; Energy storage systems; Game theory; Smart grids; Transactive control; Transactive energy management}, keywords = {Computation theory; Distributed parameter control systems; Electric power transmission networks; Energy storage; Smart power grids; Control framework; Distributed-control; Energy; Energy community; Energy storage system; Smart grid; Storage services; Storage systems; Transactive controls; Transactive energy management; Game theory}, type = {Article}, publication_stage = {Final}, source = {Scopus}, note = {Cited by: 30; All Open Access, Green Open Access} }
- Proia, S., Cavone, G., Carli, R. & Dotoli, M. (2023) Optimal Control of Drones for a Train-Drone Railway Diagnostic System IN IEEE International Conference on Automation Science and Engineering.. doi:10.1109/CASE56687.2023.10260390
[BibTeX] [Abstract] [Download PDF]The inspection of railway systems with traditional wayside detectors allows mainly the detection of wheels and axle bearings defects and can be time-demanding, unsafe, and heavily dependent on humans. To overcome these issues and then optimize and automate the rail and track diagnosis process, drones can be an excellent solution thanks to their onboard state-of-the-art cameras and sensors. Thus, with the aim of rapidly collecting highly accurate data, an innovative hybrid movable railway diagnostic architecture, consisting of a diagnostic train and a fleet of drones, is defined in this work. From the control point of view, the main interest is in optimally managing the crucial phase of drones returning to and landing on the moving train when the railway inspection mission is completed. To control the fleet of drones, a combination of consensus algorithm in the leader-following mode and linear quadratic regulator (LQR) is implemented for the flight formation phase and the landing phase on the moving base platform (i.e., the diagnostic train), respectively. The landing phase is performed both as vertical or oblique descent through a go to goal and as oblique descent along a predefined path. The obtained results of the railway diagnostic architecture simulations are presented and discussed in detail. In particular, they show that the vertical and oblique descent performed as go to goal are certainly faster than the oblique descent along a predefined path. © 2023 IEEE.
@CONFERENCE{Proia2023, author = {Proia, Silvia and Cavone, Graziana and Carli, Raffaele and Dotoli, Mariagrazia}, title = {Optimal Control of Drones for a Train-Drone Railway Diagnostic System}, year = {2023}, journal = {IEEE International Conference on Automation Science and Engineering}, volume = {2023-August}, doi = {10.1109/CASE56687.2023.10260390}, url = {https://www.scopus.com/inward/record.uri?eid=2-s2.0-85174392294&doi=10.1109%2fCASE56687.2023.10260390&partnerID=40&md5=e22983c3ba56e013e991d3313a84b33f}, abstract = {The inspection of railway systems with traditional wayside detectors allows mainly the detection of wheels and axle bearings defects and can be time-demanding, unsafe, and heavily dependent on humans. To overcome these issues and then optimize and automate the rail and track diagnosis process, drones can be an excellent solution thanks to their onboard state-of-the-art cameras and sensors. Thus, with the aim of rapidly collecting highly accurate data, an innovative hybrid movable railway diagnostic architecture, consisting of a diagnostic train and a fleet of drones, is defined in this work. From the control point of view, the main interest is in optimally managing the crucial phase of drones returning to and landing on the moving train when the railway inspection mission is completed. To control the fleet of drones, a combination of consensus algorithm in the leader-following mode and linear quadratic regulator (LQR) is implemented for the flight formation phase and the landing phase on the moving base platform (i.e., the diagnostic train), respectively. The landing phase is performed both as vertical or oblique descent through a go to goal and as oblique descent along a predefined path. The obtained results of the railway diagnostic architecture simulations are presented and discussed in detail. In particular, they show that the vertical and oblique descent performed as go to goal are certainly faster than the oblique descent along a predefined path. © 2023 IEEE.}, author_keywords = {diagnostics; drone; flight formation; landing; leader following consensus; linear quadratic regulator; quadrotor; Railways}, keywords = {Landing; Railroads; Rails; Diagnostic; Flight formation; Landing phase; Leader following; Leader following consensus; Linear quadratic; Linear quadratic regulator; Quad rotors; Quadratic regulators; Railway; Drones}, type = {Conference paper}, publication_stage = {Final}, source = {Scopus}, note = {Cited by: 1} }
- Askari, B., Bozza, A., Cavone, G., Carli, R. & Dotoli, M. (2023) An adaptive constrained clustering approach for real-time fault detection of industrial systems. IN European Journal of Control, 74.. doi:10.1016/j.ejcon.2023.100858
[BibTeX] [Abstract] [Download PDF]Thanks to the pervasive deployment of sensors in Industry 4.0, data-driven methods are recently playing an important role in the fault diagnosis and prognosis of industrial systems. In this paper, a novel Adaptive Constrained Clustering algorithm is defined to support real-time fault detection of an industrial machine, by clustering the incoming monitoring data into two clusters over time, representing the nominal and non-nominal work conditions, respectively. To this aim, the proposed algorithm relies on a two-stage procedure: micro-clustering and constrained macro-clustering. The former stage is responsible for grouping the batches of work-cycle data into micro-clusters, while the data stream continuously arrives from the data acquisition system. Then, after condensing the micro-clusters into vectors of cluster features, and leveraging on additional knowledge on the nominal and non-nominal working conditions (i.e., constraints on some samples), the second stage aims at offline grouping the micro-clusters features into macro-clusters. Experimental results on a real-world industrial case study show that the proposed real time framework achieves the same results of offline baseline methods (e.g., Constrained K-means) with a higher responsiveness and processing speed; in comparison to stream baseline methods (e.g., Stream K-means), the proposed approach obtains more accurate and easily interpretable results. © 2023 European Control Association
@ARTICLE{Askari2023, author = {Askari, Bahman and Bozza, Augusto and Cavone, Graziana and Carli, Raffaele and Dotoli, Mariagrazia}, title = {An adaptive constrained clustering approach for real-time fault detection of industrial systems}, year = {2023}, journal = {European Journal of Control}, volume = {74}, doi = {10.1016/j.ejcon.2023.100858}, url = {https://www.scopus.com/inward/record.uri?eid=2-s2.0-85162877723&doi=10.1016%2fj.ejcon.2023.100858&partnerID=40&md5=e062207fcb506e79c37bedf7a930b430}, abstract = {Thanks to the pervasive deployment of sensors in Industry 4.0, data-driven methods are recently playing an important role in the fault diagnosis and prognosis of industrial systems. In this paper, a novel Adaptive Constrained Clustering algorithm is defined to support real-time fault detection of an industrial machine, by clustering the incoming monitoring data into two clusters over time, representing the nominal and non-nominal work conditions, respectively. To this aim, the proposed algorithm relies on a two-stage procedure: micro-clustering and constrained macro-clustering. The former stage is responsible for grouping the batches of work-cycle data into micro-clusters, while the data stream continuously arrives from the data acquisition system. Then, after condensing the micro-clusters into vectors of cluster features, and leveraging on additional knowledge on the nominal and non-nominal working conditions (i.e., constraints on some samples), the second stage aims at offline grouping the micro-clusters features into macro-clusters. Experimental results on a real-world industrial case study show that the proposed real time framework achieves the same results of offline baseline methods (e.g., Constrained K-means) with a higher responsiveness and processing speed; in comparison to stream baseline methods (e.g., Stream K-means), the proposed approach obtains more accurate and easily interpretable results. © 2023 European Control Association}, author_keywords = {Constrained clustering; Fault detection; Industrial systems; Machine learning; Stream clustering; Unsupervised learning}, keywords = {Data acquisition; K-means clustering; Knowledge management; Real time systems; Unsupervised learning; Cluster feature; Clusterings; Constrained clustering; Faults detection; Industrial systems; Machine-learning; Micro-clusters; Offline; Real time fault detection; Stream clustering; Fault detection}, type = {Article}, publication_stage = {Final}, source = {Scopus}, note = {Cited by: 2} }
- Dammacco, L., Carli, R., Gattullo, M., Lazazzera, V., Fiorentino, M. & Dotoli, M. (2023) Virtual Golden Zone for Enhancing the Ergonomics of Complex Production Lines. IN Lecture Notes in Mechanical Engineering, .1436 – 1447. doi:10.1007/978-3-031-15928-2_125
[BibTeX] [Abstract] [Download PDF]For the sake of being competitive in an ever-changing market, industrial companies need a redefinition of traditional design and integration of parts, equipment, and services such a redefinition allows effectively addressing the interaction between machines and operators, particularly in the area of complex production lines. In this context, enhancing ergonomics is crucial to reduce fatigue and stress of workers and increase work-place efficiency and comfort. Moreover, identifying ergonomic flaws in three-dimensional human-machine design problems (e.g., body posture, reach, visibility) at an early stage of the engineering process allows to prevent these issues at a low cost. Virtual reality (VR) is emerging as a powerful tool to improve the ergonomic assessment in the design of complex production lines. However, VR is not yet a well-consolidated practice for industrial companies, and the state-of-the-art applications are limited to simplified, isolated, and customized experiments. This work proposes the use of a virtual golden zone (VGZ) as a standard and efficient VR method for the ergonomic analysis and optimization of operator activities in manual manufacturing stations. The resulting effectiveness and benefits are highlighted through the application of the approach to a real industrial case study. Finally, the outcomes of a usability questionnaire, compiled by the professionals involved in the VR reviews, are presented to evaluate the usability of the VGZ methodology in the design process of complex production lines. © 2023, The Author(s), under exclusive license to Springer Nature Switzerland AG.
@ARTICLE{Dammacco20231436, author = {Dammacco, Lucilla and Carli, Raffaele and Gattullo, Michele and Lazazzera, Vito and Fiorentino, Michele and Dotoli, Mariagrazia}, title = {Virtual Golden Zone for Enhancing the Ergonomics of Complex Production Lines}, year = {2023}, journal = {Lecture Notes in Mechanical Engineering}, pages = {1436 – 1447}, doi = {10.1007/978-3-031-15928-2_125}, url = {https://www.scopus.com/inward/record.uri?eid=2-s2.0-85140467887&doi=10.1007%2f978-3-031-15928-2_125&partnerID=40&md5=b6846c4969dd54de6bdc98c21de2c979}, abstract = {For the sake of being competitive in an ever-changing market, industrial companies need a redefinition of traditional design and integration of parts, equipment, and services such a redefinition allows effectively addressing the interaction between machines and operators, particularly in the area of complex production lines. In this context, enhancing ergonomics is crucial to reduce fatigue and stress of workers and increase work-place efficiency and comfort. Moreover, identifying ergonomic flaws in three-dimensional human-machine design problems (e.g., body posture, reach, visibility) at an early stage of the engineering process allows to prevent these issues at a low cost. Virtual reality (VR) is emerging as a powerful tool to improve the ergonomic assessment in the design of complex production lines. However, VR is not yet a well-consolidated practice for industrial companies, and the state-of-the-art applications are limited to simplified, isolated, and customized experiments. This work proposes the use of a virtual golden zone (VGZ) as a standard and efficient VR method for the ergonomic analysis and optimization of operator activities in manual manufacturing stations. The resulting effectiveness and benefits are highlighted through the application of the approach to a real industrial case study. Finally, the outcomes of a usability questionnaire, compiled by the professionals involved in the VR reviews, are presented to evaluate the usability of the VGZ methodology in the design process of complex production lines. © 2023, The Author(s), under exclusive license to Springer Nature Switzerland AG.}, author_keywords = {Complex production lines; Ergonomics; Human computer interaction; Virtual reality}, keywords = {Cost engineering; Ergonomics; Human computer interaction; Machine design; Body postures; Complex production; Complex production line; Design and integrations; Design problems; Human-machine; Industrial companies; Production line; Work place; Workers'; Virtual reality}, type = {Conference paper}, publication_stage = {Final}, source = {Scopus}, note = {Cited by: 0} }
- Askari, B., Cavone, G., Carli, R., Grall, A. & Dotoli, M. (2023) A Semi-Supervised Learning Approach for Fault Detection and Diagnosis in Complex Mechanical Systems IN IEEE International Conference on Automation Science and Engineering.. doi:10.1109/CASE56687.2023.10260469
[BibTeX] [Abstract] [Download PDF]The integration of artificial intelligence in mechanical fault detection and diagnosis (FDD) helps to increase reliability, reduce costs, and improve the overall performance of mechanical systems in Industry 4.0 applications. Most interesting industrial applications nowadays come from dynamic environments where data are generated continuously over time and where the labeled data are scarce and expensive. Therefore, semi-supervised learning (SSL) can be particularly useful in FDD because faults may be rare or difficult to identify, and may not be fully represented in the labeled data. By using a combination of labeled and unlabeled data, SSL can help to identify these rare or difficult-to-detect faults, leading to more effective FDD. In this paper, graph-based SSL relying on label propagation is combined with conventional classification algorithms to detect potential failures in complex mechanical systems. Experimental results on realistic pneumatic and hydraulic systems from the related literature show that the proposed method can effectively enlarge the labeled datasets and interestingly identify different types of non-nominal conditions with higher accuracy compared to baseline methodologies. © 2023 IEEE.
@CONFERENCE{Askari2023, author = {Askari, Bahman and Cavone, Graziana and Carli, Raffaele and Grall, Antoine and Dotoli, Mariagrazia}, title = {A Semi-Supervised Learning Approach for Fault Detection and Diagnosis in Complex Mechanical Systems}, year = {2023}, journal = {IEEE International Conference on Automation Science and Engineering}, volume = {2023-August}, doi = {10.1109/CASE56687.2023.10260469}, url = {https://www.scopus.com/inward/record.uri?eid=2-s2.0-85174423884&doi=10.1109%2fCASE56687.2023.10260469&partnerID=40&md5=ec89c19b64a796dcd68e955a87b2769a}, abstract = {The integration of artificial intelligence in mechanical fault detection and diagnosis (FDD) helps to increase reliability, reduce costs, and improve the overall performance of mechanical systems in Industry 4.0 applications. Most interesting industrial applications nowadays come from dynamic environments where data are generated continuously over time and where the labeled data are scarce and expensive. Therefore, semi-supervised learning (SSL) can be particularly useful in FDD because faults may be rare or difficult to identify, and may not be fully represented in the labeled data. By using a combination of labeled and unlabeled data, SSL can help to identify these rare or difficult-to-detect faults, leading to more effective FDD. In this paper, graph-based SSL relying on label propagation is combined with conventional classification algorithms to detect potential failures in complex mechanical systems. Experimental results on realistic pneumatic and hydraulic systems from the related literature show that the proposed method can effectively enlarge the labeled datasets and interestingly identify different types of non-nominal conditions with higher accuracy compared to baseline methodologies. © 2023 IEEE.}, keywords = {Fault detection; Hydraulic equipment; Learning systems; Complex mechanical system; Dynamic environments; Fault detection and diagnosis; Labeled data; Mechanical faults; Mechanical systems; Performance; Reduce costs; Semi-supervised learning; Supervised learning approaches; Graphic methods}, type = {Conference paper}, publication_stage = {Final}, source = {Scopus}, note = {Cited by: 0} }
- Mignoni, N., Carli, R. & Dotoli, M. (2023) An Optimization Tool for Displacing Photovoltaic Arrays in Polygonal Areas IN EUROCON 2023 – 20th International Conference on Smart Technologies, Proceedings., 573 – 578. doi:10.1109/EUROCON56442.2023.10198934
[BibTeX] [Abstract] [Download PDF]In this paper, we discuss the problem of optimally designing the layout of a given number of photovoltaic arrays on a flat polygonal surface, in order to maximize a suitable objective function, e.g., the total generated energy. This means finding their optimal position, azimuth and tilt. The considered problem becomes non-trivial when considering effects such as irradiance variability and self-shadowing. We first provide a description of the system model and the associated optimization problem, showing how the resulting formulation presents non-convexities. Then, we provide a tight parametrized convex relaxation, which is computationally tractable and for which optimality conditions hold. We provide numerical simulations using realistic data, showing how the proposed methodology yields near-optimal solutions in lower computational time with respect to the traditional global resolution approach. © 2023 IEEE.
@CONFERENCE{Mignoni2023573, author = {Mignoni, Nicola and Carli, Raffaele and Dotoli, Mariagrazia}, title = {An Optimization Tool for Displacing Photovoltaic Arrays in Polygonal Areas}, year = {2023}, journal = {EUROCON 2023 - 20th International Conference on Smart Technologies, Proceedings}, pages = {573 – 578}, doi = {10.1109/EUROCON56442.2023.10198934}, url = {https://www.scopus.com/inward/record.uri?eid=2-s2.0-85168705720&doi=10.1109%2fEUROCON56442.2023.10198934&partnerID=40&md5=918aa0710a8540e5ce9ea625b0a385d4}, abstract = {In this paper, we discuss the problem of optimally designing the layout of a given number of photovoltaic arrays on a flat polygonal surface, in order to maximize a suitable objective function, e.g., the total generated energy. This means finding their optimal position, azimuth and tilt. The considered problem becomes non-trivial when considering effects such as irradiance variability and self-shadowing. We first provide a description of the system model and the associated optimization problem, showing how the resulting formulation presents non-convexities. Then, we provide a tight parametrized convex relaxation, which is computationally tractable and for which optimality conditions hold. We provide numerical simulations using realistic data, showing how the proposed methodology yields near-optimal solutions in lower computational time with respect to the traditional global resolution approach. © 2023 IEEE.}, keywords = {Energy; Non-trivial; Nonconvexity; Objective functions; Optimal position; Optimization problems; Optimization tools; Photovoltaic arrays; Polygonal surface; System models; Relaxation processes}, type = {Conference paper}, publication_stage = {Final}, source = {Scopus}, note = {Cited by: 0} }
- Proia, S., Cavone, G., Scarabaggio, P., Carli, R. & Dotoli, M. (2023) Safety Compliant, Ergonomic and Time-Optimal Trajectory Planning for Collaborative Robotics. IN IEEE Transactions on Automation Science and Engineering, .1–12. doi:10.1109/TASE.2023.3331505
[BibTeX] [Abstract] [Download PDF]The demand for safe and ergonomic workplaces is rapidly growing in modern industrial scenarios, especially for companies that intensely rely on Human-Robot Collaboration (HRC). This work focuses on optimizing the trajectory of the end-effector of a cobot arm in a collaborative industrial environment, ensuring the maximization of the operator’s safety and ergonomics without sacrificing production efficiency requirements. Hence, a multi-objective optimization strategy for trajectory planning in a safe and ergonomic HRC is defined. This approach aims at finding the best trade-off between the total traversal time of the cobot’s end-effector trajectory and ergonomics for the human worker, while respecting in the kinematic constraint of the optimization problem the ISO safety requirements through the well-known Speed and Separation Monitoring (SSM) methodology. Guaranteeing an ergonomic HRC means reducing musculoskeletal disorders linked to risky and highly repetitive activities. The three main phases of the proposed technique are described as follows. First, a manikin designed using a dedicated software is employed to evaluate the Rapid Upper Limb Assessment (RULA) ergonomic index in the working area. Next, a second-order cone programming problem is defined to represent a time-optimal safety compliant trajectory planning problem. Finally, the trajectory that ensures the best compromise between these two opposing goals –minimizing the task’s traversal time and maintaining a high level of ergonomics for the human worker– is computed by defining and solving a multi-objective control problem. The method is tested on an experimental case study in reference to an assembly task and the obtained results are discussed, showing the effectiveness of the proposed approach.
Note to Practitioners —Health and safety in workplaces are business imperatives, since they ensure not only a safe collaboration between industrial machinery and human operators, but also an increased productivity and flexibility of the entire industrial process. Hence, investing in health is a real driver for business growth. The key enabling technologies of Industry 4.0, such as collaborative robotics, exoskeletons, virtual and augmented reality, require standardization and indispensable technical safety requirements that cannot ignore physical, sensory, and psychological peculiarities of the human worker and aspects like usability and acceptability of these technologies in performing their activities. Against this ongoing industrial challenge, the aim of this paper is to provide researchers and practitioners with an innovative HRC trajectory planning methodology focused on enhancing production efficiency while respecting the SSM ISO safety requirement and guaranteeing the ergonomic optimal position of the operator during an assembly task. Therefore, the proposed methodology can be a convenient solution to be deployed in industrial companies, since it can support human operators by drastically reducing work-related musculoskeletal disorders and augmenting their performance in the working environment. Authors@ARTICLE{Proia20231, author = {Proia, Silvia and Cavone, Graziana and Scarabaggio, Paolo and Carli, Raffaele and Dotoli, Mariagrazia}, title = {Safety Compliant, Ergonomic and Time-Optimal Trajectory Planning for Collaborative Robotics}, year = {2023}, journal = {IEEE Transactions on Automation Science and Engineering}, pages = {1–12}, doi = {10.1109/TASE.2023.3331505}, url = {https://www.scopus.com/inward/record.uri?eid=2-s2.0-85178048425&doi=10.1109%2fTASE.2023.3331505&partnerID=40&md5=7fe6504b770617ce6996f3bf55c5d7c1}, abstract = {The demand for safe and ergonomic workplaces is rapidly growing in modern industrial scenarios, especially for companies that intensely rely on Human-Robot Collaboration (HRC). This work focuses on optimizing the trajectory of the end-effector of a cobot arm in a collaborative industrial environment, ensuring the maximization of the operator’s safety and ergonomics without sacrificing production efficiency requirements. Hence, a multi-objective optimization strategy for trajectory planning in a safe and ergonomic HRC is defined. This approach aims at finding the best trade-off between the total traversal time of the cobot’s end-effector trajectory and ergonomics for the human worker, while respecting in the kinematic constraint of the optimization problem the ISO safety requirements through the well-known Speed and Separation Monitoring (SSM) methodology. Guaranteeing an ergonomic HRC means reducing musculoskeletal disorders linked to risky and highly repetitive activities. The three main phases of the proposed technique are described as follows. First, a manikin designed using a dedicated software is employed to evaluate the Rapid Upper Limb Assessment (RULA) ergonomic index in the working area. Next, a second-order cone programming problem is defined to represent a time-optimal safety compliant trajectory planning problem. Finally, the trajectory that ensures the best compromise between these two opposing goals –minimizing the task’s traversal time and maintaining a high level of ergonomics for the human worker– is computed by defining and solving a multi-objective control problem. The method is tested on an experimental case study in reference to an assembly task and the obtained results are discussed, showing the effectiveness of the proposed approach.
Note to Practitioners —Health and safety in workplaces are business imperatives, since they ensure not only a safe collaboration between industrial machinery and human operators, but also an increased productivity and flexibility of the entire industrial process. Hence, investing in health is a real driver for business growth. The key enabling technologies of Industry 4.0, such as collaborative robotics, exoskeletons, virtual and augmented reality, require standardization and indispensable technical safety requirements that cannot ignore physical, sensory, and psychological peculiarities of the human worker and aspects like usability and acceptability of these technologies in performing their activities. Against this ongoing industrial challenge, the aim of this paper is to provide researchers and practitioners with an innovative HRC trajectory planning methodology focused on enhancing production efficiency while respecting the SSM ISO safety requirement and guaranteeing the ergonomic optimal position of the operator during an assembly task. Therefore, the proposed methodology can be a convenient solution to be deployed in industrial companies, since it can support human operators by drastically reducing work-related musculoskeletal disorders and augmenting their performance in the working environment. Authors}, author_keywords = {cobots; Collaboration; Collaborative robotics; Ergonomics; ergonomics; human-robot collaboration (HRC); Optimization; rapid upper limb assessment (RULA); Robots; safety; Safety; speed and separation monitoring; time-optimal trajectory planning; Trajectory; Trajectory planning}, keywords = {Accident prevention; Augmented reality; Collaborative robots; Distributed computer systems; Economic and social effects; Ergonomics; Exoskeleton (Robotics); Industrial robots; Multiobjective optimization; Occupational risks; Production efficiency; Robot programming; Virtual reality; Collaboration; Human-robot collaboration; Optimisations; Rapid upper limb assessment; Rapid upper limb assessments; Speed and separation monitoring; Time-optimal trajectory planning; Trajectory Planning; Workers'; Trajectories}, type = {Article}, publication_stage = {Article in press}, source = {Scopus}, note = {Cited by: 0; All Open Access, Hybrid Gold Open Access} } - Prunella, M., Scardigno, R. M., Buongiorno, D., Brunetti, A., Longo, N., Carli, R., Dotoli, M. & Bevilacqua, V. (2023) Deep Learning for Automatic Vision-Based Recognition of Industrial Surface Defects: A Survey. IN IEEE Access, 11.43370 – 43423. doi:10.1109/ACCESS.2023.3271748
[BibTeX] [Abstract] [Download PDF]Automatic vision-based inspection systems have played a key role in product quality assessment for decades through the segmentation, detection, and classification of defects. Historically, machine learning frameworks, based on hand-crafted feature extraction, selection, and validation, counted on a combined approach of parameterized image processing algorithms and explicated human knowledge. The outstanding performance of deep learning (DL) for vision systems, in automatically discovering a feature representation suitable for the corresponding task, has exponentially increased the number of scientific articles and commercial products aiming at industrial quality assessment. In such a context, this article reviews more than 220 relevant articles from the related literature published until February 2023, covering the recent consolidation and advances in the field of fully-automatic DL-based surface defects inspection systems, deployed in various industrial applications. The analyzed papers have been classified according to a bi-dimensional taxonomy, that considers both the specific defect recognition task and the employed learning paradigm. The dependency on large and high-quality labeled datasets and the different neural architectures employed to achieve an overall perception of both well-visible and subtle defects, through the supervision of fine or/and coarse data annotations have been assessed. The results of our analysis highlight a growing research interest in defect representation power enrichment, especially by transferring pre-trained layers to an optimized network and by explaining the network decisions to suggest trustworthy retention or rejection of the products being evaluated. © 2013 IEEE.
@ARTICLE{Prunella202343370, author = {Prunella, Michela and Scardigno, Roberto Maria and Buongiorno, Domenico and Brunetti, Antonio and Longo, Nicola and Carli, Raffaele and Dotoli, Mariagrazia and Bevilacqua, Vitoantonio}, title = {Deep Learning for Automatic Vision-Based Recognition of Industrial Surface Defects: A Survey}, year = {2023}, journal = {IEEE Access}, volume = {11}, pages = {43370 – 43423}, doi = {10.1109/ACCESS.2023.3271748}, url = {https://www.scopus.com/inward/record.uri?eid=2-s2.0-85159721935&doi=10.1109%2fACCESS.2023.3271748&partnerID=40&md5=d488df827f296aca24ea29641932e0b3}, abstract = {Automatic vision-based inspection systems have played a key role in product quality assessment for decades through the segmentation, detection, and classification of defects. Historically, machine learning frameworks, based on hand-crafted feature extraction, selection, and validation, counted on a combined approach of parameterized image processing algorithms and explicated human knowledge. The outstanding performance of deep learning (DL) for vision systems, in automatically discovering a feature representation suitable for the corresponding task, has exponentially increased the number of scientific articles and commercial products aiming at industrial quality assessment. In such a context, this article reviews more than 220 relevant articles from the related literature published until February 2023, covering the recent consolidation and advances in the field of fully-automatic DL-based surface defects inspection systems, deployed in various industrial applications. The analyzed papers have been classified according to a bi-dimensional taxonomy, that considers both the specific defect recognition task and the employed learning paradigm. The dependency on large and high-quality labeled datasets and the different neural architectures employed to achieve an overall perception of both well-visible and subtle defects, through the supervision of fine or/and coarse data annotations have been assessed. The results of our analysis highlight a growing research interest in defect representation power enrichment, especially by transferring pre-trained layers to an optimized network and by explaining the network decisions to suggest trustworthy retention or rejection of the products being evaluated. © 2013 IEEE.}, author_keywords = {Artificial vision; auto-encoder; automatic recognition; convolutional neural network; deep learning; explainable artificial intelligence; feature attention mechanism; generative-adversarial network; industrial surface defects; transfer learning}, keywords = {Computer vision; Deep neural networks; Extraction; Generative adversarial networks; Image recognition; Image segmentation; Inspection; Inspection equipment; Network coding; Surface defects; Attention mechanisms; Auto encoders; Automatic recognition; Autonomous system; Convolutional neural network; Deep learning; Explainable artificial intelligence; Feature attention mechanism; Features extraction; Industrial surface defect; Manual; Transfer learning; Feature extraction}, type = {Article}, publication_stage = {Final}, source = {Scopus}, note = {Cited by: 21; All Open Access, Gold Open Access} }
- Proia, S., Cavone, G., Tresca, G., Carli, R. & Dotoli, M. (2023) Automatic Control of Drones’ Missions in a Hybrid Truck-Drone Delivery System IN 9th 2023 International Conference on Control, Decision and Information Technologies, CoDIT 2023., 1477 – 1482. doi:10.1109/CoDIT58514.2023.10284110
[BibTeX] [Abstract] [Download PDF]Last-mile delivery is one of the most discussed problems of the last decade due to the growing importance of e-commerce and the development of Industry 4.0. In particular, this problem regards the delivery of parcels from the warehouse to the final customers. In order to bring efficiency and innovation, in this paper a hybrid delivery architecture is considered, which takes advantage of the combined use of a drone and a truck to perform a sequence of pick-ups and deliveries, and the problem of optimal control of the drones’ missions is addressed. The reference scenario is the smart city where the drone of the hybrid delivery architecture is in charge of three different pick-up and delivery missions: truck to point (i.e., pick-up from the truck and delivery to the customer), point to point (i.e., delivery to a customer and pick-up from the subsequent customer), and point to truck (i.e., reentry from a customer to the truck). From the control point of view, the drone is optimally guided in all the operating modes, i.e., ascent and descent from/to truck mode, free flight mode with/without payload, and descent for pick-up/delivery mode, by a receding horizon linear quadratic regulator (LQR), which is able to manage the drone in the dynamic landing on a movable vehicle and to allow the changing in real time of the landing point on the truck. Simulation results of the truck-drone delivery architecture are presented and discussed in detail, proving the effectiveness of the proposed method. © 2023 IEEE.
@CONFERENCE{Proia20231477, author = {Proia, Silvia and Cavone, Graziana and Tresca, Giulia and Carli, Raffaele and Dotoli, Mariagrazia}, title = {Automatic Control of Drones' Missions in a Hybrid Truck-Drone Delivery System}, year = {2023}, journal = {9th 2023 International Conference on Control, Decision and Information Technologies, CoDIT 2023}, pages = {1477 – 1482}, doi = {10.1109/CoDIT58514.2023.10284110}, url = {https://www.scopus.com/inward/record.uri?eid=2-s2.0-85177429627&doi=10.1109%2fCoDIT58514.2023.10284110&partnerID=40&md5=01984a4e3bdb410678f081578de2dd3c}, abstract = {Last-mile delivery is one of the most discussed problems of the last decade due to the growing importance of e-commerce and the development of Industry 4.0. In particular, this problem regards the delivery of parcels from the warehouse to the final customers. In order to bring efficiency and innovation, in this paper a hybrid delivery architecture is considered, which takes advantage of the combined use of a drone and a truck to perform a sequence of pick-ups and deliveries, and the problem of optimal control of the drones' missions is addressed. The reference scenario is the smart city where the drone of the hybrid delivery architecture is in charge of three different pick-up and delivery missions: truck to point (i.e., pick-up from the truck and delivery to the customer), point to point (i.e., delivery to a customer and pick-up from the subsequent customer), and point to truck (i.e., reentry from a customer to the truck). From the control point of view, the drone is optimally guided in all the operating modes, i.e., ascent and descent from/to truck mode, free flight mode with/without payload, and descent for pick-up/delivery mode, by a receding horizon linear quadratic regulator (LQR), which is able to manage the drone in the dynamic landing on a movable vehicle and to allow the changing in real time of the landing point on the truck. Simulation results of the truck-drone delivery architecture are presented and discussed in detail, proving the effectiveness of the proposed method. © 2023 IEEE.}, author_keywords = {Aerial robotics; drones; last-mile delivery; receding horizon linear quadratic regulator; trajectory tracking}, keywords = {Aircraft detection; Antennas; Automation; Drones; Free flight; Pickups; Trucks; Aerial robotics; Delivery architecture; Hybrid delivery; Last mile; Last-mile delivery; Linear quadratic; Quadratic regulators; Receding horizon; Receding horizon linear quadratic regulator; Trajectory-tracking; Sales}, type = {Conference paper}, publication_stage = {Final}, source = {Scopus}, note = {Cited by: 1} }
- Atrigna, M., Buonanno, A., Carli, R., Cavone, G., Scarabaggio, P., Valenti, M., Graditi, G. & Dotoli, M. (2023) A Machine Learning Approach to Fault Prediction of Power Distribution Grids Under Heatwaves. IN IEEE Transactions on Industry Applications, 59.4835 – 4845. doi:10.1109/TIA.2023.3262230
[BibTeX] [Abstract] [Download PDF]Climate change is increasing the occurrence of the so-called heatwaves with a trend that is expected to worsen in the next years due to global warming. The growing intensity and duration of these extreme weather events are leading to a significant number of power system failures, especially in urban areas. This is drastically affecting the reliability and normal operation of power distribution grids around the world, with high financial costs and huge negative impacts on people’s life. Typically, the response to these failure events is approached by post-event analysis, aimed at identifying the grid areas that require resources to increase the resilience of the system and prevent future outages. Nevertheless, understanding the nature of heatwaves and forecasting their impact on power distribution systems can be useful to anticipate them and accelerate a reaction, possibly avoiding negative impacts on power systems and customers. In this study, a structured method to predict distribution grid disruptions caused by heatwaves is defined. The proposed method relies on machine learning to analyze previous failure data and forecast power grid outages using operational and meteorological information. The method is evaluated using real failure data from a large power distribution network located in southern Italy. © 1972-2012 IEEE.
@ARTICLE{Atrigna20234835, author = {Atrigna, Mauro and Buonanno, Amedeo and Carli, Raffaele and Cavone, Graziana and Scarabaggio, Paolo and Valenti, Maria and Graditi, Giorgio and Dotoli, Mariagrazia}, title = {A Machine Learning Approach to Fault Prediction of Power Distribution Grids Under Heatwaves}, year = {2023}, journal = {IEEE Transactions on Industry Applications}, volume = {59}, number = {4}, pages = {4835 – 4845}, doi = {10.1109/TIA.2023.3262230}, url = {https://www.scopus.com/inward/record.uri?eid=2-s2.0-85151511272&doi=10.1109%2fTIA.2023.3262230&partnerID=40&md5=89991feff02c7dffe5f98c9f90e0be87}, abstract = {Climate change is increasing the occurrence of the so-called heatwaves with a trend that is expected to worsen in the next years due to global warming. The growing intensity and duration of these extreme weather events are leading to a significant number of power system failures, especially in urban areas. This is drastically affecting the reliability and normal operation of power distribution grids around the world, with high financial costs and huge negative impacts on people's life. Typically, the response to these failure events is approached by post-event analysis, aimed at identifying the grid areas that require resources to increase the resilience of the system and prevent future outages. Nevertheless, understanding the nature of heatwaves and forecasting their impact on power distribution systems can be useful to anticipate them and accelerate a reaction, possibly avoiding negative impacts on power systems and customers. In this study, a structured method to predict distribution grid disruptions caused by heatwaves is defined. The proposed method relies on machine learning to analyze previous failure data and forecast power grid outages using operational and meteorological information. The method is evaluated using real failure data from a large power distribution network located in southern Italy. © 1972-2012 IEEE.}, author_keywords = {condition monitoring; fault prediction; heatwaves; machine learning; power system failures; Power system reliability}, keywords = {Artificial intelligence; Forecasting; Global warming; Learning systems; Outages; Fault prediction; Heating system; Heatwaves; Machine-learning; Power; Power cables; Power system; Power system failures; Power systems reliability; Resilience; Condition monitoring}, type = {Article}, publication_stage = {Final}, source = {Scopus}, note = {Cited by: 13} }
- Bozza, A., Cavone, G., Carli, R. & Dotoli, M. (2023) A Power Electronic Converters-Inspired Approach for Modeling PWM Switched-Based Nonlinear Hydraulic Servo Actuators IN Conference Proceedings – IEEE International Conference on Systems, Man and Cybernetics., 2477 – 2482. doi:10.1109/SMC53992.2023.10394065
[BibTeX] [Abstract] [Download PDF]This paper investigates a novel approach for properly modeling Hydraulic Servo Actuators (HSAs) based on ON-OFF switching valves. HSAs represent very high efficiency and small size-to-power ratio hydraulic actuators. Their functioning is guaranteed by their control system that ensures the desired flow-rate, and consequently, the proper pressure, to be provided to the actuator’s chambers. Nevertheless, achieving a good model of such HSAs for control purposes is non-trivial, due to their hybrid nature inherited from the switching between the different operating modes produced by valves. To overcome this limit, we propose an average equivalent discrete-time model of the chambers’ pressure dynamics related to a single control input for the digital valves. The proposed model takes inspiration from the analogy existing between hydraulic systems and power electronic converters, and guarantees the same performance as the traditional model, with the advantage of greatly simplifying the control of the servo actuator. Finally, the consistency of the proposed model with respect to its nonlinear hybrid version is proved via numerical examples. © 2023 IEEE.
@CONFERENCE{Bozza20232477, author = {Bozza, Augusto and Cavone, Graziana and Carli, Raffaele and Dotoli, Mariagrazia}, title = {A Power Electronic Converters-Inspired Approach for Modeling PWM Switched-Based Nonlinear Hydraulic Servo Actuators}, year = {2023}, journal = {Conference Proceedings - IEEE International Conference on Systems, Man and Cybernetics}, pages = {2477 – 2482}, doi = {10.1109/SMC53992.2023.10394065}, url = {https://www.scopus.com/inward/record.uri?eid=2-s2.0-85187260571&doi=10.1109%2fSMC53992.2023.10394065&partnerID=40&md5=ba0c9a7b491927b01db552a62bd2d7de}, abstract = {This paper investigates a novel approach for properly modeling Hydraulic Servo Actuators (HSAs) based on ON-OFF switching valves. HSAs represent very high efficiency and small size-to-power ratio hydraulic actuators. Their functioning is guaranteed by their control system that ensures the desired flow-rate, and consequently, the proper pressure, to be provided to the actuator's chambers. Nevertheless, achieving a good model of such HSAs for control purposes is non-trivial, due to their hybrid nature inherited from the switching between the different operating modes produced by valves. To overcome this limit, we propose an average equivalent discrete-time model of the chambers' pressure dynamics related to a single control input for the digital valves. The proposed model takes inspiration from the analogy existing between hydraulic systems and power electronic converters, and guarantees the same performance as the traditional model, with the advantage of greatly simplifying the control of the servo actuator. Finally, the consistency of the proposed model with respect to its nonlinear hybrid version is proved via numerical examples. © 2023 IEEE.}, author_keywords = {Electro-Hydraulic Analogy; Hybrid Systems; Hydraulic Servo Actuators; Nonlinear Systems; Pulse Width Modulation (PWM); Switching Systems}, keywords = {Hydraulic actuators; Hydraulic equipment; Power converters; Power electronics; Pulse width modulation; Switching systems; Control purpose; Electro-hydraulic analogy; Electro-hydraulics; Higher efficiency; Hydraulic servo actuator; Non-trivial; Power electronics converters; Power ratio; Pulse width modulation; Pulsewidth modulations (PWM); Hybrid systems}, type = {Conference paper}, publication_stage = {Final}, source = {Scopus}, note = {Cited by: 1} }
- Cavone, G., Stella, S., Scarabaggio, P., Carli, R., Lisi, S., Garavelli, A. C. & Dotoli, M. (2023) A Colored Petri Net Tool for the Design of Robotic Palletizing Cells IN 9th 2023 International Conference on Control, Decision and Information Technologies, CoDIT 2023., 12 – 17. doi:10.1109/CoDIT58514.2023.10284186
[BibTeX] [Abstract] [Download PDF]Driven by the digital transformation required by Logistics 4.0, the use of automation in warehouses is constantly growing. In particular, robotic palletizers offer significant potential for optimizing warehouse operations, thanks to higher flexibility and throughput than traditional palletizing systems. Despite the availability of several solutions in the market, the optimal deployment of a robotic palletizer in warehouses is not straightforward: a design phase is needed to determine the most convenient configuration that ensures automatic palletizing is fully integrated into the warehouse processes. In this paper, we propose a simulation-based versatile tool for modeling and analysis purposes, aimed at supporting the design of a robotic palletizing cell in a bottom-up fashion. As a core methodology, we employ timed colored Petri nets, which allow – once the analysis on packing requirements and constraints is conducted – to rapidly model the system as a composition of basic subsystems, and implement alternative simulations to evaluate the corresponding performance and effectively benchmark the alternative configurations. The proposed approach is applied to a real case study, showing its effectiveness in identifying the solution that achieves a good compromise between the use of resources and the performance of warehouse operations. © 2023 IEEE.
@CONFERENCE{Cavone202312, author = {Cavone, Graziana and Stella, Silvia and Scarabaggio, Paolo and Carli, Raffaele and Lisi, Stefano and Garavelli, Achille Claudio and Dotoli, Mariagrazia}, title = {A Colored Petri Net Tool for the Design of Robotic Palletizing Cells}, year = {2023}, journal = {9th 2023 International Conference on Control, Decision and Information Technologies, CoDIT 2023}, pages = {12 – 17}, doi = {10.1109/CoDIT58514.2023.10284186}, url = {https://www.scopus.com/inward/record.uri?eid=2-s2.0-85177446511&doi=10.1109%2fCoDIT58514.2023.10284186&partnerID=40&md5=fb1f951ba12c45828607bf01cf994fff}, abstract = {Driven by the digital transformation required by Logistics 4.0, the use of automation in warehouses is constantly growing. In particular, robotic palletizers offer significant potential for optimizing warehouse operations, thanks to higher flexibility and throughput than traditional palletizing systems. Despite the availability of several solutions in the market, the optimal deployment of a robotic palletizer in warehouses is not straightforward: a design phase is needed to determine the most convenient configuration that ensures automatic palletizing is fully integrated into the warehouse processes. In this paper, we propose a simulation-based versatile tool for modeling and analysis purposes, aimed at supporting the design of a robotic palletizing cell in a bottom-up fashion. As a core methodology, we employ timed colored Petri nets, which allow - once the analysis on packing requirements and constraints is conducted - to rapidly model the system as a composition of basic subsystems, and implement alternative simulations to evaluate the corresponding performance and effectively benchmark the alternative configurations. The proposed approach is applied to a real case study, showing its effectiveness in identifying the solution that achieves a good compromise between the use of resources and the performance of warehouse operations. © 2023 IEEE.}, author_keywords = {Colored Petri net; Discrete Event Systems; Modeling and Simulation; Robotic Palletizing System}, keywords = {Benchmarking; Petri nets; Robotics; Warehouses; Colored Petri Nets; Digital transformation; Discrete events systems; High-throughput; Model and simulation; Performance; Robotic palletizers; Robotic palletizing system; Robotic-palletizing cell; Warehouse operation; Discrete event simulation}, type = {Conference paper}, publication_stage = {Final}, source = {Scopus}, note = {Cited by: 0} }
- Tresca, G., Cavone, G., Carli, R. & Dotoli, M. (2023) A mathematical model for the optimal configuration of automated storage systems with sliding trays IN 2023 European Control Conference, ECC 2023.. doi:10.23919/ECC57647.2023.10178251
[BibTeX] [Abstract] [Download PDF]This work aims at contributing to the advancement of Logistics 4.0, focusing on the management of the storage of goods. The goal is to solve the complex problem of efficiently and rapidly configuring Vertical Lift Modules (VLMs) with sliding trays in automated warehouses. This problem is still barely discussed in the related literature and most contributions mainly focus on the optimization of the VLM throughput instead of trays allocation and respective items configuration. To fill this gap, this work proposes a novel mathematical model that allows to properly represent and solve this complex problem, taking into account practical logistic constraints. The problem is defined as a mixed integer non-linear programming model, which is validated on realistic scenarios. Further, a scalability analysis is performed to evaluate its performance even in complex scenarios. The obtained results demonstrate the effectiveness of the model in defining space efficient configurations in short computation time. © 2023 EUCA.
@CONFERENCE{Tresca2023, author = {Tresca, Giulia and Cavone, Graziana and Carli, Raffaele and Dotoli, Mariagrazia}, title = {A mathematical model for the optimal configuration of automated storage systems with sliding trays}, year = {2023}, journal = {2023 European Control Conference, ECC 2023}, doi = {10.23919/ECC57647.2023.10178251}, url = {https://www.scopus.com/inward/record.uri?eid=2-s2.0-85166474973&doi=10.23919%2fECC57647.2023.10178251&partnerID=40&md5=7a7e0c93dea4f182cc0bc9d38c8350a3}, abstract = {This work aims at contributing to the advancement of Logistics 4.0, focusing on the management of the storage of goods. The goal is to solve the complex problem of efficiently and rapidly configuring Vertical Lift Modules (VLMs) with sliding trays in automated warehouses. This problem is still barely discussed in the related literature and most contributions mainly focus on the optimization of the VLM throughput instead of trays allocation and respective items configuration. To fill this gap, this work proposes a novel mathematical model that allows to properly represent and solve this complex problem, taking into account practical logistic constraints. The problem is defined as a mixed integer non-linear programming model, which is validated on realistic scenarios. Further, a scalability analysis is performed to evaluate its performance even in complex scenarios. The obtained results demonstrate the effectiveness of the model in defining space efficient configurations in short computation time. © 2023 EUCA.}, keywords = {Nonlinear programming; Automated storage; Automated warehouse; Complex problems; Linear programming models; Logistics constraints; Mixed-integer nonlinear programming; Optimisations; Realistic scenario; Storage systems; Vertical lift modules; Integer programming}, type = {Conference paper}, publication_stage = {Final}, source = {Scopus}, note = {Cited by: 0} }
2022
- Scarabaggio, P., Carli, R., Parisio, A. & Dotoli, M. (2022) On Controlling Battery Degradation in Vehicle-to-Grid Energy Markets IN IEEE International Conference on Automation Science and Engineering., 1206 – 1211. doi:10.1109/CASE49997.2022.9926729
[BibTeX] [Abstract] [Download PDF]Nowadays, power grids are facing reduced total system inertia as traditional generators are phased out in favor of renewable energy sources. This issue is expected to deepen with the increasing penetration of electric vehicles (EVs). The influence of a single EV on power networks is low; nevertheless, the aggregate impact becomes relevant when they are properly coordinated. In this context, we consider the frequent case of a group of EVs connected to a parking lot with a photovoltaic facility. We propose a novel strategy to optimally control their batteries during the parking session, which is able to satisfy their requirements and energy constraints. EVs participate in a noncooperative energy market based on a smart pricing mechanism that is designed in order to increase the predictability and flexibility of the aggregate parking load. Differently from the existing contributions, we employ a novel approach to minimize the degradation of batteries. The effectiveness of the proposed method is validated through numerical experiments based on a real scenario. © 2022 IEEE.
@CONFERENCE{Scarabaggio20221206, author = {Scarabaggio, Paolo and Carli, Raffaele and Parisio, Alessandra and Dotoli, Mariagrazia}, title = {On Controlling Battery Degradation in Vehicle-to-Grid Energy Markets}, year = {2022}, journal = {IEEE International Conference on Automation Science and Engineering}, volume = {2022-August}, pages = {1206 – 1211}, doi = {10.1109/CASE49997.2022.9926729}, url = {https://www.scopus.com/inward/record.uri?eid=2-s2.0-85141672066&doi=10.1109%2fCASE49997.2022.9926729&partnerID=40&md5=ed24721222037df90795b0e4d7a1cbb1}, abstract = {Nowadays, power grids are facing reduced total system inertia as traditional generators are phased out in favor of renewable energy sources. This issue is expected to deepen with the increasing penetration of electric vehicles (EVs). The influence of a single EV on power networks is low; nevertheless, the aggregate impact becomes relevant when they are properly coordinated. In this context, we consider the frequent case of a group of EVs connected to a parking lot with a photovoltaic facility. We propose a novel strategy to optimally control their batteries during the parking session, which is able to satisfy their requirements and energy constraints. EVs participate in a noncooperative energy market based on a smart pricing mechanism that is designed in order to increase the predictability and flexibility of the aggregate parking load. Differently from the existing contributions, we employ a novel approach to minimize the degradation of batteries. The effectiveness of the proposed method is validated through numerical experiments based on a real scenario. © 2022 IEEE.}, author_keywords = {charging scheduling; Electric vehicles; model predictive control}, keywords = {Aggregates; Charging (batteries); Electric power transmission networks; Electric vehicles; Numerical methods; Renewable energy resources; Secondary batteries; Vehicle-to-grid; Battery degradation; Charging scheduling; Energy markets; Model-predictive control; Parking lots; Power grids; Power networks; Renewable energy source; System inertia; Vehicle to grids; Model predictive control}, type = {Conference paper}, publication_stage = {Final}, source = {Scopus}, note = {Cited by: 0} }
- Mohsen Hosseini, S., Carli, R., Jantzen, J. & Dotoli, M. (2022) Multi-block ADMM Approach for Decentralized Demand Response of Energy Communities with Flexible Loads and Shared Energy Storage System IN 2022 30th Mediterranean Conference on Control and Automation, MED 2022., 67 – 72. doi:10.1109/MED54222.2022.9837173
[BibTeX] [Abstract] [Download PDF]This paper proposes a novel decentralized energy scheduling framework for demand response of energy communities in the case of limited overall capacity of distribution networks. A combined energy scheduling of heating, ventilation, and air conditioning systems and a community energy storage system (CESS) for multiple smart residential users is presented. The proposed approach aims at minimizing the total expected energy costs while ensuring the occupants’ thermal comfort. The optimization problem is first formulated as a mixed-integer linear programming problem, which is converted into a linear programming problem using a tractable approximation method based on a non-complementary charging/discharging strategy of the CESS. The decentralized resolution process is based on multi-block proximal Jacobian alternating direction method of multipliers, ensuring efficient computation and protecting users’ privacy. We assess the effectiveness of the proposed approach through numerical experiments on a realistic case study. © 2022 IEEE.
@CONFERENCE{Mohsen Hosseini202267, author = {Mohsen Hosseini, Seyed and Carli, Raffaele and Jantzen, Jan and Dotoli, Mariagrazia}, title = {Multi-block ADMM Approach for Decentralized Demand Response of Energy Communities with Flexible Loads and Shared Energy Storage System}, year = {2022}, journal = {2022 30th Mediterranean Conference on Control and Automation, MED 2022}, pages = {67 – 72}, doi = {10.1109/MED54222.2022.9837173}, url = {https://www.scopus.com/inward/record.uri?eid=2-s2.0-85136256232&doi=10.1109%2fMED54222.2022.9837173&partnerID=40&md5=05482ee51ee4bd7730a9109693de5802}, abstract = {This paper proposes a novel decentralized energy scheduling framework for demand response of energy communities in the case of limited overall capacity of distribution networks. A combined energy scheduling of heating, ventilation, and air conditioning systems and a community energy storage system (CESS) for multiple smart residential users is presented. The proposed approach aims at minimizing the total expected energy costs while ensuring the occupants' thermal comfort. The optimization problem is first formulated as a mixed-integer linear programming problem, which is converted into a linear programming problem using a tractable approximation method based on a non-complementary charging/discharging strategy of the CESS. The decentralized resolution process is based on multi-block proximal Jacobian alternating direction method of multipliers, ensuring efficient computation and protecting users' privacy. We assess the effectiveness of the proposed approach through numerical experiments on a realistic case study. © 2022 IEEE.}, author_keywords = {alternating direction method of multipliers (ADMM); decentralized control; demand response; energy communities; energy storage}, keywords = {Air conditioning; Decentralized control; Integer programming; Scheduling; Alternating direction method of multiplier; Alternating directions method of multipliers; Decentralised; Decentralised control; Demand response; Energy; Energy community; Multiblocks; Response of energy; Storage systems; Energy storage}, type = {Conference paper}, publication_stage = {Final}, source = {Scopus}, note = {Cited by: 12} }
- Proia, S., Carli, R., Cavone, G. & Dotoli, M. (2022) Control Techniques for Safe, Ergonomic, and Efficient Human-Robot Collaboration in the Digital Industry: A Survey. IN IEEE Transactions on Automation Science and Engineering, 19.1798 – 1819. doi:10.1109/TASE.2021.3131011
[BibTeX] [Abstract] [Download PDF]The fourth industrial revolution, also known as Industry 4.0, is reshaping the way individuals live and work while providing a substantial influence on the manufacturing scenario. The key enabling technology that has made Industry 4.0 a concrete reality is without doubt collaborative robotics, which is also evolving as a fundamental pillar of the next revolution, the so-called Industry 5.0. The improvement of employees’ safety and well-being, together with the increase of profitability and productivity, are indeed the main goals of human-robot collaboration (HRC) in the industrial setting. The robotic controller design and the analysis of existing decision and control techniques are crucially needed to develop innovative models and state-of-the-art methodologies for a safe, ergonomic, and efficient HRC. To this aim, this paper presents an accurate review of the most recent and relevant contributions to the related literature, focusing on the control perspective. All the surveyed works are carefully selected and categorized by target (i.e., safety, ergonomics, and efficiency), and then by problem and type of control, in presence or absence of optimization. Finally, the discussion of the achieved results and the analysis of the emerging challenges in this research field are reported, highlighting the identified gaps and the promising future developments in the context of the digital evolution. © 2004-2012 IEEE.
@ARTICLE{Proia20221798, author = {Proia, Silvia and Carli, Raffaele and Cavone, Graziana and Dotoli, Mariagrazia}, title = {Control Techniques for Safe, Ergonomic, and Efficient Human-Robot Collaboration in the Digital Industry: A Survey}, year = {2022}, journal = {IEEE Transactions on Automation Science and Engineering}, volume = {19}, number = {3}, pages = {1798 – 1819}, doi = {10.1109/TASE.2021.3131011}, url = {https://www.scopus.com/inward/record.uri?eid=2-s2.0-85121365732&doi=10.1109%2fTASE.2021.3131011&partnerID=40&md5=eae23f3b0dbfaf83b934942c9c0736ff}, abstract = {The fourth industrial revolution, also known as Industry 4.0, is reshaping the way individuals live and work while providing a substantial influence on the manufacturing scenario. The key enabling technology that has made Industry 4.0 a concrete reality is without doubt collaborative robotics, which is also evolving as a fundamental pillar of the next revolution, the so-called Industry 5.0. The improvement of employees' safety and well-being, together with the increase of profitability and productivity, are indeed the main goals of human-robot collaboration (HRC) in the industrial setting. The robotic controller design and the analysis of existing decision and control techniques are crucially needed to develop innovative models and state-of-the-art methodologies for a safe, ergonomic, and efficient HRC. To this aim, this paper presents an accurate review of the most recent and relevant contributions to the related literature, focusing on the control perspective. All the surveyed works are carefully selected and categorized by target (i.e., safety, ergonomics, and efficiency), and then by problem and type of control, in presence or absence of optimization. Finally, the discussion of the achieved results and the analysis of the emerging challenges in this research field are reported, highlighting the identified gaps and the promising future developments in the context of the digital evolution. © 2004-2012 IEEE.}, author_keywords = {cobots; collaborative robotics; efficiency; ergonomics; HRC control systems; human-robot collaboration (HRC); industrial automation; Industry 4.0; safety}, keywords = {Collaborative robots; Controllers; Efficiency; Ergonomics; Industry 4.0; Job analysis; Robotics; Surveys; Collaboration; Control techniques; Efficiency.; Human-robot collaboration; Human-robot collaboration control system; Industrial automation; Service robots; Task analysis; Accident prevention}, type = {Article}, publication_stage = {Final}, source = {Scopus}, note = {Cited by: 68; All Open Access, Hybrid Gold Open Access} }
- Dammacco, L., Carli, R., Lazazzera, V., Fiorentino, M. & Dotoli, M. (2022) Simulation-based Design for the Layout and Operation of AGVs in Sustainable and Efficient Manufacturing Systems IN Proceedings of the International Conference on Cyber-Physical Social Intelligence, ICCSI 2022., 309 – 314. doi:10.1109/ICCSI55536.2022.9970620
[BibTeX] [Abstract] [Download PDF]Complex manufacturing systems are recently undergoing a green revolution due to manufacturing customization towards sustainable products. A key enabler for the implementation of green and energy efficient production is simulation-based design, which supports system engineers and designers in making decision choices aimed at enhancing the performance of smart and sustainable production. In this context, this work proposes a simulation approach to support the design of the layout and operation of automated guided vehicles (AGVs) in complex production lines. In particular, a case study related to the assembly of electric axles for heavy-duty vehicles is presented: a scenario analysis implemented in the Plant Simulation platform is used to determine the optimal configuration of AGVs in terms of number of vehicles and operation (e.g., definition of charging strategies, scheduling of charging stops, routing). The simulation results first show that the reduction of the AGVs’ energy consumption and the increase of production throughput are competing criteria; second, the choice of AGV charging strategies has a significant influence on the energy consumption as well as on the productivity performance. © 2022 IEEE.
@CONFERENCE{Dammacco2022309, author = {Dammacco, Lucilla and Carli, Raffaele and Lazazzera, Vito and Fiorentino, Michele and Dotoli, Mariagrazia}, title = {Simulation-based Design for the Layout and Operation of AGVs in Sustainable and Efficient Manufacturing Systems}, year = {2022}, journal = {Proceedings of the International Conference on Cyber-Physical Social Intelligence, ICCSI 2022}, pages = {309 – 314}, doi = {10.1109/ICCSI55536.2022.9970620}, url = {https://www.scopus.com/inward/record.uri?eid=2-s2.0-85145662907&doi=10.1109%2fICCSI55536.2022.9970620&partnerID=40&md5=29f65c54294fd15458b294507bca7628}, abstract = {Complex manufacturing systems are recently undergoing a green revolution due to manufacturing customization towards sustainable products. A key enabler for the implementation of green and energy efficient production is simulation-based design, which supports system engineers and designers in making decision choices aimed at enhancing the performance of smart and sustainable production. In this context, this work proposes a simulation approach to support the design of the layout and operation of automated guided vehicles (AGVs) in complex production lines. In particular, a case study related to the assembly of electric axles for heavy-duty vehicles is presented: a scenario analysis implemented in the Plant Simulation platform is used to determine the optimal configuration of AGVs in terms of number of vehicles and operation (e.g., definition of charging strategies, scheduling of charging stops, routing). The simulation results first show that the reduction of the AGVs' energy consumption and the increase of production throughput are competing criteria; second, the choice of AGV charging strategies has a significant influence on the energy consumption as well as on the productivity performance. © 2022 IEEE.}, author_keywords = {automated guided vehicle (AGV); Complex manufacturing systems; discrete event simulation; sustainable and efficient manufacturing; what-if analysis}, keywords = {Automatic guided vehicles; Energy efficiency; Energy utilization; Simulation platform; Throughput; Automated guided vehicle; Automated guided vehicles; Charging strategies; Complex manufacturing systems; Customisation; Discrete-event simulations; Green revolution; Simulation-based designs; Sustainable and efficient manufacturing; What-if Analysis; Discrete event simulation}, type = {Conference paper}, publication_stage = {Final}, source = {Scopus}, note = {Cited by: 3} }
- Helmi, A. M., Carli, R., Dotoli, M. & Ramadan, H. S. (2022) Efficient and Sustainable Reconfiguration of Distribution Networks via Metaheuristic Optimization. IN IEEE Transactions on Automation Science and Engineering, 19.82 – 98. doi:10.1109/TASE.2021.3072862
[BibTeX] [Abstract] [Download PDF]Improving the efficiency and sustainability of distribution networks (DNs) is nowadays a challenging objective both for large networks and microgrids connected to the main grid. In this context, a crucial role is played by the so-called network reconfiguration problem, which aims at determining the optimal DN topology. This process is enabled by properly changing the close/open status of all available branch switches to form an admissible graph connecting network buses. The reconfiguration problem is typically modeled as an NP-hard combinatorial problem with a complex search space due to current and voltage constraints. Even though several metaheuristic algorithms have been used to obtain – without guarantees – the global optimal solution, searching for near-optimal solutions in reasonable time is still a research challenge for the DN reconfiguration problem. Facing this issue, this article proposes a novel effective optimization framework for the reconfiguration problem of modern DNs. The objective of reconfiguration is minimizing the overall power losses while ensuring an enhanced DN voltage profile. A multiple-step resolution procedure is then presented, where the recent Harris hawks optimization (HHO) algorithm constitutes the core part. This optimizer is here intelligently accompanied by appropriate preprocessing (i.e., search space preparation and initial feasible population generation) and postprocessing (i.e., solution refinement) phases aimed at improving the search for near-optimal configurations. The effectiveness of the method is validated through numerical experiments on the IEEE 33-bus, the IEEE 85-bus systems, and an artificial 295-bus system under distributed generation and load variation. Finally, the performance of the proposed HHO-based approach is compared with two related metaheuristic techniques, namely the particle swarm optimization algorithm and the Cuckoo search algorithm. The results show that HHO outperforms the other two optimizers in terms of minimized power losses, enhanced voltage profile, and running time. Note to Practitioners – This article is motivated by the emerging need for effective network reconfiguration approaches in modern power distribution systems, including microgrids. The proposed metaheuristic optimization strategy allows the decision maker (i.e., the distribution system operator) to determine in reasonable time the optimal network topology, minimizing the overall power losses and considering the system operational requirements. The proposed optimization framework is generic and flexible, as it can be applied to different architectures both of large distribution networks (DNs) and microgrids, considering various types of system objectives and technical constraints. The presented strategy can be implemented in any decision support system or engineering software for power grids, providing decision makers with an effective information and communication technology tool for the optimal planning of the energy efficiency and environmental sustainability of DNs. © 2004-2012 IEEE.
@ARTICLE{Helmi202282, author = {Helmi, Ahmed M. and Carli, Raffaele and Dotoli, Mariagrazia and Ramadan, Haitham S.}, title = {Efficient and Sustainable Reconfiguration of Distribution Networks via Metaheuristic Optimization}, year = {2022}, journal = {IEEE Transactions on Automation Science and Engineering}, volume = {19}, number = {1}, pages = {82 – 98}, doi = {10.1109/TASE.2021.3072862}, url = {https://www.scopus.com/inward/record.uri?eid=2-s2.0-85105879516&doi=10.1109%2fTASE.2021.3072862&partnerID=40&md5=1b9b5290b92a3a5ad8886f61ec903dc8}, abstract = {Improving the efficiency and sustainability of distribution networks (DNs) is nowadays a challenging objective both for large networks and microgrids connected to the main grid. In this context, a crucial role is played by the so-called network reconfiguration problem, which aims at determining the optimal DN topology. This process is enabled by properly changing the close/open status of all available branch switches to form an admissible graph connecting network buses. The reconfiguration problem is typically modeled as an NP-hard combinatorial problem with a complex search space due to current and voltage constraints. Even though several metaheuristic algorithms have been used to obtain - without guarantees - the global optimal solution, searching for near-optimal solutions in reasonable time is still a research challenge for the DN reconfiguration problem. Facing this issue, this article proposes a novel effective optimization framework for the reconfiguration problem of modern DNs. The objective of reconfiguration is minimizing the overall power losses while ensuring an enhanced DN voltage profile. A multiple-step resolution procedure is then presented, where the recent Harris hawks optimization (HHO) algorithm constitutes the core part. This optimizer is here intelligently accompanied by appropriate preprocessing (i.e., search space preparation and initial feasible population generation) and postprocessing (i.e., solution refinement) phases aimed at improving the search for near-optimal configurations. The effectiveness of the method is validated through numerical experiments on the IEEE 33-bus, the IEEE 85-bus systems, and an artificial 295-bus system under distributed generation and load variation. Finally, the performance of the proposed HHO-based approach is compared with two related metaheuristic techniques, namely the particle swarm optimization algorithm and the Cuckoo search algorithm. The results show that HHO outperforms the other two optimizers in terms of minimized power losses, enhanced voltage profile, and running time. Note to Practitioners - This article is motivated by the emerging need for effective network reconfiguration approaches in modern power distribution systems, including microgrids. The proposed metaheuristic optimization strategy allows the decision maker (i.e., the distribution system operator) to determine in reasonable time the optimal network topology, minimizing the overall power losses and considering the system operational requirements. The proposed optimization framework is generic and flexible, as it can be applied to different architectures both of large distribution networks (DNs) and microgrids, considering various types of system objectives and technical constraints. The presented strategy can be implemented in any decision support system or engineering software for power grids, providing decision makers with an effective information and communication technology tool for the optimal planning of the energy efficiency and environmental sustainability of DNs. © 2004-2012 IEEE.}, author_keywords = {Distribution network (DN) reconfiguration; Harris hawks optimization (HHO) algorithm; metaheuristic optimization; microgrids; power losses reduction; voltage profile improvement}, keywords = {Microgrids; NP-hard; Numerical methods; Optimal systems; Particle swarm optimization (PSO); Cuckoo search algorithms; Global optimal solutions; Meta heuristic algorithm; Meta-heuristic optimizations; Meta-heuristic techniques; Network re-configuration; Particle swarm optimization algorithm; Reconfiguration of distribution networks; Internet protocols}, type = {Article}, publication_stage = {Final}, source = {Scopus}, note = {Cited by: 88} }
- Dammacco, L., Carli, R., Lazazzera, V., Fiorentino, M. & Dotoli, M. (2022) Designing complex manufacturing systems by virtual reality: A novel approach and its application to the virtual commissioning of a production line. IN Computers in Industry, 143.. doi:10.1016/j.compind.2022.103761
[BibTeX] [Abstract] [Download PDF]The design of complex manufacturing systems (CMSs) is challenging, because of the requirements of efficiency, safety, and ergonomics, and the need of optimizing resources, i.e., space, machines, operators, and data. Virtual reality (VR) – one of the promising technologies at the base of Industry 4.0 – is able to address the design issues of CMSs, and even decrease costs and time when employed from the initial conception to the final validation of production lines, since it facilitates their virtual commissioning, i.e., it enables the full verification of systems and related components by virtual inspection and tests. Despite the above advantages, VR is still rarely used in the design of CMSs, and there is no standard VR approach in industry yet. In addition, the related scientific literature is scarce and often limited to small or simplified cases. To fill this gap, this work presents a novel VR-based approach for designing CMSs, composed of four phases: Three-dimensional CAD Export, Model Import, Scene Creation, and VR Review. The proposed approach is applied to a real industrial use case related to the virtual commissioning of an electric axles production line and it is evaluated through a questionnaire from industry professionals. The case study shows that using the VR technology enhanced the technical communication between experts in the teamwork, and it was particularly effective in finding ergonomics flaws like issues in visibility, reach, and posture using a virtual golden zone. In addition, all users found the VR interaction enjoyable and easy to learn, and beginner users perceived a comparable workload as advanced users. © 2022 Elsevier B.V.
@ARTICLE{Dammacco2022, author = {Dammacco, Lucilla and Carli, Raffaele and Lazazzera, Vito and Fiorentino, Michele and Dotoli, Mariagrazia}, title = {Designing complex manufacturing systems by virtual reality: A novel approach and its application to the virtual commissioning of a production line}, year = {2022}, journal = {Computers in Industry}, volume = {143}, doi = {10.1016/j.compind.2022.103761}, url = {https://www.scopus.com/inward/record.uri?eid=2-s2.0-85136109989&doi=10.1016%2fj.compind.2022.103761&partnerID=40&md5=c803a45d690d457ee75499235ee266ec}, abstract = {The design of complex manufacturing systems (CMSs) is challenging, because of the requirements of efficiency, safety, and ergonomics, and the need of optimizing resources, i.e., space, machines, operators, and data. Virtual reality (VR) – one of the promising technologies at the base of Industry 4.0 – is able to address the design issues of CMSs, and even decrease costs and time when employed from the initial conception to the final validation of production lines, since it facilitates their virtual commissioning, i.e., it enables the full verification of systems and related components by virtual inspection and tests. Despite the above advantages, VR is still rarely used in the design of CMSs, and there is no standard VR approach in industry yet. In addition, the related scientific literature is scarce and often limited to small or simplified cases. To fill this gap, this work presents a novel VR-based approach for designing CMSs, composed of four phases: Three-dimensional CAD Export, Model Import, Scene Creation, and VR Review. The proposed approach is applied to a real industrial use case related to the virtual commissioning of an electric axles production line and it is evaluated through a questionnaire from industry professionals. The case study shows that using the VR technology enhanced the technical communication between experts in the teamwork, and it was particularly effective in finding ergonomics flaws like issues in visibility, reach, and posture using a virtual golden zone. In addition, all users found the VR interaction enjoyable and easy to learn, and beginner users perceived a comparable workload as advanced users. © 2022 Elsevier B.V.}, author_keywords = {Complex manufacturing systems; Human computer interaction; Industry 4.0; System design; Virtual commissioning; Virtual reality}, keywords = {Computer aided design; Electric lines; Human computer interaction; Industry 4.0; Virtual addresses; Complex manufacturing systems; Design issues; Designing complex; ITS applications; Machine data; Machine operators; Production line; Virtual commissioning; Virtual inspection; Virtual tests; Virtual reality}, type = {Article}, publication_stage = {Final}, source = {Scopus}, note = {Cited by: 21} }
- Scarabaggio, P., Carli, R., Cavone, G., Epicoco, N. & Dotoli, M. (2022) Nonpharmaceutical Stochastic Optimal Control Strategies to Mitigate the COVID-19 Spread. IN IEEE Transactions on Automation Science and Engineering, 19.560 – 575. doi:10.1109/TASE.2021.3111338
[BibTeX] [Abstract] [Download PDF]This article proposes a stochastic nonlinear model predictive controller to support policymakers in determining robust optimal nonpharmaceutical strategies to tackle the COVID-19 pandemic waves. First, a time-varying SIRCQTHE epidemiological model is defined to get predictions on the pandemic dynamics. A stochastic model predictive control problem is then formulated to select the necessary control actions (i.e., restrictions on the mobility for different socioeconomic categories) to minimize the socioeconomic costs. In particular, considering the uncertainty characterizing this decision-making process, we ensure that the capacity of the healthcare system is not violated in accordance with a chance constraint approach. The effectiveness of the presented method in properly supporting the definition of diversified nonpharmaceutical strategies for tackling the COVID-19 spread is tested on the network of Italian regions using real data. The proposed approach can be easily extended to cope with other countries’ characteristics and different levels of the spatial scale. Note to Practitioners – This article is motivated by the emerging need for developing effective methods to support policymakers in mitigating the effects of the COVID-19 pandemic. The proposed feedback control strategy – combining a multiregion epidemiological model with a nonlinear stochastic model predictive control approach – allows the robust identification of the most effective restrictive measures considering the corresponding effects on the healthcare and socioeconomic systems. The proposed framework is a general and flexible method that can be applied to various real scenarios, leveraging mobility data, available from the Google mobility service, to recognize patterns and predict future behaviors of individuals. © 2004-2012 IEEE.
@ARTICLE{Scarabaggio2022560, author = {Scarabaggio, Paolo and Carli, Raffaele and Cavone, Graziana and Epicoco, Nicola and Dotoli, Mariagrazia}, title = {Nonpharmaceutical Stochastic Optimal Control Strategies to Mitigate the COVID-19 Spread}, year = {2022}, journal = {IEEE Transactions on Automation Science and Engineering}, volume = {19}, number = {2}, pages = {560 – 575}, doi = {10.1109/TASE.2021.3111338}, url = {https://www.scopus.com/inward/record.uri?eid=2-s2.0-85115686920&doi=10.1109%2fTASE.2021.3111338&partnerID=40&md5=ef02a3b0f8f2eeff0ec2f0e0a861a0b8}, abstract = {This article proposes a stochastic nonlinear model predictive controller to support policymakers in determining robust optimal nonpharmaceutical strategies to tackle the COVID-19 pandemic waves. First, a time-varying SIRCQTHE epidemiological model is defined to get predictions on the pandemic dynamics. A stochastic model predictive control problem is then formulated to select the necessary control actions (i.e., restrictions on the mobility for different socioeconomic categories) to minimize the socioeconomic costs. In particular, considering the uncertainty characterizing this decision-making process, we ensure that the capacity of the healthcare system is not violated in accordance with a chance constraint approach. The effectiveness of the presented method in properly supporting the definition of diversified nonpharmaceutical strategies for tackling the COVID-19 spread is tested on the network of Italian regions using real data. The proposed approach can be easily extended to cope with other countries' characteristics and different levels of the spatial scale. Note to Practitioners - This article is motivated by the emerging need for developing effective methods to support policymakers in mitigating the effects of the COVID-19 pandemic. The proposed feedback control strategy - combining a multiregion epidemiological model with a nonlinear stochastic model predictive control approach - allows the robust identification of the most effective restrictive measures considering the corresponding effects on the healthcare and socioeconomic systems. The proposed framework is a general and flexible method that can be applied to various real scenarios, leveraging mobility data, available from the Google mobility service, to recognize patterns and predict future behaviors of individuals. © 2004-2012 IEEE.}, author_keywords = {COVID-19; epidemic control; mitigation strategies; pandemic modeling; stochastic model predictive control (MPC)}, keywords = {Decision making; Disease control; Model predictive control; Optimization; Predictive control systems; Random processes; Stochastic control systems; Stochastic models; COVID-19; Epidemic control; Medical services; Mitigation strategy; Pandemic; Pandemic modeling; Predictive models; Stochastic model predictive control .; Stochastic model predictive controls; Uncertainty; Stochastic systems}, type = {Article}, publication_stage = {Final}, source = {Scopus}, note = {Cited by: 22; All Open Access, Green Open Access} }
- Bozza, A., Askari, B., Cavone, G., Carli, R. & Dotoli, M. (2022) An Adaptive Model Predictive Control Approach for Position Tracking and Force Control of a Hydraulic Actuator IN IEEE International Conference on Automation Science and Engineering., 1029 – 1034. doi:10.1109/CASE49997.2022.9926645
[BibTeX] [Abstract] [Download PDF]This paper presents an Adaptive Model Predictive Control (AMPC) approach for the position tracking and force control of a hydraulic actuator (HA). Due to its nonlinear dynamics, the iterative linearization paradigm is employed to approximate the HA system by a linear time-varying model. Such a representation is used as the internal plant model of the predictive controller to effectively make predictions on the system state. The effectiveness of the proposed AMPC architecture is shown through numerical experiments addressing the control of a real HA on different scenarios. Finally, a comparative analysis on several values of sampling time, prediction and control horizon is carried out in order to investigate the effect of the parameters tuning on the performance of the closed-loop control system. © 2022 IEEE.
@CONFERENCE{Bozza20221029, author = {Bozza, Augusto and Askari, Bahman and Cavone, Graziana and Carli, Raffaele and Dotoli, Mariagrazia}, title = {An Adaptive Model Predictive Control Approach for Position Tracking and Force Control of a Hydraulic Actuator}, year = {2022}, journal = {IEEE International Conference on Automation Science and Engineering}, volume = {2022-August}, pages = {1029 – 1034}, doi = {10.1109/CASE49997.2022.9926645}, url = {https://www.scopus.com/inward/record.uri?eid=2-s2.0-85141675790&doi=10.1109%2fCASE49997.2022.9926645&partnerID=40&md5=8ea9bb1c05f9c04a733472aeef5755d1}, abstract = {This paper presents an Adaptive Model Predictive Control (AMPC) approach for the position tracking and force control of a hydraulic actuator (HA). Due to its nonlinear dynamics, the iterative linearization paradigm is employed to approximate the HA system by a linear time-varying model. Such a representation is used as the internal plant model of the predictive controller to effectively make predictions on the system state. The effectiveness of the proposed AMPC architecture is shown through numerical experiments addressing the control of a real HA on different scenarios. Finally, a comparative analysis on several values of sampling time, prediction and control horizon is carried out in order to investigate the effect of the parameters tuning on the performance of the closed-loop control system. © 2022 IEEE.}, author_keywords = {Adaptive MPC; Hydraulic Actuator; Model Linearization; Nonlinear Systems; Position and Force Tracking}, keywords = {Adaptive control systems; Closed loop control systems; Hydraulic actuators; Linearization; Predictive control systems; Actuator system; Adaptive model predictive control; Adaptive MPC; Force tracking; Iterative linearizations; Model linearization; Model-predictive control approach; Position and force tracking; Position tracking control; Position/force control; Model predictive control}, type = {Conference paper}, publication_stage = {Final}, source = {Scopus}, note = {Cited by: 3} }
- Scarabaggio, P., Grammatico, S., Carli, R. & Dotoli, M. (2022) Distributed Demand Side Management with Stochastic Wind Power Forecasting. IN IEEE Transactions on Control Systems Technology, 30.97 – 112. doi:10.1109/TCST.2021.3056751
[BibTeX] [Abstract] [Download PDF]In this article, we propose a distributed demand-side management (DSM) approach for smart grids taking into account uncertainty in wind power forecasting. The smart grid model comprehends traditional users as well as active users (prosumers). Through a rolling-horizon approach, prosumers participate in a DSM program, aiming at minimizing their cost in the presence of uncertain wind power generation by a game theory approach. We assume that each user selfishly formulates its grid optimization problem as a noncooperative game. The core challenge in this article is defining an approach to cope with the uncertainty in wind power availability. We tackle this issue from two different sides: by employing the expected value to define a deterministic counterpart for the problem and by adopting a stochastic approximated framework. In the latter case, we employ the sample average approximation (SAA) technique, whose results are based on a probability density function (PDF) for the wind speed forecasts. We improve the PDF by using historical wind speed data, and by employing a control index that takes into account the weather condition stability. Numerical simulations on a real data set show that the proposed stochastic strategy generates lower individual costs compared to the standard expected value approach. © 1993-2012 IEEE.
@ARTICLE{Scarabaggio202297, author = {Scarabaggio, Paolo and Grammatico, Sergio and Carli, Raffaele and Dotoli, Mariagrazia}, title = {Distributed Demand Side Management with Stochastic Wind Power Forecasting}, year = {2022}, journal = {IEEE Transactions on Control Systems Technology}, volume = {30}, number = {1}, pages = {97 – 112}, doi = {10.1109/TCST.2021.3056751}, url = {https://www.scopus.com/inward/record.uri?eid=2-s2.0-85100918524&doi=10.1109%2fTCST.2021.3056751&partnerID=40&md5=4f491e205e325ad467291e71ce8dbff8}, abstract = {In this article, we propose a distributed demand-side management (DSM) approach for smart grids taking into account uncertainty in wind power forecasting. The smart grid model comprehends traditional users as well as active users (prosumers). Through a rolling-horizon approach, prosumers participate in a DSM program, aiming at minimizing their cost in the presence of uncertain wind power generation by a game theory approach. We assume that each user selfishly formulates its grid optimization problem as a noncooperative game. The core challenge in this article is defining an approach to cope with the uncertainty in wind power availability. We tackle this issue from two different sides: by employing the expected value to define a deterministic counterpart for the problem and by adopting a stochastic approximated framework. In the latter case, we employ the sample average approximation (SAA) technique, whose results are based on a probability density function (PDF) for the wind speed forecasts. We improve the PDF by using historical wind speed data, and by employing a control index that takes into account the weather condition stability. Numerical simulations on a real data set show that the proposed stochastic strategy generates lower individual costs compared to the standard expected value approach. © 1993-2012 IEEE.}, author_keywords = {Demand-side management (DSM); model predictive control; sample average approximation (SAA); smart grid; stochastic optimization}, keywords = {Electric power generation; Electric power transmission networks; Electric utilities; Game theory; Probability density function; Smart power grids; Stochastic systems; Weather forecasting; Wind; Wind power; Grid optimization; Noncooperative game; Probability density function (pdf); Sample average approximation; Stochastic winds; Wind power availability; Wind power forecasting; Wind speed forecast; Demand side management}, type = {Article}, publication_stage = {Final}, source = {Scopus}, note = {Cited by: 88; All Open Access, Green Open Access} }
- Proia, S., Cavone, G., Camposeo, A., Ceglie, F., Carli, R. & Dotoli, M. (2022) Safe and Ergonomic Human-Drone Interaction in Warehouses IN IEEE International Conference on Intelligent Robots and Systems., 6681 – 6686. doi:10.1109/IROS47612.2022.9981469
[BibTeX] [Abstract] [Download PDF]This paper presents an application of human-drone interaction (HDI) for inventory management in a ware-house 4.0 that aims at improving the operators’ safety and well-being together with increasing efficiency and reducing production costs. In our work, the speed and separation monitoring (SSM) methodology is applied for the first time to HDI, in analogy to the human-robot interaction (HRI) ISO safety requirements as well as the rapid upper limb assessment (RULA), for evaluating the operator’s ergonomic posture during the interaction with the drone. With the aim of validating the proposed approach in a realistic scenario, a quadrotor is controlled to perform a pick and place task along a desired trajectory, from the picking bay to the palletizing area where the operator is located, avoiding collisions with the warehouse shelves by implementing the artificial potential field technique (APF) for planning and the linear quadratic regulator (LQR) and iterative LQR (iLQR) algorithms for tracking. The obtained results of the HDI architecture simulations are presented and discussed in detail proving the effectiveness of the proposed method for a safe and ergonomic HDI. © 2022 IEEE.
@CONFERENCE{Proia20226681, author = {Proia, Silvia and Cavone, Graziana and Camposeo, Antonio and Ceglie, Fabio and Carli, Raffaele and Dotoli, Mariagrazia}, title = {Safe and Ergonomic Human-Drone Interaction in Warehouses}, year = {2022}, journal = {IEEE International Conference on Intelligent Robots and Systems}, volume = {2022-October}, pages = {6681 – 6686}, doi = {10.1109/IROS47612.2022.9981469}, url = {https://www.scopus.com/inward/record.uri?eid=2-s2.0-85146319710&doi=10.1109%2fIROS47612.2022.9981469&partnerID=40&md5=61b20f9f8ff557ea77ff2ae551b6207b}, abstract = {This paper presents an application of human-drone interaction (HDI) for inventory management in a ware-house 4.0 that aims at improving the operators' safety and well-being together with increasing efficiency and reducing production costs. In our work, the speed and separation monitoring (SSM) methodology is applied for the first time to HDI, in analogy to the human-robot interaction (HRI) ISO safety requirements as well as the rapid upper limb assessment (RULA), for evaluating the operator's ergonomic posture during the interaction with the drone. With the aim of validating the proposed approach in a realistic scenario, a quadrotor is controlled to perform a pick and place task along a desired trajectory, from the picking bay to the palletizing area where the operator is located, avoiding collisions with the warehouse shelves by implementing the artificial potential field technique (APF) for planning and the linear quadratic regulator (LQR) and iterative LQR (iLQR) algorithms for tracking. The obtained results of the HDI architecture simulations are presented and discussed in detail proving the effectiveness of the proposed method for a safe and ergonomic HDI. © 2022 IEEE.}, author_keywords = {aerial robotics; ergonomics; Human-drone interaction; quadrotor; safety; speed and separation monitoring; trajectory planning; trajectory tracking}, keywords = {Antennas; Ergonomics; Human robot interaction; Inventory control; Iterative methods; Robot programming; Trajectories; Warehouses; Aerial robotics; Human-drone interaction; Inventory management; Operator safety; Production cost; Quad rotors; Speed and separation monitoring; Trajectory Planning; Trajectory-tracking; Well being; Drones}, type = {Conference paper}, publication_stage = {Final}, source = {Scopus}, note = {Cited by: 5} }
- Askari, B., Carli, R., Cavone, G. & Dotoli, M. (2022) Data-Driven Fault Diagnosis in a Complex Hydraulic System based on Early Classification IN IFAC-PapersOnLine., 187 – 192. doi:10.1016/j.ifacol.2023.01.070
[BibTeX] [Abstract] [Download PDF]In this paper, an early time-series classification (ETSC) algorithm is applied to support fault diagnosis in a complex hydraulic system (HS) with several interconnected components. The proposed technique aims at early classifying the state of the system while keeping the loss of classification inaccuracy at the minimum level. In contrast to baseline models that detect the eventual faults at the end of each working cycle, the ETSC model can diagnose any fault type of the HS components before observing the entire working cycle. Indeed, the early classification model successfully achieves a trade-off between the accuracy and the earliness criterion. Experimental results on a realistic HS dataset from the related literature show that the ETSC method can effectively identify different fault types with a higher accuracy and earlier compared to baseline methodologies. Copyright © 2022 The Authors. This is an open access article under the CC BY-NC-ND license (https://creativecommons.org/licenses/by-nc-nd/4.0/)
@CONFERENCE{Askari2022187, author = {Askari, Bahman and Carli, Raffaele and Cavone, Graziana and Dotoli, Mariagrazia}, title = {Data-Driven Fault Diagnosis in a Complex Hydraulic System based on Early Classification}, year = {2022}, journal = {IFAC-PapersOnLine}, volume = {55}, number = {40}, pages = {187 – 192}, doi = {10.1016/j.ifacol.2023.01.070}, url = {https://www.scopus.com/inward/record.uri?eid=2-s2.0-85159280640&doi=10.1016%2fj.ifacol.2023.01.070&partnerID=40&md5=ab3c6dd293b6af56ac23ff81957cc262}, abstract = {In this paper, an early time-series classification (ETSC) algorithm is applied to support fault diagnosis in a complex hydraulic system (HS) with several interconnected components. The proposed technique aims at early classifying the state of the system while keeping the loss of classification inaccuracy at the minimum level. In contrast to baseline models that detect the eventual faults at the end of each working cycle, the ETSC model can diagnose any fault type of the HS components before observing the entire working cycle. Indeed, the early classification model successfully achieves a trade-off between the accuracy and the earliness criterion. Experimental results on a realistic HS dataset from the related literature show that the ETSC method can effectively identify different fault types with a higher accuracy and earlier compared to baseline methodologies. Copyright © 2022 The Authors. This is an open access article under the CC BY-NC-ND license (https://creativecommons.org/licenses/by-nc-nd/4.0/)}, author_keywords = {Condition Based Monitoring; Early Classification; Fault Diagnosis; Hydraulic Systems; Machine Learning; Prognostics and Health Management; Time-series}, keywords = {Computer aided diagnosis; Economic and social effects; Failure analysis; Fault detection; Hydraulic equipment; Information management; Machine learning; Complex hydraulic system; Condition-based monitoring; Early classification; Faults diagnosis; Hydraulic system; Machine-learning; Prognostic and health management; Time series classifications; Times series; Working cycle; Time series}, type = {Conference paper}, publication_stage = {Final}, source = {Scopus}, note = {Cited by: 3; All Open Access, Gold Open Access} }
- Proia, S., Cavone, G., Carli, R. & Dotoli, M. (2022) A Multi-objective Optimization Approach for Trajectory Planning in a Safe and Ergonomic Human-Robot Collaboration IN IEEE International Conference on Automation Science and Engineering., 2068 – 2073. doi:10.1109/CASE49997.2022.9926513
[BibTeX] [Abstract] [Download PDF]In today’s manufacturing companies that rely on Human-Robot Collaboration (HRC), ensuring a safe and ergonomic workplace is becoming of pivotal importance. In a collaborative assembly scenario, this paper aims at planning the trajectory of a collaborative robotic arm, guaranteeing safety and ergonomics for the operator without neglecting production requirements. In particular, a multi-objective optimization approach for the trajectory planning in safe and ergonomic HRC is defined, with the aim of finding the best trade-off between the total traversal time of the trajectory for the robot and ergonomics for the human worker, while respecting safety requirements. The proposed approach consists of three main steps. First, the Rapid Upper Limb Assessment (RULA) ergonomic index is evaluated on a manikin designed on a dedicated software. The aim is to ensure a high quality of work in the considered HRC scenario with a consequent decrease of the musculoskeletal disorders associated with highly repetitive and dangerous activities. Second, a time-optimal and safety-constrained trajectory planning problem is defined as a second-order cone programming problem. Finally, a multi-objective control problem is formulated and solved to compute the trajectory that ensures the best compromise between time end ergonomics. The method is tested on numerical simulations and the obtained results are discussed, proving the effectiveness of the approach. © 2022 IEEE.
@CONFERENCE{Proia20222068, author = {Proia, Silvia and Cavone, Graziana and Carli, Raffaele and Dotoli, Mariagrazia}, title = {A Multi-objective Optimization Approach for Trajectory Planning in a Safe and Ergonomic Human-Robot Collaboration}, year = {2022}, journal = {IEEE International Conference on Automation Science and Engineering}, volume = {2022-August}, pages = {2068 – 2073}, doi = {10.1109/CASE49997.2022.9926513}, url = {https://www.scopus.com/inward/record.uri?eid=2-s2.0-85141688471&doi=10.1109%2fCASE49997.2022.9926513&partnerID=40&md5=2fd12a5e7dc0272a9ecfb95dcd756daf}, abstract = {In today's manufacturing companies that rely on Human-Robot Collaboration (HRC), ensuring a safe and ergonomic workplace is becoming of pivotal importance. In a collaborative assembly scenario, this paper aims at planning the trajectory of a collaborative robotic arm, guaranteeing safety and ergonomics for the operator without neglecting production requirements. In particular, a multi-objective optimization approach for the trajectory planning in safe and ergonomic HRC is defined, with the aim of finding the best trade-off between the total traversal time of the trajectory for the robot and ergonomics for the human worker, while respecting safety requirements. The proposed approach consists of three main steps. First, the Rapid Upper Limb Assessment (RULA) ergonomic index is evaluated on a manikin designed on a dedicated software. The aim is to ensure a high quality of work in the considered HRC scenario with a consequent decrease of the musculoskeletal disorders associated with highly repetitive and dangerous activities. Second, a time-optimal and safety-constrained trajectory planning problem is defined as a second-order cone programming problem. Finally, a multi-objective control problem is formulated and solved to compute the trajectory that ensures the best compromise between time end ergonomics. The method is tested on numerical simulations and the obtained results are discussed, proving the effectiveness of the approach. © 2022 IEEE.}, author_keywords = {cobots; collaborative robotics; ergonomics; Human-Robot Collaboration (HRC); safety; time-optimal trajectory planning}, keywords = {Collaborative robots; Economic and social effects; Multiobjective optimization; Numerical methods; Robot programming; Robotic assembly; Trajectories; Collaborative assembly; Ergonomic workplace; Human-robot collaboration; Manufacturing companies; Multi-objectives optimization; Optimization approach; Production requirements; Time-optimal trajectory planning; Trajectory Planning; Ergonomics}, type = {Conference paper}, publication_stage = {Final}, source = {Scopus}, note = {Cited by: 2} }
- Scarabaggio, P., Carli, R. & Dotoli, M. (2022) Noncooperative Equilibrium-Seeking in Distributed Energy Systems under AC Power Flow Nonlinear Constraints. IN IEEE Transactions on Control of Network Systems, 9.1731 – 1742. doi:10.1109/TCNS.2022.3181527
[BibTeX] [Abstract] [Download PDF]Power distribution grids are commonly controlled through centralized approaches, such as the optimal power flow. However, the current pervasive deployment of distributed renewable energy sources and the increasing growth of active players, providing ancillary services to the grid, have made these centralized frameworks no longer appropriate. In this context, we propose a novel noncooperative control mechanism for optimally regulating the operation of power distribution networks equipped with traditional loads, distributed generation, and active users. The latter, also known as prosumers, contribute to the grid optimization process by leveraging their flexible demand, dispatchable generation capability, and/or energy storage potential. Active users participate in a noncooperative liberalized market designed to increase the penetration of renewable generation and improve the predictability of power injection from the high-voltage grid. The novelty of our game-theoretical approach consists in incorporating economic factors as well as physical constraints and grid stability aspects. Finally, by integrating the proposed framework into a rolling-horizon approach, we show its effectiveness and resiliency through numerical experiments. © 2014 IEEE.
@ARTICLE{Scarabaggio20221731, author = {Scarabaggio, Paolo and Carli, Raffaele and Dotoli, Mariagrazia}, title = {Noncooperative Equilibrium-Seeking in Distributed Energy Systems under AC Power Flow Nonlinear Constraints}, year = {2022}, journal = {IEEE Transactions on Control of Network Systems}, volume = {9}, number = {4}, pages = {1731 – 1742}, doi = {10.1109/TCNS.2022.3181527}, url = {https://www.scopus.com/inward/record.uri?eid=2-s2.0-85132727510&doi=10.1109%2fTCNS.2022.3181527&partnerID=40&md5=01b1c79aa91772c7e9920d019e70b5b8}, abstract = {Power distribution grids are commonly controlled through centralized approaches, such as the optimal power flow. However, the current pervasive deployment of distributed renewable energy sources and the increasing growth of active players, providing ancillary services to the grid, have made these centralized frameworks no longer appropriate. In this context, we propose a novel noncooperative control mechanism for optimally regulating the operation of power distribution networks equipped with traditional loads, distributed generation, and active users. The latter, also known as prosumers, contribute to the grid optimization process by leveraging their flexible demand, dispatchable generation capability, and/or energy storage potential. Active users participate in a noncooperative liberalized market designed to increase the penetration of renewable generation and improve the predictability of power injection from the high-voltage grid. The novelty of our game-theoretical approach consists in incorporating economic factors as well as physical constraints and grid stability aspects. Finally, by integrating the proposed framework into a rolling-horizon approach, we show its effectiveness and resiliency through numerical experiments. © 2014 IEEE.}, author_keywords = {AC power flow; distributed control; electric power networks; game theory}, keywords = {Distributed parameter control systems; Distributed power generation; Electric load flow; Electric load management; Electric network analysis; Electric power system control; Electric power transmission networks; Natural resources; Renewable energy resources; AC power flow; Distributed energy systems; Distributed-control; Electric power networks; Generator; Load modeling; Network systems; Non-linear constraints; Power grids; Renewable energy source; Game theory}, type = {Article}, publication_stage = {Final}, source = {Scopus}, note = {Cited by: 34; All Open Access, Green Open Access, Hybrid Gold Open Access} }
- Tresca, G., Cavone, G., Carli, R., Cerviotti, A. & Dotoli, M. (2022) Automating Bin Packing: A Layer Building Matheuristics for Cost Effective Logistics. IN IEEE Transactions on Automation Science and Engineering, 19.1599 – 1613. doi:10.1109/TASE.2022.3177422
[BibTeX] [Abstract] [Download PDF]In this paper, we address the problem of automating the definition of feasible pallets configurations. This issue is crucial for the competitiveness of logistic companies and is still one of the most difficult problems in internal logistics. In fact, it requires the fast solution of a three-dimensional Bin Packing Problem (3D-BPP) with additional logistic specifications that are fundamental in real applications. To this aim, we propose a matheuristics that, given a set of items, provides feasible pallets configurations that satisfy the practical requirements of items’ grouping by logistic features, load bearing, stability, height homogeneity, overhang as well as weight limits, and robotized layer picking. The proposed matheuristics combines a mixed integer linear programming (MILP) formulation of the 3D-Single Bin-Size BPP (3D-SBSBPP) and a layer building heuristics. In particular, the feasible pallets configurations are obtained by sequentially solving two MILP sub-problems: the first, given the set of items to be packed, aims at minimizing the unused space in each layer and thus the number of layers; the latter aims at minimizing the number of shipping bins given the set of layers obtained from the first problem. The approach is extensively tested and compared with existing approaches. For its validation we use both realistic data-sets drawn from the literature and real data-sets, obtained from an Italian logistics leader. The resulting outcomes show the effectiveness of the method in providing high-quality bin configurations in short computational times. Note to Practitioners – This work is motivated by the intention of facilitating the transition from Logistics 3.0 to Logistics 4.0 by providing an effective tool to automate bin packing, suitable for automated warehouses. On the one hand, the proposed technique provides stable and compact bin configurations in less than half a minute per bin on average, despite the high computational complexity of the 3D-SBSBPP. On the other hand, the approach allows to consider compatibility constraints for the items (e.g., final customer and category of the items), and the use of robotized layer picking in automated warehouses. In effect, layers composed by only one type of items (i.e., monoitem layers) can be directly picked and placed on the pallet by a robotic arm without the intervention of any operator. Consequently, the adoption of this approach in warehouses could drastically improve the efficiency of the packing process. © 2004-2012 IEEE.
@ARTICLE{Tresca20221599, author = {Tresca, Giulia and Cavone, Graziana and Carli, Raffaele and Cerviotti, Antonio and Dotoli, Mariagrazia}, title = {Automating Bin Packing: A Layer Building Matheuristics for Cost Effective Logistics}, year = {2022}, journal = {IEEE Transactions on Automation Science and Engineering}, volume = {19}, number = {3}, pages = {1599 – 1613}, doi = {10.1109/TASE.2022.3177422}, url = {https://www.scopus.com/inward/record.uri?eid=2-s2.0-85131738365&doi=10.1109%2fTASE.2022.3177422&partnerID=40&md5=1c640184f7ba0ceda489906b68da49b9}, abstract = {In this paper, we address the problem of automating the definition of feasible pallets configurations. This issue is crucial for the competitiveness of logistic companies and is still one of the most difficult problems in internal logistics. In fact, it requires the fast solution of a three-dimensional Bin Packing Problem (3D-BPP) with additional logistic specifications that are fundamental in real applications. To this aim, we propose a matheuristics that, given a set of items, provides feasible pallets configurations that satisfy the practical requirements of items' grouping by logistic features, load bearing, stability, height homogeneity, overhang as well as weight limits, and robotized layer picking. The proposed matheuristics combines a mixed integer linear programming (MILP) formulation of the 3D-Single Bin-Size BPP (3D-SBSBPP) and a layer building heuristics. In particular, the feasible pallets configurations are obtained by sequentially solving two MILP sub-problems: the first, given the set of items to be packed, aims at minimizing the unused space in each layer and thus the number of layers; the latter aims at minimizing the number of shipping bins given the set of layers obtained from the first problem. The approach is extensively tested and compared with existing approaches. For its validation we use both realistic data-sets drawn from the literature and real data-sets, obtained from an Italian logistics leader. The resulting outcomes show the effectiveness of the method in providing high-quality bin configurations in short computational times. Note to Practitioners - This work is motivated by the intention of facilitating the transition from Logistics 3.0 to Logistics 4.0 by providing an effective tool to automate bin packing, suitable for automated warehouses. On the one hand, the proposed technique provides stable and compact bin configurations in less than half a minute per bin on average, despite the high computational complexity of the 3D-SBSBPP. On the other hand, the approach allows to consider compatibility constraints for the items (e.g., final customer and category of the items), and the use of robotized layer picking in automated warehouses. In effect, layers composed by only one type of items (i.e., monoitem layers) can be directly picked and placed on the pallet by a robotic arm without the intervention of any operator. Consequently, the adoption of this approach in warehouses could drastically improve the efficiency of the packing process. © 2004-2012 IEEE.}, author_keywords = {bin packing; Logistics 4.0; matheuristics; pallets configuration}, keywords = {Cost effectiveness; Integer programming; Logistics; Pallets; Bin packing; Cost-effective logistics; Fast solutions; Internal Logistics; Logistic 4.0; Logistics company; Matheuristic.; Mixed integer linear; Pallet configuration; Stability criterions; Stability criteria}, type = {Article}, publication_stage = {Final}, source = {Scopus}, note = {Cited by: 26; All Open Access, Hybrid Gold Open Access} }
- Carli, R., Cavone, G., Pippia, T., De Schutter, B. & Dotoli, M. (2022) Robust Optimal Control for Demand Side Management of Multi-Carrier Microgrids. IN IEEE Transactions on Automation Science and Engineering, 19.1338 – 1351. doi:10.1109/TASE.2022.3148856
[BibTeX] [Abstract] [Download PDF]This paper focuses on the control of microgrids where both gas and electricity are provided to the final customer, i.e., multi-carrier microgrids. Hence, these microgrids include thermal and electrical loads, renewable energy sources, energy storage systems, heat pumps, and combined heat and power units. The parameters characterizing the multi-carrier microgrid are subject to several disturbances, such as fluctuations in the provision of renewable energy, variability in the electrical and thermal demand, and uncertainties in the electricity and gas pricing. With the aim of accounting for the data uncertainties in the microgrid, we propose a Robust Model Predictive Control (RMPC) approach whose goal is to minimize the total economical cost, while satisfying comfort and energy requests of the final users. In the related literature various RMPC approaches have been proposed, focusing either on electrical or on thermal microgrids. Only a few contributions have addressed the robust control of multi-carrier microgrids. Consequently, we propose an innovative RMPC algorithm that employs on an uncertainty set-based method and that can provide better performance compared with deterministic model predictive controllers applied to multi-carrier microgrids. With the aim of mitigating the conservativeness of the approach, we define suitable robustness factors and we investigate the effects of such factors on the robustness of the solution against variations of the uncertain parameters. We show the effectiveness of the proposed RMPC approach by applying it to a realistic residential multi-carrier microgrid and comparing the obtained results with the ones of a baseline robust method. Note to Practitioners – This work is motivated by the emerging need for effective energy management approaches in multi-carrier microgrids. The inherent difficulty of scheduling simultaneously the operations of various energy infrastructures (e.g., electricity, natural gas) is exacerbated by the inevitable presence of uncertainties that affect the inter-dependent dynamics of different energy resources and equipment. The proposed robust MPC-based control strategy allows the energy manager to effectively determine an optimal energy scheduling of multi-faceted system components, making a tradeoff between performance and protection against data uncertainty. The presented strategy is comprehensive and generic, as it can be applied to different microgrid frameworks integrating various types of system components and sources of uncertainty, while at the same time being implementable in any energy management system. © 2004-2012 IEEE.
@ARTICLE{Carli20221338, author = {Carli, Raffaele and Cavone, Graziana and Pippia, Tomas and De Schutter, Bart and Dotoli, Mariagrazia}, title = {Robust Optimal Control for Demand Side Management of Multi-Carrier Microgrids}, year = {2022}, journal = {IEEE Transactions on Automation Science and Engineering}, volume = {19}, number = {3}, pages = {1338 – 1351}, doi = {10.1109/TASE.2022.3148856}, url = {https://www.scopus.com/inward/record.uri?eid=2-s2.0-85124835914&doi=10.1109%2fTASE.2022.3148856&partnerID=40&md5=bba929de55ddb0e8109670e2ef200715}, abstract = {This paper focuses on the control of microgrids where both gas and electricity are provided to the final customer, i.e., multi-carrier microgrids. Hence, these microgrids include thermal and electrical loads, renewable energy sources, energy storage systems, heat pumps, and combined heat and power units. The parameters characterizing the multi-carrier microgrid are subject to several disturbances, such as fluctuations in the provision of renewable energy, variability in the electrical and thermal demand, and uncertainties in the electricity and gas pricing. With the aim of accounting for the data uncertainties in the microgrid, we propose a Robust Model Predictive Control (RMPC) approach whose goal is to minimize the total economical cost, while satisfying comfort and energy requests of the final users. In the related literature various RMPC approaches have been proposed, focusing either on electrical or on thermal microgrids. Only a few contributions have addressed the robust control of multi-carrier microgrids. Consequently, we propose an innovative RMPC algorithm that employs on an uncertainty set-based method and that can provide better performance compared with deterministic model predictive controllers applied to multi-carrier microgrids. With the aim of mitigating the conservativeness of the approach, we define suitable robustness factors and we investigate the effects of such factors on the robustness of the solution against variations of the uncertain parameters. We show the effectiveness of the proposed RMPC approach by applying it to a realistic residential multi-carrier microgrid and comparing the obtained results with the ones of a baseline robust method. Note to Practitioners - This work is motivated by the emerging need for effective energy management approaches in multi-carrier microgrids. The inherent difficulty of scheduling simultaneously the operations of various energy infrastructures (e.g., electricity, natural gas) is exacerbated by the inevitable presence of uncertainties that affect the inter-dependent dynamics of different energy resources and equipment. The proposed robust MPC-based control strategy allows the energy manager to effectively determine an optimal energy scheduling of multi-faceted system components, making a tradeoff between performance and protection against data uncertainty. The presented strategy is comprehensive and generic, as it can be applied to different microgrid frameworks integrating various types of system components and sources of uncertainty, while at the same time being implementable in any energy management system. © 2004-2012 IEEE.}, author_keywords = {demand side management (DSM); Energy and environment-aware automation; multi-carrier microgrid; robust model predictive control; robust optimization; set-based uncertainty}, keywords = {Demand side management; Digital storage; Electric utilities; Heat pump systems; Heat resistance; Heat storage; Model predictive control; Natural resources; Optimization; Random processes; Renewable energy resources; Stochastic systems; Uncertainty analysis; Demand side management; Energy and environment; Energy and environment-aware automation; Heat pumps; Microgrid; Multi-carrier microgrid; Multicarriers; Renewable energy source; Resistance-heating; Robust model predictive control; Robust model predictive control.; Robust optimization; Robustness; Set-based uncertainty; Uncertainty; Robust control}, type = {Article}, publication_stage = {Final}, source = {Scopus}, note = {Cited by: 59; All Open Access, Green Open Access} }
- Cavone, G., Bozza, A., Carli, R. & Dotoli, M. (2022) MPC-Based Process Control of Deep Drawing: An Industry 4.0 Case Study in Automotive. IN IEEE Transactions on Automation Science and Engineering, 19.1586 – 1598. doi:10.1109/TASE.2022.3177362
[BibTeX] [Abstract] [Download PDF]Deep drawing is a metalworking procedure aimed at getting a cold metal sheet plastically deformed in accordance with a pre-defined mould. Although this procedure is well-established in industry, it is still susceptible to several issues affecting the quality of the stamped metal products. In order to reduce defects of workpieces, process control approaches can be performed. Typically, process control employs simple proportional-integral-derivative (PID) regulators that steer the blank holder force (BHF) based on the error on the punch force. However, a single PID can only control single-input single-output systems and cannot handle constraints on the process variables. Differently from the state of the art, in this paper we propose a process control architecture based on Model Predictive Control (MPC), which considers a multi-variable system model. In particular, we represent the deep drawing process with a single-input multiple-output Hammerstein-Wiener model that relates the BHF with the draw-in of $n$ different critical points around the die. This allows the avoidance of workpiece defects that are due to the abnormal sliding of the metal sheet during the forming phase. The effectiveness of the proposed process controller is shown on a real case study in a digital twin framework, where the performance achieved by the MPC-based system is analyzed in detail and compared against the results obtained through an ad-hoc defined multiple PID-based control architecture. Note to Practitioners – This work is motivated by the emerging need for the effective implementation of the zero-defect manufacturing paradigm in the Industry 4.0 framework. Especially in the deep drawing process, various quality issues in stamped parts can lead to significant product waste and manufacturing inefficiencies. This turns into considerable economic losses for companies, particularly in the automotive sector, where deep drawing is one of the most used cold sheet metal forming techniques. In most applications, only sample inspections are performed on batches of finished-product, with subsequent losses of time and resources. For the sake of improving the workpiece quality, innovative strategies for real-time process control represent a viable and promising solution. In this context, the proposed MPC-based process control approach allows the correct shaping of the metal sheet that is getting deformed during the forming stroke, thanks to the draw-in monitoring at various locations around the die. The draw-in is indeed one of the most effective forming variables to control in order to provide a correct BHF during the forming stroke. A useful and easy-to-implement non-linear metal sheet deep drawing process model is provided by this paper to perform an innovative process control strategy. A comprehensive methodology is applied in detail to an automotive case study, ranging from process modeling (model identification and validation based on experimental data acquisition) to MPC implementation (controller tuning and testing and software-in-the-loop system validation). The presented method can be easily implemented on any real deep drawing press, providing the multivariable constrained process with a suitable control system able to make the stamped parts well formed. © 2004-2012 IEEE.
@ARTICLE{Cavone20221586, author = {Cavone, Graziana and Bozza, Augusto and Carli, Raffaele and Dotoli, Mariagrazia}, title = {MPC-Based Process Control of Deep Drawing: An Industry 4.0 Case Study in Automotive}, year = {2022}, journal = {IEEE Transactions on Automation Science and Engineering}, volume = {19}, number = {3}, pages = {1586 – 1598}, doi = {10.1109/TASE.2022.3177362}, url = {https://www.scopus.com/inward/record.uri?eid=2-s2.0-85131727980&doi=10.1109%2fTASE.2022.3177362&partnerID=40&md5=689e5dd375cab07673634f266a278717}, abstract = {Deep drawing is a metalworking procedure aimed at getting a cold metal sheet plastically deformed in accordance with a pre-defined mould. Although this procedure is well-established in industry, it is still susceptible to several issues affecting the quality of the stamped metal products. In order to reduce defects of workpieces, process control approaches can be performed. Typically, process control employs simple proportional-integral-derivative (PID) regulators that steer the blank holder force (BHF) based on the error on the punch force. However, a single PID can only control single-input single-output systems and cannot handle constraints on the process variables. Differently from the state of the art, in this paper we propose a process control architecture based on Model Predictive Control (MPC), which considers a multi-variable system model. In particular, we represent the deep drawing process with a single-input multiple-output Hammerstein-Wiener model that relates the BHF with the draw-in of $n$ different critical points around the die. This allows the avoidance of workpiece defects that are due to the abnormal sliding of the metal sheet during the forming phase. The effectiveness of the proposed process controller is shown on a real case study in a digital twin framework, where the performance achieved by the MPC-based system is analyzed in detail and compared against the results obtained through an ad-hoc defined multiple PID-based control architecture. Note to Practitioners - This work is motivated by the emerging need for the effective implementation of the zero-defect manufacturing paradigm in the Industry 4.0 framework. Especially in the deep drawing process, various quality issues in stamped parts can lead to significant product waste and manufacturing inefficiencies. This turns into considerable economic losses for companies, particularly in the automotive sector, where deep drawing is one of the most used cold sheet metal forming techniques. In most applications, only sample inspections are performed on batches of finished-product, with subsequent losses of time and resources. For the sake of improving the workpiece quality, innovative strategies for real-time process control represent a viable and promising solution. In this context, the proposed MPC-based process control approach allows the correct shaping of the metal sheet that is getting deformed during the forming stroke, thanks to the draw-in monitoring at various locations around the die. The draw-in is indeed one of the most effective forming variables to control in order to provide a correct BHF during the forming stroke. A useful and easy-to-implement non-linear metal sheet deep drawing process model is provided by this paper to perform an innovative process control strategy. A comprehensive methodology is applied in detail to an automotive case study, ranging from process modeling (model identification and validation based on experimental data acquisition) to MPC implementation (controller tuning and testing and software-in-the-loop system validation). The presented method can be easily implemented on any real deep drawing press, providing the multivariable constrained process with a suitable control system able to make the stamped parts well formed. © 2004-2012 IEEE.}, author_keywords = {Metal deep drawing process control; model predictive control; real-time process control; software-in-the-loop simulation; zero-defect manufacturing}, keywords = {Computer software; Defects; Metal drawing; Metals; Predictive control systems; Proportional control systems; Sheet metal; Two term control systems; Deep-drawing process; Force; Metal deep drawing process control; Model-predictive control; Real-time process control; Software-in-the-loop simulation.; Software-in-the-loop simulations; Uncertainty; Zero defects; Zero-defect manufacturing; Model predictive control}, type = {Article}, publication_stage = {Final}, source = {Scopus}, note = {Cited by: 19; All Open Access, Hybrid Gold Open Access} }
- Cavone, G., Carli, R. & Dotoli, M. (2022) Decision and Control Approaches for Enhancing the Resilience of Distribution Networks: a Survey IN IFAC-PapersOnLine., 271 – 276. doi:10.1016/j.ifacol.2023.01.084
[BibTeX] [Abstract] [Download PDF]Recently, the concept of resilience of electrical infrastructures has been introduced to quantify the ability of the grid to resist, adapt to, and rapidly recover after the occurrence of high-impact and low-probability (HILP) events. Various surveys discuss the state of the art on the resilience of distribution networks (DNs), which are a subsystem of the electrical infrastructure particularly susceptible to HILP events. It emerges that automation has a central role in guaranteeing and enhancing DNs resilience, although a classification of the existing contributions is missing. To fill this gap, in this paper we review the literature contributions regarding decision and control methods to enhance the resilience of DNs. We classify the reviewed approaches into tactical/strategic and operational level ones, we group them by the time of application and type, and finally we provide a detailed discussion and comparison of the available methods, highlighting open issues and future trends in this field. Copyright © 2022 The Authors. This is an open access article under the CC BY-NC-ND license (https://creativecommons.org/licenses/by-nc-nd/4.0/)
@CONFERENCE{Cavone2022271, author = {Cavone, Graziana and Carli, Raffaele and Dotoli, Mariagrazia}, title = {Decision and Control Approaches for Enhancing the Resilience of Distribution Networks: a Survey}, year = {2022}, journal = {IFAC-PapersOnLine}, volume = {55}, number = {40}, pages = {271 – 276}, doi = {10.1016/j.ifacol.2023.01.084}, url = {https://www.scopus.com/inward/record.uri?eid=2-s2.0-85159328754&doi=10.1016%2fj.ifacol.2023.01.084&partnerID=40&md5=daaa34f2c14722ee40039f3d2c434daf}, abstract = {Recently, the concept of resilience of electrical infrastructures has been introduced to quantify the ability of the grid to resist, adapt to, and rapidly recover after the occurrence of high-impact and low-probability (HILP) events. Various surveys discuss the state of the art on the resilience of distribution networks (DNs), which are a subsystem of the electrical infrastructure particularly susceptible to HILP events. It emerges that automation has a central role in guaranteeing and enhancing DNs resilience, although a classification of the existing contributions is missing. To fill this gap, in this paper we review the literature contributions regarding decision and control methods to enhance the resilience of DNs. We classify the reviewed approaches into tactical/strategic and operational level ones, we group them by the time of application and type, and finally we provide a detailed discussion and comparison of the available methods, highlighting open issues and future trends in this field. Copyright © 2022 The Authors. This is an open access article under the CC BY-NC-ND license (https://creativecommons.org/licenses/by-nc-nd/4.0/)}, author_keywords = {control; decision; Electrical distribution networks; high-impact low-probability events; resilience}, keywords = {Internet protocols; Control approach; Decision; Electrical distribution networks; Electrical infrastructure; High impact; High impact/low probabilities; High-impact low-probability event; High-low; Lower probabilities; Resilience; Probability distributions}, type = {Conference paper}, publication_stage = {Final}, source = {Scopus}, note = {Cited by: 0; All Open Access, Gold Open Access} }
- Mignoni, N., Scarabaggio, P., Carli, R. & Dotoli, M. (2022) Game Theoretical Control Frameworks for Multiple Energy Storage Services in Energy Communities IN 2022 8th International Conference on Control, Decision and Information Technologies, CoDIT 2022., 1580 – 1585. doi:10.1109/CoDIT55151.2022.9804087
[BibTeX] [Abstract] [Download PDF]In the last decade, distributed energy generation and storage have significantly contributed to the widespread of energy communities. In this context, we propose an energy community model constituted by prosumers, characterized by their own demand and renewable generation, and service-oriented energy storage providers, able to store energy surplus and release it upon a fee payment. We address the problem of optimally schedule the energy flows in the community, with the final goal of making the prosumers’ energy supply more efficient, while creating a sustainable and profitable business model for storage providers. The proposed resolution algorithms are based on decentralized and distributed game theoretical control schemes. These approaches are mathematically formulated and then effectively validated and compared with a centralized method through numerical simulations on realistic scenarios. © 2022 IEEE.
@CONFERENCE{Mignoni20221580, author = {Mignoni, Nicola and Scarabaggio, Paolo and Carli, Raffaele and Dotoli, Mariagrazia}, title = {Game Theoretical Control Frameworks for Multiple Energy Storage Services in Energy Communities}, year = {2022}, journal = {2022 8th International Conference on Control, Decision and Information Technologies, CoDIT 2022}, pages = {1580 – 1585}, doi = {10.1109/CoDIT55151.2022.9804087}, url = {https://www.scopus.com/inward/record.uri?eid=2-s2.0-85134303591&doi=10.1109%2fCoDIT55151.2022.9804087&partnerID=40&md5=c5c686570c9998d9639b765af7b24245}, abstract = {In the last decade, distributed energy generation and storage have significantly contributed to the widespread of energy communities. In this context, we propose an energy community model constituted by prosumers, characterized by their own demand and renewable generation, and service-oriented energy storage providers, able to store energy surplus and release it upon a fee payment. We address the problem of optimally schedule the energy flows in the community, with the final goal of making the prosumers' energy supply more efficient, while creating a sustainable and profitable business model for storage providers. The proposed resolution algorithms are based on decentralized and distributed game theoretical control schemes. These approaches are mathematically formulated and then effectively validated and compared with a centralized method through numerical simulations on realistic scenarios. © 2022 IEEE.}, keywords = {Game theory; Numerical methods; Community model; Control framework; Demand generation; Distributed energy generation and storages; Energy; Energy flow; Energy supplies; Renewable generation; Service Oriented; Storage services; Energy storage}, type = {Conference paper}, publication_stage = {Final}, source = {Scopus}, note = {Cited by: 1} }
- Hosseini, S. M., Carli, R. & Dotoli, M. (2022) Robust Optimal Demand Response of Energy-efficient Commercial Buildings IN 2022 European Control Conference, ECC 2022., 1606 – 1609. doi:10.23919/ECC55457.2022.9837962
[BibTeX] [Abstract] [Download PDF]Commercial buildings show a great potential for participating in demand response (DR) programs due to their extensive use of energy-intensive flexible loads such as heating, ventilation, and air conditioning (HVAC) systems. The capability of HVAC systems for responding to automated control and intelligent energy scheduling strategies makes them essential flexibility sources in commercial DR. This capability, in combination with the use of local energy storage systems (ESSs), can substantially enhance the energy management performance. This paper proposes a novel robust model predictive control (MPC) approach for online energy scheduling of multiple commercial buildings comprising individual HVAC systems, ESSs, and non-controllable loads. The proposed approach aims at minimizing the total expected energy costs while ensuring the occupants’ thermal comfort under the presence of uncertainties in electricity market pricing. Moreover, operational constraints of the power grid and buildings’ components are considered. To this aim, we firstly formulate the energy scheduling problem as a min-max robust optimization problem which is transformed into a mixed-integer linear programming problem using duality. Next, we apply MPC to solve the robust optimization problem iteratively based on the receding horizon concept. Finally, we assess the performance of the proposed approach on a simulated realistic case study. © 2022 EUCA.
@CONFERENCE{Hosseini20221606, author = {Hosseini, Seyed Mohsen and Carli, Raffaele and Dotoli, Mariagrazia}, title = {Robust Optimal Demand Response of Energy-efficient Commercial Buildings}, year = {2022}, journal = {2022 European Control Conference, ECC 2022}, pages = {1606 – 1609}, doi = {10.23919/ECC55457.2022.9837962}, url = {https://www.scopus.com/inward/record.uri?eid=2-s2.0-85136689124&doi=10.23919%2fECC55457.2022.9837962&partnerID=40&md5=b113d8da8b5520d7ef6badc038c033df}, abstract = {Commercial buildings show a great potential for participating in demand response (DR) programs due to their extensive use of energy-intensive flexible loads such as heating, ventilation, and air conditioning (HVAC) systems. The capability of HVAC systems for responding to automated control and intelligent energy scheduling strategies makes them essential flexibility sources in commercial DR. This capability, in combination with the use of local energy storage systems (ESSs), can substantially enhance the energy management performance. This paper proposes a novel robust model predictive control (MPC) approach for online energy scheduling of multiple commercial buildings comprising individual HVAC systems, ESSs, and non-controllable loads. The proposed approach aims at minimizing the total expected energy costs while ensuring the occupants' thermal comfort under the presence of uncertainties in electricity market pricing. Moreover, operational constraints of the power grid and buildings' components are considered. To this aim, we firstly formulate the energy scheduling problem as a min-max robust optimization problem which is transformed into a mixed-integer linear programming problem using duality. Next, we apply MPC to solve the robust optimization problem iteratively based on the receding horizon concept. Finally, we assess the performance of the proposed approach on a simulated realistic case study. © 2022 EUCA.}, keywords = {Air conditioning; Costs; Electric energy storage; Electric power transmission networks; Energy efficiency; Model predictive control; Office buildings; Online systems; Optimal control systems; Predictive control systems; Robust control; Scheduling; Commercial building; Conditioning systems; Demand response; Energy; Heating ventilation and air conditioning; Optimization problems; Performance; Response of energy; Robust optimization; Storage systems; Integer programming}, type = {Conference paper}, publication_stage = {Final}, source = {Scopus}, note = {Cited by: 11} }
- Nasiri, F., Ooka, R., Haghighat, F., Shirzadi, N., Dotoli, M., Carli, R., Scarabaggio, P., Behzadi, A., Rahnama, S., Afshari, A., Kuznik, F., Fabrizio, E., Choudhary, R. & Sadrizadeh, S. (2022) Data Analytics and Information Technologies for Smart Energy Storage Systems: A State-of-the-Art Review. IN Sustainable Cities and Society, 84.. doi:10.1016/j.scs.2022.104004
[BibTeX] [Abstract] [Download PDF]This article provides a state-of-the-art review on emerging applications of smart tools such as data analytics and smart technologies such as internet-of-things in case of design, management and control of energy storage systems. In particular, we have established a classification of the types and targets of various predictive analytics for estimation of load, energy prices, renewable energy inputs, state of the charge, fault diagnosis, etc. In addition, the applications of information technologies, and in particular, use of cloud, internet-of-things, building management systems and building information modeling and their contributions to management of energy storage systems will be reviewed in details. The paper concludes by highlighting the emerging issues in smart energy storage systems and providing directions for future research. © 2022 Elsevier Ltd
@ARTICLE{Nasiri2022, author = {Nasiri, Fuzhan and Ooka, Ryozo and Haghighat, Fariborz and Shirzadi, Navid and Dotoli, Mariagrazia and Carli, Raffaele and Scarabaggio, Paolo and Behzadi, Amirmohammad and Rahnama, Samira and Afshari, Alireza and Kuznik, Frédéric and Fabrizio, Enrico and Choudhary, Ruchi and Sadrizadeh, Sasan}, title = {Data Analytics and Information Technologies for Smart Energy Storage Systems: A State-of-the-Art Review}, year = {2022}, journal = {Sustainable Cities and Society}, volume = {84}, doi = {10.1016/j.scs.2022.104004}, url = {https://www.scopus.com/inward/record.uri?eid=2-s2.0-85133235991&doi=10.1016%2fj.scs.2022.104004&partnerID=40&md5=c1409a9f5cd360d29ca6e0f1bf7f9fe9}, abstract = {This article provides a state-of-the-art review on emerging applications of smart tools such as data analytics and smart technologies such as internet-of-things in case of design, management and control of energy storage systems. In particular, we have established a classification of the types and targets of various predictive analytics for estimation of load, energy prices, renewable energy inputs, state of the charge, fault diagnosis, etc. In addition, the applications of information technologies, and in particular, use of cloud, internet-of-things, building management systems and building information modeling and their contributions to management of energy storage systems will be reviewed in details. The paper concludes by highlighting the emerging issues in smart energy storage systems and providing directions for future research. © 2022 Elsevier Ltd}, author_keywords = {Artificial Intelligence; Data Analytics; Energy Storage; Information Technology; Renewable Energy Intermittency; Smart Systems}, keywords = {Classification (of information); Digital storage; Energy storage; Information management; Internet of things; Predictive analytics; Data analytics; Data informations; Emerging applications; Intermittency; Renewable energies; Renewable energy intermittency; Smart energies; Smart System; State-of-the art reviews; Storage systems; alternative energy; artificial intelligence; data processing; design; energy storage; information technology; Data Analytics}, type = {Article}, publication_stage = {Final}, source = {Scopus}, note = {Cited by: 12} }
2021
- Cavone, G., Epicoco, N., Carli, R., Del Zotti, A., Paulo Ribeiro Pereira, J. & Dotoli, M. (2021) Parcel delivery with drones: Multi-criteria analysis of trendy system architectures IN 2021 29th Mediterranean Conference on Control and Automation, MED 2021., 693 – 698. doi:10.1109/MED51440.2021.9480332
[BibTeX] [Abstract] [Download PDF]New technologies, such as Unmanned Aerial Vehicles (UAVs), are transforming facilities and vehicles into intelligent systems that will significantly modify logistic deliveries in any organization. With the appearance of automated vehicles, drones offer multiple new technological solutions that might trigger different delivery networks or boost new delivery services. Differently from the related works, where a single specific delivery system model is typically addressed, this paper deals with the use of UAVs for logistic deliveries focusing on a multi-criteria analysis of trendy drone-based system architectures. In particular, using the cross-efficiency Data Envelopment Analysis approach, a comparative analysis among three different delivery systems is performed: the classic system based on trucks only, the drone-only system using a fleet of drones, and the hybrid truck and drone system combining trucks and drones. The proposed technique constitutes an effective decision-making tool aimed at helping delivery companies in selecting the optimal delivery system architecture according to their specific needs. The effectiveness of the proposed methodology is shown by a simulation analysis based on a realistic data case study that pertains to the main logistic service providers. © 2021 IEEE.
@CONFERENCE{Cavone2021693, author = {Cavone, Graziana and Epicoco, Nicola and Carli, Raffaele and Del Zotti, Anna and Paulo Ribeiro Pereira, Joao and Dotoli, Mariagrazia}, title = {Parcel delivery with drones: Multi-criteria analysis of trendy system architectures}, year = {2021}, journal = {2021 29th Mediterranean Conference on Control and Automation, MED 2021}, pages = {693 – 698}, doi = {10.1109/MED51440.2021.9480332}, url = {https://www.scopus.com/inward/record.uri?eid=2-s2.0-85113688309&doi=10.1109%2fMED51440.2021.9480332&partnerID=40&md5=b1147e916948e156664d7ff61520c1dd}, abstract = {New technologies, such as Unmanned Aerial Vehicles (UAVs), are transforming facilities and vehicles into intelligent systems that will significantly modify logistic deliveries in any organization. With the appearance of automated vehicles, drones offer multiple new technological solutions that might trigger different delivery networks or boost new delivery services. Differently from the related works, where a single specific delivery system model is typically addressed, this paper deals with the use of UAVs for logistic deliveries focusing on a multi-criteria analysis of trendy drone-based system architectures. In particular, using the cross-efficiency Data Envelopment Analysis approach, a comparative analysis among three different delivery systems is performed: the classic system based on trucks only, the drone-only system using a fleet of drones, and the hybrid truck and drone system combining trucks and drones. The proposed technique constitutes an effective decision-making tool aimed at helping delivery companies in selecting the optimal delivery system architecture according to their specific needs. The effectiveness of the proposed methodology is shown by a simulation analysis based on a realistic data case study that pertains to the main logistic service providers. © 2021 IEEE.}, author_keywords = {Data Envelopment Analysis; Drones; Multi-criteria decision making; Parcel delivery; UAVs}, keywords = {Antennas; Automation; Automobiles; Data envelopment analysis; Decision making; Drones; Fleet operations; Intelligent systems; Network architecture; Trucks; Automated vehicles; Comparative analysis; Decision making tool; Logistic services; Multi Criteria Analysis; Simulation analysis; System architectures; Technological solution; Computer architecture}, type = {Conference paper}, publication_stage = {Final}, source = {Scopus}, note = {Cited by: 12; All Open Access, Green Open Access} }
- Cavone, G., Carli, R., Troccoli, G., Tresca, G. & Dotoli, M. (2021) A MILP approach for the multi-drop container loading problem resolution in logistics 4.0 IN 2021 29th Mediterranean Conference on Control and Automation, MED 2021., 687 – 692. doi:10.1109/MED51440.2021.9480359
[BibTeX] [Abstract] [Download PDF]This paper addresses the multi-drop container loading problem (CLP), i.e., the problem of packing multiple bins -associated to multiple deliveries to one or more customers- into a finite number of transport units (TUs). Differently from the traditional CLP, the multi-drop CLP has been rarely handled in the literature, while effective algorithms to automatically solve this problem are needed to improve the efficiency and sustainability of internal logistics. To this aim, we propose a novel algorithm that solves a delivery-based mixed integer linear programming formulation of the problem. The algorithm efficiently determines the optimal composition of TUs by minimizing the unused space, while fulfilling a set of geometric and safety constraints, and complying with the delivery allocation. In particular, the proposed algorithm includes two steps: the first aims at clustering bins into groups to be compatibly loaded in various TUs; the latter aims at determining the optimal configuration of each group in the related TU. Finally, the proposed algorithm is applied to several realistic case studies with the aim of testing and analysing its effectiveness in producing stable and compact TU loading configurations in a short computation time, despite the high computational complexity of the multi-drop CLP. © 2021 IEEE.
@CONFERENCE{Cavone2021687, author = {Cavone, Graziana and Carli, Raffaele and Troccoli, Giorgio and Tresca, Giulia and Dotoli, Mariagrazia}, title = {A MILP approach for the multi-drop container loading problem resolution in logistics 4.0}, year = {2021}, journal = {2021 29th Mediterranean Conference on Control and Automation, MED 2021}, pages = {687 – 692}, doi = {10.1109/MED51440.2021.9480359}, url = {https://www.scopus.com/inward/record.uri?eid=2-s2.0-85113699292&doi=10.1109%2fMED51440.2021.9480359&partnerID=40&md5=734929101ce82724d3629ddb1e06f4a4}, abstract = {This paper addresses the multi-drop container loading problem (CLP), i.e., the problem of packing multiple bins -associated to multiple deliveries to one or more customers- into a finite number of transport units (TUs). Differently from the traditional CLP, the multi-drop CLP has been rarely handled in the literature, while effective algorithms to automatically solve this problem are needed to improve the efficiency and sustainability of internal logistics. To this aim, we propose a novel algorithm that solves a delivery-based mixed integer linear programming formulation of the problem. The algorithm efficiently determines the optimal composition of TUs by minimizing the unused space, while fulfilling a set of geometric and safety constraints, and complying with the delivery allocation. In particular, the proposed algorithm includes two steps: the first aims at clustering bins into groups to be compatibly loaded in various TUs; the latter aims at determining the optimal configuration of each group in the related TU. Finally, the proposed algorithm is applied to several realistic case studies with the aim of testing and analysing its effectiveness in producing stable and compact TU loading configurations in a short computation time, despite the high computational complexity of the multi-drop CLP. © 2021 IEEE.}, author_keywords = {Container loading problem; Logistics; MILP; Multi-drop; Optimization}, keywords = {Bins; Drops; Integer programming; Container-loading problems; Effective algorithms; Internal Logistics; Loading configuration; Mixed integer linear programming; Multiple deliveries; Optimal composition; Safety constraint; Clustering algorithms}, type = {Conference paper}, publication_stage = {Final}, source = {Scopus}, note = {Cited by: 5} }
- Atrigna, M., Buonanno, A., Carli, R., Cavone, G., Scarabaggio, P., Valenti, M., Graditi, G. & Dotoli, M. (2021) Effects of Heatwaves on the Failure of Power Distribution Grids: A Fault Prediction System Based on Machine Learning IN 21st IEEE International Conference on Environment and Electrical Engineering and 2021 5th IEEE Industrial and Commercial Power System Europe, EEEIC / I and CPS Europe 2021 – Proceedings.. doi:10.1109/EEEIC/ICPSEurope51590.2021.9584751
[BibTeX] [Abstract] [Download PDF]Nowadays, a power system failure can drastically affect the reliability and normal operation of power distribution grids. The preparation for these failure events is currently approached with post-event analysis to identify the area of the system that requires the most resources in order to prevent future failures. Nevertheless, the forecasting of such events can be useful to anticipate the failure and possibly avoid it. In this work, we employ several machine learning approaches to analyze historical failure data and predict power grid outages based on operational and meteorological data. The approach is tested with real failure data of a power distribution network in the South of Italy, demonstrating advantageous results also to determine areas requiring particular attention. © 2021 IEEE
@CONFERENCE{Atrigna2021, author = {Atrigna, Mauro and Buonanno, Amedeo and Carli, Raffaele and Cavone, Graziana and Scarabaggio, Paolo and Valenti, Maria and Graditi, Giorgio and Dotoli, Mariagrazia}, title = {Effects of Heatwaves on the Failure of Power Distribution Grids: A Fault Prediction System Based on Machine Learning}, year = {2021}, journal = {21st IEEE International Conference on Environment and Electrical Engineering and 2021 5th IEEE Industrial and Commercial Power System Europe, EEEIC / I and CPS Europe 2021 - Proceedings}, doi = {10.1109/EEEIC/ICPSEurope51590.2021.9584751}, url = {https://www.scopus.com/inward/record.uri?eid=2-s2.0-85126456706&doi=10.1109%2fEEEIC%2fICPSEurope51590.2021.9584751&partnerID=40&md5=fc9788f00e259fdaabf0cb50c06253be}, abstract = {Nowadays, a power system failure can drastically affect the reliability and normal operation of power distribution grids. The preparation for these failure events is currently approached with post-event analysis to identify the area of the system that requires the most resources in order to prevent future failures. Nevertheless, the forecasting of such events can be useful to anticipate the failure and possibly avoid it. In this work, we employ several machine learning approaches to analyze historical failure data and predict power grid outages based on operational and meteorological data. The approach is tested with real failure data of a power distribution network in the South of Italy, demonstrating advantageous results also to determine areas requiring particular attention. © 2021 IEEE}, author_keywords = {machine learning; Power system failures; Power system reliability}, keywords = {Electric power transmission networks; Failure (mechanical); Forecasting; Machine learning; Meteorology; Systems engineering; Failure data; Fault prediction; Heatwaves; Machine-learning; Normal operations; On-machines; Power distribution grids; Power system failures; Power systems reliability; Prediction systems; Outages}, type = {Conference paper}, publication_stage = {Final}, source = {Scopus}, note = {Cited by: 4} }
- Calefati, M., Proia, S., Scarabaggio, P., Carli, R. & Dotoli, M. (2021) A Decentralized Noncooperative Control Approach for Sharing Energy Storage Systems in Energy Communities IN Conference Proceedings – IEEE International Conference on Systems, Man and Cybernetics., 1430 – 1435. doi:10.1109/SMC52423.2021.9658851
[BibTeX] [Abstract] [Download PDF]This paper focuses on the optimal scheduling of the charging and discharging strategies of a community energy storage (CES) system, which is shared by the prosumers belonging to a grid-connected energy community. The prosumers own renewable energy sources (RESs), while they can buy/sell their energy imbalance directly from/to the power grid. For the sake of increasing the penetration of RESs and reducing the operating cost, prosumers leverage on the shared CES: in particular, each user can only employ a portion of the overall CES charge/discharge profile. Differently from the related literature, where storage devices are individually owned and the battery degradation aspects are typically disregarded, we propose a novel control mechanism based on noncooperative game theory, which allows users to minimize their energy cost as well as concur on the CES resources allocation with minimal-degradation. The effectiveness of the method is validated through numerical experiments on a realistic case study, where a shared CES supplies energy to the local community of residential prosumers. Finally, the comparison with a centralized control approach shows that the proposed framework allows all prosumers to achieve a fair cost-optimal utilization of the shared CES. © 2021 IEEE.
@CONFERENCE{Calefati20211430, author = {Calefati, Marino and Proia, Silvia and Scarabaggio, Paolo and Carli, Raffaele and Dotoli, Mariagrazia}, title = {A Decentralized Noncooperative Control Approach for Sharing Energy Storage Systems in Energy Communities}, year = {2021}, journal = {Conference Proceedings - IEEE International Conference on Systems, Man and Cybernetics}, pages = {1430 – 1435}, doi = {10.1109/SMC52423.2021.9658851}, url = {https://www.scopus.com/inward/record.uri?eid=2-s2.0-85124302216&doi=10.1109%2fSMC52423.2021.9658851&partnerID=40&md5=9472abc4fceb04dd351c66a9d3b2a30b}, abstract = {This paper focuses on the optimal scheduling of the charging and discharging strategies of a community energy storage (CES) system, which is shared by the prosumers belonging to a grid-connected energy community. The prosumers own renewable energy sources (RESs), while they can buy/sell their energy imbalance directly from/to the power grid. For the sake of increasing the penetration of RESs and reducing the operating cost, prosumers leverage on the shared CES: in particular, each user can only employ a portion of the overall CES charge/discharge profile. Differently from the related literature, where storage devices are individually owned and the battery degradation aspects are typically disregarded, we propose a novel control mechanism based on noncooperative game theory, which allows users to minimize their energy cost as well as concur on the CES resources allocation with minimal-degradation. The effectiveness of the method is validated through numerical experiments on a realistic case study, where a shared CES supplies energy to the local community of residential prosumers. Finally, the comparison with a centralized control approach shows that the proposed framework allows all prosumers to achieve a fair cost-optimal utilization of the shared CES. © 2021 IEEE.}, author_keywords = {decentralized control; energy community; energy storage; game theory; noncooperative control}, keywords = {Decentralized control; Electric power transmission networks; Energy storage; Numerical methods; Renewable energy resources; Smart power grids; Virtual storage; Community energy; Control approach; Decentralised; Decentralised control; Energy; Energy community; Noncooperative control; Optimal scheduling; Renewable energy source; Storage systems; Game theory}, type = {Conference paper}, publication_stage = {Final}, source = {Scopus}, note = {Cited by: 1} }
- Scarabaggio, P., Carli, R., Jantzen, J. & Dotoli, M. (2021) Stochastic model predictive control of community energy storage under high renewable penetration IN 2021 29th Mediterranean Conference on Control and Automation, MED 2021., 973 – 978. doi:10.1109/MED51440.2021.9480353
[BibTeX] [Abstract] [Download PDF]This paper focuses on the robust optimal on-line scheduling of a grid-connected energy community, where users are equipped with non-controllable (NCLs) and controllable loads (CLs) and share renewable energy sources (RESs) and a community energy storage system (CESS). Leveraging on the pricing signals gathered from the power grid and the predicted values for local production and demand, the energy activities inside the community are decided by a community energy manager. Differently from literature contributions commonly focused on deterministic optimal control schemes, to cope with the uncertainty that affects the forecast of the inflexible demand profile and the renewable production curve, we propose a Stochastic Model Predictive Control (MPC) approach aimed at minimizing the community energy costs. The effectiveness of the method is validated through numerical experiments on the marina of Ballen, Samso (Denmark). The comparison with a standard deterministic optimal control approach shows that the proposed stochastic MPC achieves higher performance in terms of minimized energy cost and maximized self-consumption of on-site production. © 2021 IEEE.
@CONFERENCE{Scarabaggio2021973, author = {Scarabaggio, Paolo and Carli, Raffaele and Jantzen, Jan and Dotoli, Mariagrazia}, title = {Stochastic model predictive control of community energy storage under high renewable penetration}, year = {2021}, journal = {2021 29th Mediterranean Conference on Control and Automation, MED 2021}, pages = {973 – 978}, doi = {10.1109/MED51440.2021.9480353}, url = {https://www.scopus.com/inward/record.uri?eid=2-s2.0-85113644274&doi=10.1109%2fMED51440.2021.9480353&partnerID=40&md5=6156faadbaf382124749771a715a60df}, abstract = {This paper focuses on the robust optimal on-line scheduling of a grid-connected energy community, where users are equipped with non-controllable (NCLs) and controllable loads (CLs) and share renewable energy sources (RESs) and a community energy storage system (CESS). Leveraging on the pricing signals gathered from the power grid and the predicted values for local production and demand, the energy activities inside the community are decided by a community energy manager. Differently from literature contributions commonly focused on deterministic optimal control schemes, to cope with the uncertainty that affects the forecast of the inflexible demand profile and the renewable production curve, we propose a Stochastic Model Predictive Control (MPC) approach aimed at minimizing the community energy costs. The effectiveness of the method is validated through numerical experiments on the marina of Ballen, Samso (Denmark). The comparison with a standard deterministic optimal control approach shows that the proposed stochastic MPC achieves higher performance in terms of minimized energy cost and maximized self-consumption of on-site production. © 2021 IEEE.}, author_keywords = {Community energy storage; Community renewables; Energy community; Energy management; On-line energy scheduling; Stochastic model predictive control}, keywords = {Electric power transmission networks; Energy storage; Model predictive control; Numerical methods; Optimization; Predictive control systems; Renewable energy resources; Stochastic control systems; Stochastic systems; Comparison with a standard; Controllable loads; Local production; Numerical experiments; On-site production; Online scheduling; Optimal control scheme; Renewable energy source; Stochastic models}, type = {Conference paper}, publication_stage = {Final}, source = {Scopus}, note = {Cited by: 11} }
- Bozza, A., Cavone, G., Carli, R., Mazzoccoli, L. & Dotoli, M. (2021) An MPC-based Approach for the Feedback Control of the Cold Sheet Metal Forming Process IN IEEE International Conference on Automation Science and Engineering., 286 – 291. doi:10.1109/CASE49439.2021.9551602
[BibTeX] [Abstract] [Download PDF]In the automotive sector the cold forming of metal sheets is one of the main production activities. However, it is also one of the main source of production wastes. The generally adopted strategy to reduce the number of abnormal stamped parts is the feedback control of the stamping press (i.e., the machine control), while the feedback control of the stamping process is rarely considered. The process control, differently from the press control, can allow the monitoring of the state of the stamped part during the formation phase and the provision of corrective actions in case of abnormal behaviors of the metal sheet, thus ensuring a more precise control of the process. In this context, this paper presents a novel methodology for the cold metal forming process control based on Model Predictive Control (MPC). Firstly, a dynamical model of the system is defined that describes the draw-in of n critical points of the metal sheet as a function of the Blank Holder Force (BHF) and the punch stroke. Then, two different MPC-based real-time controllers are built for two different types of press configuration: the monolithic and the differential one. In the first case, a mono-MPC control system evaluates the draw-in of n critical points and computes a single couple of control signals (i.e., the BHF and the punch stroke). In the second case, a multi-MPC control system computes n different couples of control signals, i.e., one for each monitored draw-in. Finally, a case study is presented with the aim to test both the architectures, considering several simulation scenarios (with or without external disturbances on the plant), in order to make a control system architectures comparison in terms of tracking errors and workpiece quality. © 2021 IEEE.
@CONFERENCE{Bozza2021286, author = {Bozza, Augusto and Cavone, Graziana and Carli, Raffaele and Mazzoccoli, Luigi and Dotoli, Mariagrazia}, title = {An MPC-based Approach for the Feedback Control of the Cold Sheet Metal Forming Process}, year = {2021}, journal = {IEEE International Conference on Automation Science and Engineering}, volume = {2021-August}, pages = {286 – 291}, doi = {10.1109/CASE49439.2021.9551602}, url = {https://www.scopus.com/inward/record.uri?eid=2-s2.0-85117041530&doi=10.1109%2fCASE49439.2021.9551602&partnerID=40&md5=8f6b0f6133565ad0c087ea675cf9c3b9}, abstract = {In the automotive sector the cold forming of metal sheets is one of the main production activities. However, it is also one of the main source of production wastes. The generally adopted strategy to reduce the number of abnormal stamped parts is the feedback control of the stamping press (i.e., the machine control), while the feedback control of the stamping process is rarely considered. The process control, differently from the press control, can allow the monitoring of the state of the stamped part during the formation phase and the provision of corrective actions in case of abnormal behaviors of the metal sheet, thus ensuring a more precise control of the process. In this context, this paper presents a novel methodology for the cold metal forming process control based on Model Predictive Control (MPC). Firstly, a dynamical model of the system is defined that describes the draw-in of n critical points of the metal sheet as a function of the Blank Holder Force (BHF) and the punch stroke. Then, two different MPC-based real-time controllers are built for two different types of press configuration: the monolithic and the differential one. In the first case, a mono-MPC control system evaluates the draw-in of n critical points and computes a single couple of control signals (i.e., the BHF and the punch stroke). In the second case, a multi-MPC control system computes n different couples of control signals, i.e., one for each monitored draw-in. Finally, a case study is presented with the aim to test both the architectures, considering several simulation scenarios (with or without external disturbances on the plant), in order to make a control system architectures comparison in terms of tracking errors and workpiece quality. © 2021 IEEE.}, author_keywords = {Metal forming; Model Predictive Control; process control; real-time feedback control}, keywords = {Automotive industry; Feedback control; Metal forming; Metals; Predictive control systems; Presses (machine tools); Sheet metal; Stamping; Blank holder forces; Cold sheets; Control signal; Draw-in; Metal-forming process; Model-predictive control; Punch stroke; Real-time feedback; Real-time feedback control; Sheet metal forming; Model predictive control}, type = {Conference paper}, publication_stage = {Final}, source = {Scopus}, note = {Cited by: 6} }
- Hosseini, S. M., Carli, R. & Dotoli, M. (2021) Robust Optimal Energy Management of a Residential Microgrid under Uncertainties on Demand and Renewable Power Generation. IN IEEE Transactions on Automation Science and Engineering, 18.618 – 637. doi:10.1109/TASE.2020.2986269
[BibTeX] [Abstract] [Download PDF]Smart microgrids are experiencing an increasing growth due to their economic, social, and environmental benefits. However, the inherent intermittency of renewable energy sources (RESs) and users’ behavior lead to significant uncertainty, which implies important challenges on the system design. Facing this issue, this article proposes a novel robust framework for the day-ahead energy scheduling of a residential microgrid comprising interconnected smart users, each owning individual RESs, noncontrollable loads (NCLs), energy- and comfort-based CLs, and individual plug-in electric vehicles (PEVs). Moreover, users share a number of RESs and an energy storage system (ESS). We assume that the microgrid can buy/sell energy from/to the grid subject to quadratic/linear dynamic pricing functions. The objective of scheduling is minimizing the expected energy cost while satisfying device/comfort/contractual constraints, including feasibility constraints on energy transfer between users and the grid under RES generation and users’ demand uncertainties. To this aim, first, we formulate a min-max robust problem to obtain the optimal CLs scheduling and charging/discharging strategies of the ESS and PEVs. Then, based on the duality theory for multi-objective optimization, we transform the min-max problem into a mixed-integer quadratic programming problem to solve the equivalent robust counterpart of the scheduling problem effectively. We deal with the conservativeness of the proposed approach for different scenarios and quantify the effects of the budget of uncertainty on the cost saving, the peak-to-average ratio, and the constraints’ violation rate. We validate the effectiveness of the method on a simulated case study and we compare the results with a related robust approach. Note to Practitioners – This article is motivated by the emerging need for intelligent demand-side management (DSM) approaches in smart microgrids in the presence of both power generation and demand uncertainties. The proposed robust energy scheduling strategy allows the decision maker (i.e., the energy manager of the microgrid) to make a satisfactory tradeoff between the users’ payment and constraints’ violation rate considering the energy cost saving, the system technical limitations and the users’ comfort by adjusting the values of the budget of uncertainty. The proposed framework is generic and flexible as it can be applied to different structures of microgrids considering various types of uncertainties in energy generation or demand. © 2004-2012 IEEE.
@ARTICLE{Hosseini2021618, author = {Hosseini, Seyed Mohsen and Carli, Raffaele and Dotoli, Mariagrazia}, title = {Robust Optimal Energy Management of a Residential Microgrid under Uncertainties on Demand and Renewable Power Generation}, year = {2021}, journal = {IEEE Transactions on Automation Science and Engineering}, volume = {18}, number = {2}, pages = {618 – 637}, doi = {10.1109/TASE.2020.2986269}, url = {https://www.scopus.com/inward/record.uri?eid=2-s2.0-85100266630&doi=10.1109%2fTASE.2020.2986269&partnerID=40&md5=ab5c0a8a4eb48977e42d7186b3d4fc3b}, abstract = {Smart microgrids are experiencing an increasing growth due to their economic, social, and environmental benefits. However, the inherent intermittency of renewable energy sources (RESs) and users' behavior lead to significant uncertainty, which implies important challenges on the system design. Facing this issue, this article proposes a novel robust framework for the day-ahead energy scheduling of a residential microgrid comprising interconnected smart users, each owning individual RESs, noncontrollable loads (NCLs), energy- and comfort-based CLs, and individual plug-in electric vehicles (PEVs). Moreover, users share a number of RESs and an energy storage system (ESS). We assume that the microgrid can buy/sell energy from/to the grid subject to quadratic/linear dynamic pricing functions. The objective of scheduling is minimizing the expected energy cost while satisfying device/comfort/contractual constraints, including feasibility constraints on energy transfer between users and the grid under RES generation and users' demand uncertainties. To this aim, first, we formulate a min-max robust problem to obtain the optimal CLs scheduling and charging/discharging strategies of the ESS and PEVs. Then, based on the duality theory for multi-objective optimization, we transform the min-max problem into a mixed-integer quadratic programming problem to solve the equivalent robust counterpart of the scheduling problem effectively. We deal with the conservativeness of the proposed approach for different scenarios and quantify the effects of the budget of uncertainty on the cost saving, the peak-to-average ratio, and the constraints' violation rate. We validate the effectiveness of the method on a simulated case study and we compare the results with a related robust approach. Note to Practitioners - This article is motivated by the emerging need for intelligent demand-side management (DSM) approaches in smart microgrids in the presence of both power generation and demand uncertainties. The proposed robust energy scheduling strategy allows the decision maker (i.e., the energy manager of the microgrid) to make a satisfactory tradeoff between the users' payment and constraints' violation rate considering the energy cost saving, the system technical limitations and the users' comfort by adjusting the values of the budget of uncertainty. The proposed framework is generic and flexible as it can be applied to different structures of microgrids considering various types of uncertainties in energy generation or demand. © 2004-2012 IEEE.}, author_keywords = {Demand-side management (DSM); microgrid; mixed-integer quadratic programming (MIQP); optimal energy scheduling; optimization; robust control}, keywords = {Budget control; Costs; Decision making; Electric utilities; Electromagnetic wave emission; Energy storage; Energy transfer; Housing; Integer programming; Microgrids; Multiobjective optimization; Plug-in electric vehicles; Quadratic programming; Renewable energy resources; Scheduling; Energy storage systems; Environmental benefits; Mixed integer quadratic programming; Peak to average ratios; Renewable energy source; Renewable power generation; Scheduling strategies; Technical limitations; Demand side management}, type = {Article}, publication_stage = {Final}, source = {Scopus}, note = {Cited by: 147} }
- Scarabaggio, P., Carli, R., Cavone, G., Epicoco, N. & Dotoli, M. (2021) Modeling, estimation, and analysis of COVID-19 secondary waves: The Case of the Italian Country IN 2021 29th Mediterranean Conference on Control and Automation, MED 2021., 794 – 800. doi:10.1109/MED51440.2021.9480319
[BibTeX] [Abstract] [Download PDF]The recent trends of the COVID-19 research have been devoted to disease transmission modeling, with the aim of investigating the effects of different mitigation strategies mainly through scenario-based simulations. In this context we propose a novel non-linear time-varying model that effectively supports policy-makers in predicting and analyzing the dynamics of COVID-19 secondary waves. Specifically, this paper proposes an accurate SIRUCQTHE epidemiological model to get reliable predictions on the pandemic dynamics. Differently from the related literature, in the fitting phase, we make use of the google mobility reports to identify and predict the evolution of the infection rate. The effectiveness of the presented method is tested on the network of Italian regions. First, we describe the Italian epidemiological scenario in the COVID-19 second wave of contagions, showing the raw data available for the Italian scenario and discussing the main assumptions on the system parameters. Then, we present the different steps of the procedure used for the dynamical fitting of the SIRUCQTHE model. Finally, we compare the estimation results with the real data on the COVID-19 secondary waves in Italy. Provided the availability of reliable data to calibrate the model in heterogeneous scenarios, the proposed approach can be easily extended to cope with other scenarios. © 2021 IEEE.
@CONFERENCE{Scarabaggio2021794, author = {Scarabaggio, Paolo and Carli, Raffaele and Cavone, Graziana and Epicoco, Nicola and Dotoli, Mariagrazia}, title = {Modeling, estimation, and analysis of COVID-19 secondary waves: The Case of the Italian Country}, year = {2021}, journal = {2021 29th Mediterranean Conference on Control and Automation, MED 2021}, pages = {794 – 800}, doi = {10.1109/MED51440.2021.9480319}, url = {https://www.scopus.com/inward/record.uri?eid=2-s2.0-85113602900&doi=10.1109%2fMED51440.2021.9480319&partnerID=40&md5=980feaa724975719c46c214ad1dcbfed}, abstract = {The recent trends of the COVID-19 research have been devoted to disease transmission modeling, with the aim of investigating the effects of different mitigation strategies mainly through scenario-based simulations. In this context we propose a novel non-linear time-varying model that effectively supports policy-makers in predicting and analyzing the dynamics of COVID-19 secondary waves. Specifically, this paper proposes an accurate SIRUCQTHE epidemiological model to get reliable predictions on the pandemic dynamics. Differently from the related literature, in the fitting phase, we make use of the google mobility reports to identify and predict the evolution of the infection rate. The effectiveness of the presented method is tested on the network of Italian regions. First, we describe the Italian epidemiological scenario in the COVID-19 second wave of contagions, showing the raw data available for the Italian scenario and discussing the main assumptions on the system parameters. Then, we present the different steps of the procedure used for the dynamical fitting of the SIRUCQTHE model. Finally, we compare the estimation results with the real data on the COVID-19 secondary waves in Italy. Provided the availability of reliable data to calibrate the model in heterogeneous scenarios, the proposed approach can be easily extended to cope with other scenarios. © 2021 IEEE.}, author_keywords = {COVID-19; Dynamical fitting; Estimation; Identification; Pandemic modeling}, keywords = {Forecasting; Disease transmission; Epidemiological modeling; Estimation results; Infection rates; Italian regions; Mitigation strategy; Predicting and analyzing; Scenario-based simulations; Shear waves}, type = {Conference paper}, publication_stage = {Final}, source = {Scopus}, note = {Cited by: 2} }
- Scarabaggio, P., Carli, R., Cavone, G., Epicoco, N. & Dotoli, M. (2021) Modeling, Estimation, and Optimal Control of Anti-COVID-19 Multi-dose Vaccine Administration IN IEEE International Conference on Automation Science and Engineering., 990 – 995. doi:10.1109/CASE49439.2021.9551418
[BibTeX] [Abstract] [Download PDF]The recent trends of the COVID-19 research are being devoted to disease transmission modeling in presence of vaccinated individuals, while the emerging needs are being focused on developing effective strategies for the optimal distribution of vaccine between population. In this context, we propose a novel non-linear time-varying model that effectively supports policy-makers in predicting and analyzing the dynamics of COVID-19 when partially and fully immune individuals are included in the population. Differently from the related literature, where the common strategies typically rely on the prioritization of the different classes of individuals, we propose a novel Model Predictive Control approach to optimally control the multi-dose vaccine administration in the case the available number of doses is not sufficient to cover the whole population. Focusing on the minimization of the expected number of deaths, the approach discriminates between the number of first and second doses. We calibrate the model on the Israeli scenario using real data and we estimate the impact of the vaccine administration on the virus dynamics. Lastly, we assess the impact of the first dose of the Pfizer’s vaccine confirming the results of clinical tests. © 2021 IEEE.
@CONFERENCE{Scarabaggio2021990, author = {Scarabaggio, Paolo and Carli, Raffaele and Cavone, Graziana and Epicoco, Nicola and Dotoli, Mariagrazia}, title = {Modeling, Estimation, and Optimal Control of Anti-COVID-19 Multi-dose Vaccine Administration}, year = {2021}, journal = {IEEE International Conference on Automation Science and Engineering}, volume = {2021-August}, pages = {990 – 995}, doi = {10.1109/CASE49439.2021.9551418}, url = {https://www.scopus.com/inward/record.uri?eid=2-s2.0-85117035432&doi=10.1109%2fCASE49439.2021.9551418&partnerID=40&md5=489bcdcd710fb127ad522dbebe163265}, abstract = {The recent trends of the COVID-19 research are being devoted to disease transmission modeling in presence of vaccinated individuals, while the emerging needs are being focused on developing effective strategies for the optimal distribution of vaccine between population. In this context, we propose a novel non-linear time-varying model that effectively supports policy-makers in predicting and analyzing the dynamics of COVID-19 when partially and fully immune individuals are included in the population. Differently from the related literature, where the common strategies typically rely on the prioritization of the different classes of individuals, we propose a novel Model Predictive Control approach to optimally control the multi-dose vaccine administration in the case the available number of doses is not sufficient to cover the whole population. Focusing on the minimization of the expected number of deaths, the approach discriminates between the number of first and second doses. We calibrate the model on the Israeli scenario using real data and we estimate the impact of the vaccine administration on the virus dynamics. Lastly, we assess the impact of the first dose of the Pfizer's vaccine confirming the results of clinical tests. © 2021 IEEE.}, author_keywords = {COVID-19; model predictive control; pandemic modeling; vaccine; vaccine distribution}, keywords = {Model predictive control; Viruses; COVID-19; Disease transmission; Estimation controls; Model estimation; Model-predictive control; Modelling controls; Optimal controls; Pandemic modeling; Recent trends; Vaccine distributions; Vaccines}, type = {Conference paper}, publication_stage = {Final}, source = {Scopus}, note = {Cited by: 7; All Open Access, Bronze Open Access, Green Open Access} }
- Helmi, A. M., Carli, R., Dotoli, M. & Ramadan, H. S. (2021) Harris hawks optimization for the efficient reconfiguration of distribution networks IN 2021 29th Mediterranean Conference on Control and Automation, MED 2021., 214 – 219. doi:10.1109/MED51440.2021.9480179
[BibTeX] [Abstract] [Download PDF]Improving the efficiency of distribution networks (DNs) is nowadays a challenging objective for modern power grids equipped with distributed generation and storage. In this context, the so-called network reconfiguration problem can be solved to obtain the optimal DN topology that minimizes the total power losses, while ensuring the voltage profile enhancement. The DN reconfiguration problem has NP-hard complexity; hence, finding near-optimal solutions in reasonable time is still an open research need. Facing this issue, this paper proposes a novel metaheuristic approach, where the recent Harris Hawks optimization algorithm is used to efficiently obtain near-optimal configurations. The effectiveness of the proposed method is validated through numerical experiments on the IEEE 85-bus system, comparing the achieved performance with the results obtained by other related techniques. © 2021 IEEE.
@CONFERENCE{Helmi2021214, author = {Helmi, Ahmed M. and Carli, Raffaele and Dotoli, Mariagrazia and Ramadan, Haitham S.}, title = {Harris hawks optimization for the efficient reconfiguration of distribution networks}, year = {2021}, journal = {2021 29th Mediterranean Conference on Control and Automation, MED 2021}, pages = {214 – 219}, doi = {10.1109/MED51440.2021.9480179}, url = {https://www.scopus.com/inward/record.uri?eid=2-s2.0-85113690851&doi=10.1109%2fMED51440.2021.9480179&partnerID=40&md5=ae29f6caf996f43c23ab7874ded72442}, abstract = {Improving the efficiency of distribution networks (DNs) is nowadays a challenging objective for modern power grids equipped with distributed generation and storage. In this context, the so-called network reconfiguration problem can be solved to obtain the optimal DN topology that minimizes the total power losses, while ensuring the voltage profile enhancement. The DN reconfiguration problem has NP-hard complexity; hence, finding near-optimal solutions in reasonable time is still an open research need. Facing this issue, this paper proposes a novel metaheuristic approach, where the recent Harris Hawks optimization algorithm is used to efficiently obtain near-optimal configurations. The effectiveness of the proposed method is validated through numerical experiments on the IEEE 85-bus system, comparing the achieved performance with the results obtained by other related techniques. © 2021 IEEE.}, author_keywords = {Distribution Network Reconfiguration; Harris Hawks Optimization; Metaheuristic Optimization; Microgrids}, keywords = {Electric power transmission networks; NP-hard; Numerical methods; Distributed generation and storage; Meta-heuristic approach; Near-optimal solutions; Network re-configuration; Optimization algorithms; Reconfiguration of distribution networks; Reconfiguration problems; Voltage profile enhancements; Optimization}, type = {Conference paper}, publication_stage = {Final}, source = {Scopus}, note = {Cited by: 2} }
- Proia, S., Carli, R., Cavone, G. & Dotoli, M. (2021) A Literature Review on Control Techniques for Collaborative Robotics in Industrial Applications IN IEEE International Conference on Automation Science and Engineering., 591 – 596. doi:10.1109/CASE49439.2021.9551600
[BibTeX] [Abstract] [Download PDF]One of the key enabling technologies that has made Industry 4.0 a concrete reality is without doubt collaborative robotics, which is also evolving as a fundamental pillar of the next revolution, the so-called Industry 5.0. The improvement of safety and employees’ well-being, together with the increment of profitability and productivity, are indeed the main goals of human-robot collaboration (HRC) in the industrial setting. The robotic controller design and the analysis of existing decision and control techniques are crucially needed to develop innovative models and state-of-the-art methodologies for a safe, ergonomic, and efficient HRC. To this aim, this article presents an accurate review of the most recent and relevant papers in the related field, focusing on the control perspective. All the surveyed works are carefully selected and categorized by target (i.e., safety, ergonomics, and efficiency), and then by problem and type of control, in presence or absence of optimization. Finally, the discussion of the achieved results and the analysis of the emerging challenges in this research field are reported, highlighting the identified gaps and promising future developments in the context of the digital evolution. © 2021 IEEE.
@CONFERENCE{Proia2021591, author = {Proia, Silvia and Carli, Raffaele and Cavone, Graziana and Dotoli, Mariagrazia}, title = {A Literature Review on Control Techniques for Collaborative Robotics in Industrial Applications}, year = {2021}, journal = {IEEE International Conference on Automation Science and Engineering}, volume = {2021-August}, pages = {591 – 596}, doi = {10.1109/CASE49439.2021.9551600}, url = {https://www.scopus.com/inward/record.uri?eid=2-s2.0-85116978273&doi=10.1109%2fCASE49439.2021.9551600&partnerID=40&md5=a834d330c2d2bedcecdff62c8eaceae7}, abstract = {One of the key enabling technologies that has made Industry 4.0 a concrete reality is without doubt collaborative robotics, which is also evolving as a fundamental pillar of the next revolution, the so-called Industry 5.0. The improvement of safety and employees' well-being, together with the increment of profitability and productivity, are indeed the main goals of human-robot collaboration (HRC) in the industrial setting. The robotic controller design and the analysis of existing decision and control techniques are crucially needed to develop innovative models and state-of-the-art methodologies for a safe, ergonomic, and efficient HRC. To this aim, this article presents an accurate review of the most recent and relevant papers in the related field, focusing on the control perspective. All the surveyed works are carefully selected and categorized by target (i.e., safety, ergonomics, and efficiency), and then by problem and type of control, in presence or absence of optimization. Finally, the discussion of the achieved results and the analysis of the emerging challenges in this research field are reported, highlighting the identified gaps and promising future developments in the context of the digital evolution. © 2021 IEEE.}, keywords = {Accident prevention; Controllers; Ergonomics; Industry 4.0; Robotics; Control techniques; Controller designs; Enabling technologies; Human-robot collaboration; Industrial settings; Innovative models; Literature reviews; Robotic controllers; State of the art; Well being; Collaborative robots}, type = {Conference paper}, publication_stage = {Final}, source = {Scopus}, note = {Cited by: 14} }
2020
- Carli, R., Dotoli, M., Jantzen, J., Kristensen, M. & Ben Othman, S. (2020) Energy scheduling of a smart microgrid with shared photovoltaic panels and storage: The case of the Ballen marina in Samsø. IN Energy, 198.. doi:10.1016/j.energy.2020.117188
[BibTeX] [Abstract] [Download PDF]This paper focuses on the Model Predictive Control (MPC) based energy scheduling of a smart microgrid equipped with non-controllable (i.e., with fixed power profile) and controllable (i.e., with flexible and programmable operation) electrical appliances, as well as photovoltaic (PV) panels, and a battery energy storage system (BESS). The proposed control strategy aims at a simultaneous optimal planning of the controllable loads, the shared resources (i.e., the storage system charge/discharge and renewable energy usage), and the energy exchange with the grid. The control scheme relies on an iterative finite horizon on-line optimization, implementing a mixed integer linear programming energy scheduling algorithm to maximize the self-supply with solar energy and/or minimize the daily cost of energy bought from the grid under time-varying energy pricing. At each time step, the resulting optimization problem is solved providing the optimal operations of controllable loads, the optimal amount of energy to be bought/sold from/to the grid, and the optimal charging/discharging profile for the BESS. The proposed energy scheduling approach is applied to the demand side management control of the marina of Ballen, Samsø (Denmark), where a smart microgrid is currently being implemented as a demonstrator in the Horizon2020 European research project SMILE. Simulations considering the marina electric consumption (340 boat sockets, a service building equipped with a sauna and a wastewater pumping station, and the harbour master’s office equipped with a heat pump), PV production (60kWp), and the BESS (237 kWh capacity) based on a public real dataset are carried out on a one year time series with a 1 h resolution. Simulations indicate that the proposed approach allows 90% exploitation of the production of the PV plant. Furthermore, results are compared to a naïve control approach. The MPC based energy scheduling improves the self-supply by 1.6% compared to the naïve control. Optimization of the business economy using the MPC approach, instead, yields to 8.2% savings in the yearly energy cost with respect to the naïve approach. © 2020 Elsevier Ltd
@ARTICLE{Carli2020, author = {Carli, Raffaele and Dotoli, Mariagrazia and Jantzen, Jan and Kristensen, Michael and Ben Othman, Sarah}, title = {Energy scheduling of a smart microgrid with shared photovoltaic panels and storage: The case of the Ballen marina in Samsø}, year = {2020}, journal = {Energy}, volume = {198}, doi = {10.1016/j.energy.2020.117188}, url = {https://www.scopus.com/inward/record.uri?eid=2-s2.0-85082001819&doi=10.1016%2fj.energy.2020.117188&partnerID=40&md5=6477046a8e48af719b8ab95085e45579}, abstract = {This paper focuses on the Model Predictive Control (MPC) based energy scheduling of a smart microgrid equipped with non-controllable (i.e., with fixed power profile) and controllable (i.e., with flexible and programmable operation) electrical appliances, as well as photovoltaic (PV) panels, and a battery energy storage system (BESS). The proposed control strategy aims at a simultaneous optimal planning of the controllable loads, the shared resources (i.e., the storage system charge/discharge and renewable energy usage), and the energy exchange with the grid. The control scheme relies on an iterative finite horizon on-line optimization, implementing a mixed integer linear programming energy scheduling algorithm to maximize the self-supply with solar energy and/or minimize the daily cost of energy bought from the grid under time-varying energy pricing. At each time step, the resulting optimization problem is solved providing the optimal operations of controllable loads, the optimal amount of energy to be bought/sold from/to the grid, and the optimal charging/discharging profile for the BESS. The proposed energy scheduling approach is applied to the demand side management control of the marina of Ballen, Samsø (Denmark), where a smart microgrid is currently being implemented as a demonstrator in the Horizon2020 European research project SMILE. Simulations considering the marina electric consumption (340 boat sockets, a service building equipped with a sauna and a wastewater pumping station, and the harbour master's office equipped with a heat pump), PV production (60kWp), and the BESS (237 kWh capacity) based on a public real dataset are carried out on a one year time series with a 1 h resolution. Simulations indicate that the proposed approach allows 90% exploitation of the production of the PV plant. Furthermore, results are compared to a naïve control approach. The MPC based energy scheduling improves the self-supply by 1.6% compared to the naïve control. Optimization of the business economy using the MPC approach, instead, yields to 8.2% savings in the yearly energy cost with respect to the naïve approach. © 2020 Elsevier Ltd}, author_keywords = {Demand side management; Energy management; Energy storage; Microgrid; Model predictive control; On-line scheduling; Optimization algorithm; Renewable energy}, keywords = {Denmark; Costs; Demand side management; Economics; Electric utilities; Energy management; Energy storage; Energy utilization; Integer programming; Iterative methods; Marinas; Microgrids; Model predictive control; Photovoltaic cells; Predictive control systems; Pumping plants; Scheduling; Scheduling algorithms; Solar energy; Battery energy storage systems; European research project; Micro grid; Mixed integer linear programming; Online scheduling; Optimization algorithms; Renewable energies; Wastewater pumping station; alternative energy; demand-side management; energy storage; exploitation; linear programing; optimization; photovoltaic system; pumping; smart grid; time series; wastewater; Electric power system control}, type = {Article}, publication_stage = {Final}, source = {Scopus}, note = {Cited by: 79; All Open Access, Green Open Access} }
- Carli, R. & Dotoli, M. (2020) A dynamic programming approach for the decentralized control of discrete optimizers with quadratic utilities and shared constraint IN 2020 28th Mediterranean Conference on Control and Automation, MED 2020., 611 – 616. doi:10.1109/MED48518.2020.9183012
[BibTeX] [Abstract] [Download PDF]This paper addresses the problem of controlling a large set of agents, each with a quadratic utility function depending on individual combinatorial choices, and all sharing an affine constraint on available resources. Such a problem is formulated as an integer mono-constrained bounded quadratic knapsack problem. Differently from the centralized approaches typically proposed in the related literature, we present a new decentralized algorithm to solve the problem approximately in polynomial time by decomposing it into a finite series of sub-problems. We assume a minimal communication structure through the presence of a central coordinator that ensures the information exchange between agents. The proposed solution relies on a decentralized control algorithm that combines discrete dynamic programming with additive decomposition and value functions approximation. The optimality and complexity of the presented strategy are discussed, highlighting that the algorithm constitutes a fully polynomial approximation scheme. Numerical experiments are presented to show the effectiveness of the approach in the optimal resolution of large-scale instances. © 2020 IEEE.
@CONFERENCE{Carli2020611, author = {Carli, Raffaele and Dotoli, Mariagrazia}, title = {A dynamic programming approach for the decentralized control of discrete optimizers with quadratic utilities and shared constraint}, year = {2020}, journal = {2020 28th Mediterranean Conference on Control and Automation, MED 2020}, pages = {611 – 616}, doi = {10.1109/MED48518.2020.9183012}, url = {https://www.scopus.com/inward/record.uri?eid=2-s2.0-85092158668&doi=10.1109%2fMED48518.2020.9183012&partnerID=40&md5=e3c2836d54651610b499a9686ac98fe7}, abstract = {This paper addresses the problem of controlling a large set of agents, each with a quadratic utility function depending on individual combinatorial choices, and all sharing an affine constraint on available resources. Such a problem is formulated as an integer mono-constrained bounded quadratic knapsack problem. Differently from the centralized approaches typically proposed in the related literature, we present a new decentralized algorithm to solve the problem approximately in polynomial time by decomposing it into a finite series of sub-problems. We assume a minimal communication structure through the presence of a central coordinator that ensures the information exchange between agents. The proposed solution relies on a decentralized control algorithm that combines discrete dynamic programming with additive decomposition and value functions approximation. The optimality and complexity of the presented strategy are discussed, highlighting that the algorithm constitutes a fully polynomial approximation scheme. Numerical experiments are presented to show the effectiveness of the approach in the optimal resolution of large-scale instances. © 2020 IEEE.}, author_keywords = {Decentralized optimization; Dynamic programming; Fully polynomial-time approximation scheme; Knapsack problem}, keywords = {Approximation algorithms; Combinatorial optimization; Dynamic programming; Polynomial approximation; Additive decomposition; Centralized approaches; Communication structures; Decentralized algorithms; Information exchanges; Numerical experiments; Optimal resolution; Quadratic knapsack problems; Decentralized control}, type = {Conference paper}, publication_stage = {Final}, source = {Scopus}, note = {Cited by: 0} }
- Scarabaggio, P., Carli, R. & Dotoli, M. (2020) A fast and effective algorithm for influence maximization in large-scale independent cascade networks IN 7th International Conference on Control, Decision and Information Technologies, CoDIT 2020., 639 – 644. doi:10.1109/CoDIT49905.2020.9263914
[BibTeX] [Abstract] [Download PDF]A characteristic of social networks is the ability to quickly spread information between a large group of people. The widespread use of online social networks (e.g., Facebook) increases the interest of researchers on how influence propagates through these networks. One of the most important research issues in this field is the so-called influence maximization problem, which essentially consists in selecting the most influential users (i.e., those who are able to maximize the spread of influence through the social network). Due to its practical importance in various applications (e.g., viral marketing), such a problem has been studied in several variants. Nevertheless, the current open challenge in the resolution of the influence maximization problem still concerns achieving a good trade-off between accuracy and computational time. In this context, based on independent cascade modeling of social networks, we propose a novel low-complexity and highly accurate algorithm for selecting an initial group of nodes to maximize the spread of influence in large-scale networks. In particular, the key idea consists in iteratively removing the overlap of influence spread induced by different seed nodes. The application to several numerical experiments based on real datasets proves that the proposed algorithm effectively finds practical near-optimal solutions of the addressed influence maximization problem in a computationally efficient fashion. Finally, the comparison with the state of the art algorithms demonstrates that in large scale scenarios the proposed approach shows higher performance in terms of influence spread and running time. © 2020 IEEE.
@CONFERENCE{Scarabaggio2020639, author = {Scarabaggio, Paolo and Carli, Raffaele and Dotoli, Mariagrazia}, title = {A fast and effective algorithm for influence maximization in large-scale independent cascade networks}, year = {2020}, journal = {7th International Conference on Control, Decision and Information Technologies, CoDIT 2020}, pages = {639 – 644}, doi = {10.1109/CoDIT49905.2020.9263914}, url = {https://www.scopus.com/inward/record.uri?eid=2-s2.0-85098236199&doi=10.1109%2fCoDIT49905.2020.9263914&partnerID=40&md5=003f7d44f921ecd0bf436dacb2d3e136}, abstract = {A characteristic of social networks is the ability to quickly spread information between a large group of people. The widespread use of online social networks (e.g., Facebook) increases the interest of researchers on how influence propagates through these networks. One of the most important research issues in this field is the so-called influence maximization problem, which essentially consists in selecting the most influential users (i.e., those who are able to maximize the spread of influence through the social network). Due to its practical importance in various applications (e.g., viral marketing), such a problem has been studied in several variants. Nevertheless, the current open challenge in the resolution of the influence maximization problem still concerns achieving a good trade-off between accuracy and computational time. In this context, based on independent cascade modeling of social networks, we propose a novel low-complexity and highly accurate algorithm for selecting an initial group of nodes to maximize the spread of influence in large-scale networks. In particular, the key idea consists in iteratively removing the overlap of influence spread induced by different seed nodes. The application to several numerical experiments based on real datasets proves that the proposed algorithm effectively finds practical near-optimal solutions of the addressed influence maximization problem in a computationally efficient fashion. Finally, the comparison with the state of the art algorithms demonstrates that in large scale scenarios the proposed approach shows higher performance in terms of influence spread and running time. © 2020 IEEE.}, keywords = {Economic and social effects; Iterative methods; Computationally efficient; Influence maximizations; Most influential users; Near-optimal solutions; Numerical experiments; On-line social networks; Practical importance; State-of-the-art algorithms; Social networking (online)}, type = {Conference paper}, publication_stage = {Final}, source = {Scopus}, note = {Cited by: 1} }
- Carli, R., Cavone, G., Pippia, T., Schutter, B. D. & Dotoli, M. (2020) A Robust MPC Energy Scheduling Strategy for Multi-Carrier Microgrids IN IEEE International Conference on Automation Science and Engineering., 152 – 158. doi:10.1109/CASE48305.2020.9216875
[BibTeX] [Abstract] [Download PDF]We present a Robust Model Predictive Control (RMPC) approach for multi-carrier microgrids, i.e., microgrids based on gas and electricity. The microgrid that we consider includes thermal loads, electrical loads, renewable energy sources, energy storage systems, heat pumps, and combined heat and power plants. Moreover, the system under control is affected by several external disturbances, e.g., uncertainty in renewable energy generation, electrical and thermal demand. The goal of the controller is to minimize the overall economical cost and the energy exchange with the main grid, while guaranteeing comfort. Whereas several RMPC methods have been developed for electrical or thermal microgrids, little or no attention has been devoted to robust control of multi-carrier microgrids. Therefore, we consider a novel RMPC algorithm that can improve the performance with respect to classical deterministic Model Predictive Control (Det-MPC) controllers in the context of multi-carrier microgrids. The RMPC method relies on the box-uncertainty-set robust optimization, where uncertain parameters are assumed to take their values from different intervals independently. The RMPC approach is able to successfully satisfy the constraints even in the presence of the mentioned disturbances. Simulations of a realistic residential case study show the benefits of the proposed approach with respect to Det-MPC controllers. © 2020 IEEE.
@CONFERENCE{Carli2020152, author = {Carli, Raffaele and Cavone, Graziana and Pippia, Tomas and Schutter, Bart De and Dotoli, Mariagrazia}, title = {A Robust MPC Energy Scheduling Strategy for Multi-Carrier Microgrids}, year = {2020}, journal = {IEEE International Conference on Automation Science and Engineering}, volume = {2020-August}, pages = {152 – 158}, doi = {10.1109/CASE48305.2020.9216875}, url = {https://www.scopus.com/inward/record.uri?eid=2-s2.0-85094114652&doi=10.1109%2fCASE48305.2020.9216875&partnerID=40&md5=92ef257518791ef22242636d1c989285}, abstract = {We present a Robust Model Predictive Control (RMPC) approach for multi-carrier microgrids, i.e., microgrids based on gas and electricity. The microgrid that we consider includes thermal loads, electrical loads, renewable energy sources, energy storage systems, heat pumps, and combined heat and power plants. Moreover, the system under control is affected by several external disturbances, e.g., uncertainty in renewable energy generation, electrical and thermal demand. The goal of the controller is to minimize the overall economical cost and the energy exchange with the main grid, while guaranteeing comfort. Whereas several RMPC methods have been developed for electrical or thermal microgrids, little or no attention has been devoted to robust control of multi-carrier microgrids. Therefore, we consider a novel RMPC algorithm that can improve the performance with respect to classical deterministic Model Predictive Control (Det-MPC) controllers in the context of multi-carrier microgrids. The RMPC method relies on the box-uncertainty-set robust optimization, where uncertain parameters are assumed to take their values from different intervals independently. The RMPC approach is able to successfully satisfy the constraints even in the presence of the mentioned disturbances. Simulations of a realistic residential case study show the benefits of the proposed approach with respect to Det-MPC controllers. © 2020 IEEE.}, author_keywords = {Energy and Environment-Aware Automation; Microgrid; Optimization and Optimal Control; Robust Model Predictive Control; Set-based Uncertainty}, keywords = {Cogeneration plants; Electric energy storage; Electric loads; Microgrids; Model predictive control; Optimization; Predictive control systems; Renewable energy resources; Robust control; Uncertainty analysis; Deterministic modeling; Energy storage systems; External disturbances; Renewable energy generation; Renewable energy source; Robust model predictive controls (RMPC); Scheduling strategies; Uncertain parameters; Controllers}, type = {Conference paper}, publication_stage = {Final}, source = {Scopus}, note = {Cited by: 16} }
- Carli, R., Cavone, G., Epicoco, N., Scarabaggio, P. & Dotoli, M. (2020) Model predictive control to mitigate the COVID-19 outbreak in a multi-region scenario. IN Annual Reviews in Control, 50.373 – 393. doi:10.1016/j.arcontrol.2020.09.005
[BibTeX] [Abstract] [Download PDF]The COVID-19 outbreak is deeply influencing the global social and economic framework, due to restrictive measures adopted worldwide by governments to counteract the pandemic contagion. In multi-region areas such as Italy, where the contagion peak has been reached, it is crucial to find targeted and coordinated optimal exit and restarting strategies on a regional basis to effectively cope with possible onset of further epidemic waves, while efficiently returning the economic activities to their standard level of intensity. Differently from the related literature, where modeling and controlling the pandemic contagion is typically addressed on a national basis, this paper proposes an optimal control approach that supports governments in defining the most effective strategies to be adopted during post-lockdown mitigation phases in a multi-region scenario. Based on the joint use of a non-linear Model Predictive Control scheme and a modified Susceptible-Infected-Recovered (SIR)-based epidemiological model, the approach is aimed at minimizing the cost of the so-called non-pharmaceutical interventions (that is, mitigation strategies), while ensuring that the capacity of the network of regional healthcare systems is not violated. In addition, the proposed approach supports policy makers in taking targeted intervention decisions on different regions by an integrated and structured model, thus both respecting the specific regional health systems characteristics and improving the system-wide performance by avoiding uncoordinated actions of the regions. The methodology is tested on the COVID-19 outbreak data related to the network of Italian regions, showing its effectiveness in properly supporting the definition of effective regional strategies for managing the COVID-19 diffusion. © 2020 Elsevier Ltd
@ARTICLE{Carli2020373, author = {Carli, Raffaele and Cavone, Graziana and Epicoco, Nicola and Scarabaggio, Paolo and Dotoli, Mariagrazia}, title = {Model predictive control to mitigate the COVID-19 outbreak in a multi-region scenario}, year = {2020}, journal = {Annual Reviews in Control}, volume = {50}, pages = {373 – 393}, doi = {10.1016/j.arcontrol.2020.09.005}, url = {https://www.scopus.com/inward/record.uri?eid=2-s2.0-85097753585&doi=10.1016%2fj.arcontrol.2020.09.005&partnerID=40&md5=adce49e71a999948867e93de3ae2e142}, abstract = {The COVID-19 outbreak is deeply influencing the global social and economic framework, due to restrictive measures adopted worldwide by governments to counteract the pandemic contagion. In multi-region areas such as Italy, where the contagion peak has been reached, it is crucial to find targeted and coordinated optimal exit and restarting strategies on a regional basis to effectively cope with possible onset of further epidemic waves, while efficiently returning the economic activities to their standard level of intensity. Differently from the related literature, where modeling and controlling the pandemic contagion is typically addressed on a national basis, this paper proposes an optimal control approach that supports governments in defining the most effective strategies to be adopted during post-lockdown mitigation phases in a multi-region scenario. Based on the joint use of a non-linear Model Predictive Control scheme and a modified Susceptible-Infected-Recovered (SIR)-based epidemiological model, the approach is aimed at minimizing the cost of the so-called non-pharmaceutical interventions (that is, mitigation strategies), while ensuring that the capacity of the network of regional healthcare systems is not violated. In addition, the proposed approach supports policy makers in taking targeted intervention decisions on different regions by an integrated and structured model, thus both respecting the specific regional health systems characteristics and improving the system-wide performance by avoiding uncoordinated actions of the regions. The methodology is tested on the COVID-19 outbreak data related to the network of Italian regions, showing its effectiveness in properly supporting the definition of effective regional strategies for managing the COVID-19 diffusion. © 2020 Elsevier Ltd}, author_keywords = {COVID-19; Epidemic control; MPC; Multi-region SIR model; Pandemic modeling; Post-lockdown mitigation strategies; SIR model}, keywords = {Economics; Economic activities; Economic framework; Epidemiological modeling; Health-care system; Mitigation strategy; Non-pharmaceutical interventions; Nonlinear model predictive control; Optimal controls; Model predictive control}, type = {Article}, publication_stage = {Final}, source = {Scopus}, note = {Cited by: 76; All Open Access, Green Open Access} }
- Scarabaggio, P., Carli, R., Cavone, G. & Dotoli, M. (2020) Smart control strategies for primary frequency regulation through electric vehicles: A battery degradation perspective. IN Energies, 13.. doi:10.3390/en13174586
[BibTeX] [Abstract] [Download PDF]Nowadays, due to the decreasing use of traditional generators in favor of renewable energy sources, power grids are facing a reduction of system inertia and primary frequency regulation capability. Such an issue is exacerbated by the continuously increasing number of electric vehicles (EVs), which results in enforcing novel approaches in the grid operations management. However, from being an issue, the increase of EVs may turn to be a solution to several power system challenges. In this context, a crucial role is played by the so-called vehicle-to-grid (V2G) mode of operation, which has the potential to provide ancillary services to the power grid, such as peak clipping, load shifting, and frequency regulation. More in detail, EVs have recently started to be effectively used for one of the most traditional frequency regulation approaches: the so-called frequency droop control (FDC). This is a primary frequency regulation, currently obtained by adjusting the active power of generators in the main grid. Because to the decommissioning of traditional power plants, EVs are thus recognized as particularly valuable solutions since they can respond to frequency deviation signals by charging or discharging their batteries. Against this background, we address frequency regulation of a power grid model including loads, traditional generators, and several EVs. The latter independently participate in the grid optimization process providing the grid with ancillary services, namely the FDC. We propose two novel control strategies for the optimal control of the batteries of EVs during the frequency regulation service. On the one hand, the control strategies ensure re-balancing the power and stabilizing the frequency of the main grid. On the other hand, the approaches are able to satisfy different types of needs of EVs during the charging process. Differently from the related literature, where the EVs perspective is generally oriented to achieve the optimal charge level, the proposed approaches aim at minimizing the degradation of battery devices. Finally, the proposed strategies are compared with other state-of-the-art V2G control approaches. The results of numerical experiments using a realistic power grid model show the effectiveness of the proposed strategies under the actual operating conditions. © 2020 by the authors. Licensee MDPI, Basel, Switzerland. This article is an open access article distributed under the terms and conditions of the Creative Commons Attribution (CC BY) license (http://creativecommons.org/licenses/by/4.0/).
@ARTICLE{Scarabaggio2020, author = {Scarabaggio, Paolo and Carli, Raffaele and Cavone, Graziana and Dotoli, Mariagrazia}, title = {Smart control strategies for primary frequency regulation through electric vehicles: A battery degradation perspective}, year = {2020}, journal = {Energies}, volume = {13}, number = {17}, doi = {10.3390/en13174586}, url = {https://www.scopus.com/inward/record.uri?eid=2-s2.0-85090919511&doi=10.3390%2fen13174586&partnerID=40&md5=d7f07f0a819d149b5f1c143b707e731d}, abstract = {Nowadays, due to the decreasing use of traditional generators in favor of renewable energy sources, power grids are facing a reduction of system inertia and primary frequency regulation capability. Such an issue is exacerbated by the continuously increasing number of electric vehicles (EVs), which results in enforcing novel approaches in the grid operations management. However, from being an issue, the increase of EVs may turn to be a solution to several power system challenges. In this context, a crucial role is played by the so-called vehicle-to-grid (V2G) mode of operation, which has the potential to provide ancillary services to the power grid, such as peak clipping, load shifting, and frequency regulation. More in detail, EVs have recently started to be effectively used for one of the most traditional frequency regulation approaches: the so-called frequency droop control (FDC). This is a primary frequency regulation, currently obtained by adjusting the active power of generators in the main grid. Because to the decommissioning of traditional power plants, EVs are thus recognized as particularly valuable solutions since they can respond to frequency deviation signals by charging or discharging their batteries. Against this background, we address frequency regulation of a power grid model including loads, traditional generators, and several EVs. The latter independently participate in the grid optimization process providing the grid with ancillary services, namely the FDC. We propose two novel control strategies for the optimal control of the batteries of EVs during the frequency regulation service. On the one hand, the control strategies ensure re-balancing the power and stabilizing the frequency of the main grid. On the other hand, the approaches are able to satisfy different types of needs of EVs during the charging process. Differently from the related literature, where the EVs perspective is generally oriented to achieve the optimal charge level, the proposed approaches aim at minimizing the degradation of battery devices. Finally, the proposed strategies are compared with other state-of-the-art V2G control approaches. The results of numerical experiments using a realistic power grid model show the effectiveness of the proposed strategies under the actual operating conditions. © 2020 by the authors. Licensee MDPI, Basel, Switzerland. This article is an open access article distributed under the terms and conditions of the Creative Commons Attribution (CC BY) license (http://creativecommons.org/licenses/by/4.0/).}, author_keywords = {Electric vehicle batteries (EVBs); Electric vehicles (EVs); Frequency droop control (FDC); Vehicle-to-grid (V2G)}, keywords = {Automotive batteries; Charging (batteries); Electric control equipment; Electric generators; Electric network topology; Electric power transmission networks; Electric vehicles; Microgrids; Renewable energy resources; Vehicle-to-grid; Electric Vehicles (EVs); Frequency regulation services; Frequency regulations; Numerical experiments; Primary frequency regulation; Renewable energy source; Smart control strategies; Vehicle to Grid (V2G); Electric power system control}, type = {Article}, publication_stage = {Final}, source = {Scopus}, note = {Cited by: 37; All Open Access, Gold Open Access, Green Open Access} }
- Carli, R., Digiesi, S., Dotoli, M. & Facchini, F. (2020) A control strategy for smart energy charging of warehouse material handling equipment IN Procedia Manufacturing., 503 – 510. doi:10.1016/j.promfg.2020.02.041
[BibTeX] [Abstract] [Download PDF]The common driver of the ‘green-warehouse’ strategy is based on the reduction of energy consumption. In warehouses with ‘picker-to-part’ operations the minimization of energy due to material handling activities can be achieved by means of different policies: by adopting smart automatic picking systems, by adopting energy-efficient material handling equipment (MHE) as well as by identifying flexible layouts. In most cases, these strategies require investments characterized by high pay-back times. In this context, management strategies focused on the adoption of available equipment allow to increase the warehouse productivity at negligible costs. With this purpose, an optimization model is proposed in order to identify an optimal control strategy for the battery charging of a fleet of electric mobile MHE (e.g., forklifts), allowing minimizing the economic and environmental impact of material handling activities in labor-intensive warehouses. The resulting scheduling problem is formalized as an integer programming (IP) problem aimed at minimizing the total cost, which is the sum of the penalty cost related to makespan over all the material handling activities and the total electricity cost for charging batteries of MHE. Numerical experiments are used to investigate and quantify the effects of integrating the scheduling of electric loads into the scheduling of material handling operations. © 2020 The Authors. Published by Elsevier B.V. This is an open access article under the CC BY-NC-ND license (http://creativecommons.org/licenses/by-nc-nd/4.0/) Peer-review under responsibility of the scientific committee of the International Conference on Industry 4.0 and Smart Manufacturing.
@CONFERENCE{Carli2020503, author = {Carli, Raffaele and Digiesi, Salvatore and Dotoli, Mariagrazia and Facchini, Francesco}, title = {A control strategy for smart energy charging of warehouse material handling equipment}, year = {2020}, journal = {Procedia Manufacturing}, volume = {42}, pages = {503 – 510}, doi = {10.1016/j.promfg.2020.02.041}, url = {https://www.scopus.com/inward/record.uri?eid=2-s2.0-85084243764&doi=10.1016%2fj.promfg.2020.02.041&partnerID=40&md5=795bb61103f666156bed4ba693a6503f}, abstract = {The common driver of the 'green-warehouse' strategy is based on the reduction of energy consumption. In warehouses with 'picker-to-part' operations the minimization of energy due to material handling activities can be achieved by means of different policies: by adopting smart automatic picking systems, by adopting energy-efficient material handling equipment (MHE) as well as by identifying flexible layouts. In most cases, these strategies require investments characterized by high pay-back times. In this context, management strategies focused on the adoption of available equipment allow to increase the warehouse productivity at negligible costs. With this purpose, an optimization model is proposed in order to identify an optimal control strategy for the battery charging of a fleet of electric mobile MHE (e.g., forklifts), allowing minimizing the economic and environmental impact of material handling activities in labor-intensive warehouses. The resulting scheduling problem is formalized as an integer programming (IP) problem aimed at minimizing the total cost, which is the sum of the penalty cost related to makespan over all the material handling activities and the total electricity cost for charging batteries of MHE. Numerical experiments are used to investigate and quantify the effects of integrating the scheduling of electric loads into the scheduling of material handling operations. © 2020 The Authors. Published by Elsevier B.V. This is an open access article under the CC BY-NC-ND license (http://creativecommons.org/licenses/by-nc-nd/4.0/) Peer-review under responsibility of the scientific committee of the International Conference on Industry 4.0 and Smart Manufacturing.}, author_keywords = {Battery smart charging; Green warehouse; Industrial/manufacturing demand side management; Integer programming; Material handling activity; Optimization; Warehouse energy management}, type = {Conference paper}, publication_stage = {Final}, source = {Scopus}, note = {Cited by: 17; All Open Access, Gold Open Access, Green Open Access} }
- Hosseini, S. M., Carli, R., Parisio, A. & Dotoli, M. (2020) Robust Decentralized Charge Control of Electric Vehicles under Uncertainty on Inelastic Demand and Energy Pricing IN Conference Proceedings – IEEE International Conference on Systems, Man and Cybernetics., 1834 – 1839. doi:10.1109/SMC42975.2020.9283440
[BibTeX] [Abstract] [Download PDF]This paper proposes a novel robust decentralized charging strategy for large-scale EV fleets. The system incorporates multiple EVs as well as inelastic loads connected to the power grid under power flow limits. We aim at minimizing both the overall charging energy payment and the aggregated battery degradation cost of EVs while preserving the robustness of the solution against uncertainties in the price of the electricity purchased from the power grid and the demand of inelastic loads. The proposed approach relies on the so-called uncertainty set-based robust optimization. The resulting charge scheduling problem is formulated as a tractable quadratic programming problem where all the EVs’ decisions are coupled via the grid resource-sharing constraints and the robust counterpart supporting constraints. We adopt an extended Jacobi-Proximal Alternating Direction Method of Multipliers algorithm to solve effectively the formulated scheduling problem in a decentralized fashion, thus allowing the method applicability to large scale fleets. Simulations of a realistic case study show that the proposed approach not only reduces the costs of the EV fleet, but also maintains the robustness of the solution against perturbations in different uncertain parameters, which is beneficial for both EVs’ users and the power grid. © 2020 IEEE.
@CONFERENCE{Hosseini20201834, author = {Hosseini, Seyed Mohsen and Carli, Raffaele and Parisio, Alessandra and Dotoli, Mariagrazia}, title = {Robust Decentralized Charge Control of Electric Vehicles under Uncertainty on Inelastic Demand and Energy Pricing}, year = {2020}, journal = {Conference Proceedings - IEEE International Conference on Systems, Man and Cybernetics}, volume = {2020-October}, pages = {1834 – 1839}, doi = {10.1109/SMC42975.2020.9283440}, url = {https://www.scopus.com/inward/record.uri?eid=2-s2.0-85098877625&doi=10.1109%2fSMC42975.2020.9283440&partnerID=40&md5=51febb550a1cfd7909fd0ff26527f5b0}, abstract = {This paper proposes a novel robust decentralized charging strategy for large-scale EV fleets. The system incorporates multiple EVs as well as inelastic loads connected to the power grid under power flow limits. We aim at minimizing both the overall charging energy payment and the aggregated battery degradation cost of EVs while preserving the robustness of the solution against uncertainties in the price of the electricity purchased from the power grid and the demand of inelastic loads. The proposed approach relies on the so-called uncertainty set-based robust optimization. The resulting charge scheduling problem is formulated as a tractable quadratic programming problem where all the EVs' decisions are coupled via the grid resource-sharing constraints and the robust counterpart supporting constraints. We adopt an extended Jacobi-Proximal Alternating Direction Method of Multipliers algorithm to solve effectively the formulated scheduling problem in a decentralized fashion, thus allowing the method applicability to large scale fleets. Simulations of a realistic case study show that the proposed approach not only reduces the costs of the EV fleet, but also maintains the robustness of the solution against perturbations in different uncertain parameters, which is beneficial for both EVs' users and the power grid. © 2020 IEEE.}, author_keywords = {ADMM; Charge scheduling; Decentralized control; Electric vehicles; Large-scale optimization; Robust optimization; Set-based uncertainty}, keywords = {Charging (batteries); Costs; Electric load flow; Quadratic programming; Scheduling; Uncertainty analysis; Alternating direction method of multipliers; Battery degradation; Charging energies; Charging strategies; Quadratic programming problems; Robust optimization; Scheduling problem; Uncertain parameters; Electric power transmission networks}, type = {Conference paper}, publication_stage = {Final}, source = {Scopus}, note = {Cited by: 11} }
- Scarabaggio, P., Carli, R., La Scala, M. & Dotoli, M. (2020) Effects of COVID-19 on electricity demand in Northern Italy; [Effetti del COVID-19 sulla domanda di energia elettrica nel Nord Italia]. IN Energia Elettrica, 97.41 – 51.
[BibTeX] [Abstract] [Download PDF]Technical analysis of the effects of the COVID-19 pandemic on electricity demand: the case of Northern Italy. Estimation of the impact of social mobility on electricity consumption. Future challenges and prospects.
@ARTICLE{Scarabaggio202041, author = {Scarabaggio, Paolo and Carli, Raffaele and La Scala, Massimo and Dotoli, Mariagrazia}, title = {Effects of COVID-19 on electricity demand in Northern Italy; [Effetti del COVID-19 sulla domanda di energia elettrica nel Nord Italia]}, year = {2020}, journal = {Energia Elettrica}, volume = {97}, number = {5}, pages = {41 – 51}, url = {https://www.scopus.com/inward/record.uri?eid=2-s2.0-85148942790&partnerID=40&md5=a3679ff0d5aca129d11834c5886fe935}, abstract = {Technical analysis of the effects of the COVID-19 pandemic on electricity demand: the case of Northern Italy. Estimation of the impact of social mobility on electricity consumption. Future challenges and prospects.}, type = {Article}, publication_stage = {Final}, source = {Scopus}, note = {Cited by: 0} }
- Carli, R. & Dotoli, M. (2020) Distributed Alternating Direction Method of Multipliers for Linearly Constrained Optimization over a Network. IN IEEE Control Systems Letters, 4.247 – 252. doi:10.1109/LCSYS.2019.2923078
[BibTeX] [Abstract] [Download PDF]In this letter we address the distributed optimization problem for a network of agents, which commonly occurs in several control engineering applications. Differently from the related literature, where only consensus constraints are typically addressed, we consider a challenging distributed optimization set-up where agents rely on local communication and computation to optimize a sum of local objective functions, each depending on individual variables subject to local constraints, while satisfying linear coupling constraints. Thanks to the distributed scheme, the resolution of the optimization problem turns into designing an iterative control procedure that steers the strategies of agents-whose dynamics is decoupled-not only to be convergent to the optimal value but also to satisfy the coupling constraints. Based on duality and consensus theory, we develop a proximal Jacobian alternating direction method of multipliers (ADMM) for solving such a kind of linearly constrained convex optimization problems over a network. Using the monotone operator and fixed point mapping, we analyze the optimality of the proposed algorithm and establish its o(1/t) convergence rate. Finally, through numerical simulations we show that the proposed algorithm offers higher computational performances than recent distributed ADMM variants. © 2019 IEEE.
@ARTICLE{Carli2020247, author = {Carli, Raffaele and Dotoli, Mariagrazia}, title = {Distributed Alternating Direction Method of Multipliers for Linearly Constrained Optimization over a Network}, year = {2020}, journal = {IEEE Control Systems Letters}, volume = {4}, number = {1}, pages = {247 – 252}, doi = {10.1109/LCSYS.2019.2923078}, url = {https://www.scopus.com/inward/record.uri?eid=2-s2.0-85068640431&doi=10.1109%2fLCSYS.2019.2923078&partnerID=40&md5=7f07a37d7f737276c9983542771cba08}, abstract = {In this letter we address the distributed optimization problem for a network of agents, which commonly occurs in several control engineering applications. Differently from the related literature, where only consensus constraints are typically addressed, we consider a challenging distributed optimization set-up where agents rely on local communication and computation to optimize a sum of local objective functions, each depending on individual variables subject to local constraints, while satisfying linear coupling constraints. Thanks to the distributed scheme, the resolution of the optimization problem turns into designing an iterative control procedure that steers the strategies of agents-whose dynamics is decoupled-not only to be convergent to the optimal value but also to satisfy the coupling constraints. Based on duality and consensus theory, we develop a proximal Jacobian alternating direction method of multipliers (ADMM) for solving such a kind of linearly constrained convex optimization problems over a network. Using the monotone operator and fixed point mapping, we analyze the optimality of the proposed algorithm and establish its o(1/t) convergence rate. Finally, through numerical simulations we show that the proposed algorithm offers higher computational performances than recent distributed ADMM variants. © 2019 IEEE.}, author_keywords = {Distributed control; distributed optimization; optimization algorithms}, keywords = {Computation theory; Convex optimization; Iterative methods; Alternating direction method of multipliers; Computational performance; Constrained convex optimizations; Distributed control; Distributed optimization; Engineering applications; Linearly constrained optimization; Optimization algorithms; Constrained optimization}, type = {Article}, publication_stage = {Final}, source = {Scopus}, note = {Cited by: 21} }
- Carli, R. & Dotoli, M. (2020) A Dynamic Programming Approach for the Decentralized Control of Energy Retrofit in Large-Scale Street Lighting Systems. IN IEEE Transactions on Automation Science and Engineering, 17.1140 – 1157. doi:10.1109/TASE.2020.2966738
[BibTeX] [Abstract] [Download PDF]This article proposes a decision-making procedure that supports the city energy manager in determining the optimal energy retrofit plan of an existing public street lighting system throughout a wide urban area. The proposed decision model aims at simultaneously maximizing the energy consumption reduction and achieving an optimal allocation of the retrofit actions among the street lighting subsystems, while efficiently using the available budget. The resulting optimization problem is formulated as a quadratic knapsack problem. The proposed solution relies on a decentralized control algorithm that combines discrete dynamic programming with additive decomposition and value functions approximation. The optimality and complexity of the presented strategy are investigated, demonstrating that the proposed algorithm constitutes a fully polynomial approximation scheme. Simulation results related to a real street lighting system in the city of Bari (Italy) are presented to show the effectiveness of the approach in the optimal energy management of large-scale street lighting systems. Note to Practitioners-This article addresses the emerging need for decision support tools for the energy management of urban street lighting systems. The proposed decision-making strategy allows city energy managers and local policy makers taking retrofit decisions on an existing public street lighting system throughout a wide urban area. The presented strategy can be implemented in any engineering software, providing decision makers with a low-complexity and scalable Information and Communication Technology (ICT) tool for the optimization of the energy efficiency and environmental sustainability of street lighting systems. © 2004-2012 IEEE.
@ARTICLE{Carli20201140, author = {Carli, Raffaele and Dotoli, Mariagrazia}, title = {A Dynamic Programming Approach for the Decentralized Control of Energy Retrofit in Large-Scale Street Lighting Systems}, year = {2020}, journal = {IEEE Transactions on Automation Science and Engineering}, volume = {17}, number = {3}, pages = {1140 – 1157}, doi = {10.1109/TASE.2020.2966738}, url = {https://www.scopus.com/inward/record.uri?eid=2-s2.0-85087543588&doi=10.1109%2fTASE.2020.2966738&partnerID=40&md5=b2d2ba1ad644c81f451c0936fe963e18}, abstract = {This article proposes a decision-making procedure that supports the city energy manager in determining the optimal energy retrofit plan of an existing public street lighting system throughout a wide urban area. The proposed decision model aims at simultaneously maximizing the energy consumption reduction and achieving an optimal allocation of the retrofit actions among the street lighting subsystems, while efficiently using the available budget. The resulting optimization problem is formulated as a quadratic knapsack problem. The proposed solution relies on a decentralized control algorithm that combines discrete dynamic programming with additive decomposition and value functions approximation. The optimality and complexity of the presented strategy are investigated, demonstrating that the proposed algorithm constitutes a fully polynomial approximation scheme. Simulation results related to a real street lighting system in the city of Bari (Italy) are presented to show the effectiveness of the approach in the optimal energy management of large-scale street lighting systems. Note to Practitioners-This article addresses the emerging need for decision support tools for the energy management of urban street lighting systems. The proposed decision-making strategy allows city energy managers and local policy makers taking retrofit decisions on an existing public street lighting system throughout a wide urban area. The presented strategy can be implemented in any engineering software, providing decision makers with a low-complexity and scalable Information and Communication Technology (ICT) tool for the optimization of the energy efficiency and environmental sustainability of street lighting systems. © 2004-2012 IEEE.}, author_keywords = {Decision-making; dynamic programming; energy management; fully polynomial approximation scheme; optimization; urban street lighting}, keywords = {Approximation algorithms; Budget control; Combinatorial optimization; Decentralized control; Decision making; Decision support systems; Energy efficiency; Energy management; Energy management systems; Energy utilization; Green computing; Lighting fixtures; Managers; Polynomial approximation; Retrofitting; Street lighting; Sustainable development; Additive decomposition; Decision making procedure; Decision support tools; Decision-making strategies; Environmental sustainability; Information and Communication Technologies; Quadratic knapsack problems; Street lighting system; Dynamic programming}, type = {Article}, publication_stage = {Final}, source = {Scopus}, note = {Cited by: 38} }
- Hosseini, S. M., Carli, R., Cavone, G. & Dotoli, M. (2020) Distributed control of electric vehicle fleets considering grid congestion and battery degradation. IN Internet Technology Letters, 3.. doi:10.1002/itl2.161
[BibTeX] [Abstract] [Download PDF]Nowadays, developing coordinated optimal charging strategies for large-scale electric vehicle (EV) fleets is crucial to ensure the reliability and efficiency of power grids. This paper presents a novel fully distributed control strategy for the optimal charging of large-scale EV fleets aiming at the minimization of the aggregated charging cost and battery degradation, while satisfying the EVs’ individual load requirements and the overall grid congestion limits. We formulate the optimization problem as a convex quadratic programming problem where all the EVs’ decisions are coupled both via the objective function and some grid resource sharing constraints. Based on the distributed waterfilling approach, the proposed resolution algorithm requires a minimal shared information between EVs that communicate only with their neighbors without relying on a central aggregator, thus guaranteeing the EV users’ privacy. The performance of the proposed approach is evaluated through numerical experiments to validate its effectiveness in achieving a global optimum while respecting the grid constraints with a favorable computational efficiency. © 2020 John Wiley & Sons, Ltd.
@ARTICLE{Hosseini2020, author = {Hosseini, Seyed M. and Carli, Raffaele and Cavone, Graziana and Dotoli, Mariagrazia}, title = {Distributed control of electric vehicle fleets considering grid congestion and battery degradation}, year = {2020}, journal = {Internet Technology Letters}, volume = {3}, number = {3}, doi = {10.1002/itl2.161}, url = {https://www.scopus.com/inward/record.uri?eid=2-s2.0-85087647927&doi=10.1002%2fitl2.161&partnerID=40&md5=a8266a663395bc58f3c3631dc8791c0a}, abstract = {Nowadays, developing coordinated optimal charging strategies for large-scale electric vehicle (EV) fleets is crucial to ensure the reliability and efficiency of power grids. This paper presents a novel fully distributed control strategy for the optimal charging of large-scale EV fleets aiming at the minimization of the aggregated charging cost and battery degradation, while satisfying the EVs' individual load requirements and the overall grid congestion limits. We formulate the optimization problem as a convex quadratic programming problem where all the EVs' decisions are coupled both via the objective function and some grid resource sharing constraints. Based on the distributed waterfilling approach, the proposed resolution algorithm requires a minimal shared information between EVs that communicate only with their neighbors without relying on a central aggregator, thus guaranteeing the EV users' privacy. The performance of the proposed approach is evaluated through numerical experiments to validate its effectiveness in achieving a global optimum while respecting the grid constraints with a favorable computational efficiency. © 2020 John Wiley & Sons, Ltd.}, author_keywords = {distributed control; electric vehicles; large-scale optimization; smart charging}, keywords = {Charging (batteries); Computational efficiency; Electric machine control; Electric power transmission networks; Quadratic programming; Secondary batteries; Traffic congestion; Vehicle-to-grid; Battery degradation; Charging strategies; Distributed control strategy; Distributed-control; Large-scale optimization; Large-scales; Optimal charging; Power grids; Smart charging; Vehicle fleets; Electric vehicles}, type = {Letter}, publication_stage = {Final}, source = {Scopus}, note = {Cited by: 13} }
- Scarabaggio, P., Grammatico, S., Carli, R. & Dotoli, M. (2020) A distributed, rolling-horizon demand side management algorithm under wind power uncertainty IN IFAC-PapersOnLine., 12620 – 12625. doi:10.1016/j.ifacol.2020.12.1830
[BibTeX] [Abstract] [Download PDF]In this paper, we consider a smart grid where users behave selfishly, aiming at minimizing cost in the presence of uncertain wind power availability. We adopt a demand side management (DSM) model, where active users (so-called prosumers) have both private generation and local storage availability. These prosumers participate to the DSM strategy by updating their energy schedule, seeking to minimize their local cost, given their local preferences and the global grid constraints. The energy price is defined as a function of the aggregate load and the wind power availability. We model the resulting problem as a non-cooperative Nash game and propose a semi-decentralized algorithm to compute an equilibrium. To cope with the uncertainty in the wind power, we adopt a rolling-horizon approach, and in addition we use a stochastic optimization technique. We generate several wind power production scenarios from a defined probability density function (PDF), determining an approximate stochastic cost function. Simulations results on a real dataset show that the proposed approach generates lower individual costs compared to a standard expected value approach. Copyright © 2020 The Authors. This is an open access article under the CC BY-NC-ND license
@CONFERENCE{Scarabaggio202012620, author = {Scarabaggio, Paolo and Grammatico, Sergio and Carli, Raffaele and Dotoli, Mariagrazia}, title = {A distributed, rolling-horizon demand side management algorithm under wind power uncertainty}, year = {2020}, journal = {IFAC-PapersOnLine}, volume = {53}, number = {2}, pages = {12620 – 12625}, doi = {10.1016/j.ifacol.2020.12.1830}, url = {https://www.scopus.com/inward/record.uri?eid=2-s2.0-85105077171&doi=10.1016%2fj.ifacol.2020.12.1830&partnerID=40&md5=ee3d9dd2c0187078aed713ccc87a026b}, abstract = {In this paper, we consider a smart grid where users behave selfishly, aiming at minimizing cost in the presence of uncertain wind power availability. We adopt a demand side management (DSM) model, where active users (so-called prosumers) have both private generation and local storage availability. These prosumers participate to the DSM strategy by updating their energy schedule, seeking to minimize their local cost, given their local preferences and the global grid constraints. The energy price is defined as a function of the aggregate load and the wind power availability. We model the resulting problem as a non-cooperative Nash game and propose a semi-decentralized algorithm to compute an equilibrium. To cope with the uncertainty in the wind power, we adopt a rolling-horizon approach, and in addition we use a stochastic optimization technique. We generate several wind power production scenarios from a defined probability density function (PDF), determining an approximate stochastic cost function. Simulations results on a real dataset show that the proposed approach generates lower individual costs compared to a standard expected value approach. Copyright © 2020 The Authors. This is an open access article under the CC BY-NC-ND license}, author_keywords = {Demand side management; Sample average approximation; Smart grid; Stochastic optimization}, keywords = {Approximation algorithms; Cost functions; Demand side management; Electric power transmission networks; Electric utilities; Optimization; Probability density function; Stochastic systems; Wind power; Energy; Management Model; Management strategies; Minimizing costs; Rolling horizon; Sample average approximation; Smart grid; Stochastic optimizations; Uncertainty; Wind power availability; Smart power grids}, type = {Conference paper}, publication_stage = {Final}, source = {Scopus}, note = {Cited by: 3; All Open Access, Gold Open Access} }
- Scarabaggio, P., La Scala, M., Carli, R. & Dotoli, M. (2020) Analyzing the Effects of COVID-19 Pandemic on the Energy Demand: The Case of Northern Italy IN 12th AEIT International Annual Conference, AEIT 2020.. doi:10.23919/AEIT50178.2020.9241136
[BibTeX] [Abstract] [Download PDF]The COVID-19 crisis is profoundly influencing the global economic framework due to restrictive measures adopted by governments worldwide. Finding real-time data to correctly quantify this impact is very significant but not as straightforward. Nevertheless, an analysis of the power demand profiles provides insight into the overall economic trends. To accurately assess the change in energy consumption patterns, in this work we employ a multi-layer feed-forward neural network that calculates an estimation of the aggregated power demand in the north of Italy, (i.e, in one of the European areas that were most affected by the pandemics) in the absence of the COVID-19 emergency. After assessing the forecasting model reliability, we compare the estimation with the ground truth data to quantify the variation in power consumption. Moreover, we correlate this variation with the change in mobility behaviors during the lockdown period by employing the Google mobility report data. From this unexpected and unprecedented situation, we obtain some intuition regarding the power system macro-structure and its relation with the overall people’s mobility. © 2020 AEIT.
@CONFERENCE{Scarabaggio2020, author = {Scarabaggio, Paolo and La Scala, Massimo and Carli, Raffaele and Dotoli, Mariagrazia}, title = {Analyzing the Effects of COVID-19 Pandemic on the Energy Demand: The Case of Northern Italy}, year = {2020}, journal = {12th AEIT International Annual Conference, AEIT 2020}, doi = {10.23919/AEIT50178.2020.9241136}, url = {https://www.scopus.com/inward/record.uri?eid=2-s2.0-85097170993&doi=10.23919%2fAEIT50178.2020.9241136&partnerID=40&md5=a3abf95802bb66dc9a2d8715976cc126}, abstract = {The COVID-19 crisis is profoundly influencing the global economic framework due to restrictive measures adopted by governments worldwide. Finding real-time data to correctly quantify this impact is very significant but not as straightforward. Nevertheless, an analysis of the power demand profiles provides insight into the overall economic trends. To accurately assess the change in energy consumption patterns, in this work we employ a multi-layer feed-forward neural network that calculates an estimation of the aggregated power demand in the north of Italy, (i.e, in one of the European areas that were most affected by the pandemics) in the absence of the COVID-19 emergency. After assessing the forecasting model reliability, we compare the estimation with the ground truth data to quantify the variation in power consumption. Moreover, we correlate this variation with the change in mobility behaviors during the lockdown period by employing the Google mobility report data. From this unexpected and unprecedented situation, we obtain some intuition regarding the power system macro-structure and its relation with the overall people's mobility. © 2020 AEIT.}, author_keywords = {COVID-19; Lockdown; Machine learning; Neural networks; Power systems}, keywords = {Electric power utilization; Energy utilization; Feedforward neural networks; Economic trends; Forecasting modeling; Global economics; Ground truth data; Macrostructures; Mobility behavior; Multilayer feedforward neural networks; Northern Italy; Multilayer neural networks}, type = {Conference paper}, publication_stage = {Final}, source = {Scopus}, note = {Cited by: 14; All Open Access, Green Open Access} }
- Carli, R., Cavone, G., Othman, S. B. & Dotoli, M. (2020) IoT based architecture for model predictive control of HVAC systems in smart buildings. IN Sensors (Switzerland), 20.. doi:10.3390/s20030781
[BibTeX] [Abstract] [Download PDF]The efficient management of Heating Ventilation and Air Conditioning (HVAC) systems in smart buildings is one of the main applications of the Internet of Things (IoT) paradigm. In this paper we propose an IoT based architecture for the implementation of Model Predictive Control (MPC) of HVAC systems in real environments. The considered MPC algorithm optimizes on line, in a closed-loop control fashion, both the indoor thermal comfort and the related energy consumption for a single zone environment. Thanks to the proposed IoT based architecture, the sensing, control, and actuating subsystems are all connected to the Internet, and a remote interface with the HVAC control system is guaranteed to end-users. In particular, sensors and actuators communicate with a remote database server and a control unit, which provides the control actions to be actuated in the HVAC system; users can set remotely the control mode and related set-points of the system; while comfort and environmental indices are transferred via the Internet and displayed on the end-users’ interface. The proposed IoT based control architecture is implemented and tested in a campus building at the Polytechnic of Bari (Italy) in a proof of concept perspective. The effectiveness of the proposed control algorithm is assessed in the real environment evaluating both the thermal comfort results and the energy savings with respect to a classical thermostat regulation approach. © 2020 by the authors. Licensee MDPI, Basel, Switzerland.
@ARTICLE{Carli2020, author = {Carli, Raffaele and Cavone, Graziana and Othman, Sarah Ben and Dotoli, Mariagrazia}, title = {IoT based architecture for model predictive control of HVAC systems in smart buildings}, year = {2020}, journal = {Sensors (Switzerland)}, volume = {20}, number = {3}, doi = {10.3390/s20030781}, url = {https://www.scopus.com/inward/record.uri?eid=2-s2.0-85079071499&doi=10.3390%2fs20030781&partnerID=40&md5=b68287ad61a3091865fc7546425dce95}, abstract = {The efficient management of Heating Ventilation and Air Conditioning (HVAC) systems in smart buildings is one of the main applications of the Internet of Things (IoT) paradigm. In this paper we propose an IoT based architecture for the implementation of Model Predictive Control (MPC) of HVAC systems in real environments. The considered MPC algorithm optimizes on line, in a closed-loop control fashion, both the indoor thermal comfort and the related energy consumption for a single zone environment. Thanks to the proposed IoT based architecture, the sensing, control, and actuating subsystems are all connected to the Internet, and a remote interface with the HVAC control system is guaranteed to end-users. In particular, sensors and actuators communicate with a remote database server and a control unit, which provides the control actions to be actuated in the HVAC system; users can set remotely the control mode and related set-points of the system; while comfort and environmental indices are transferred via the Internet and displayed on the end-users’ interface. The proposed IoT based control architecture is implemented and tested in a campus building at the Polytechnic of Bari (Italy) in a proof of concept perspective. The effectiveness of the proposed control algorithm is assessed in the real environment evaluating both the thermal comfort results and the energy savings with respect to a classical thermostat regulation approach. © 2020 by the authors. Licensee MDPI, Basel, Switzerland.}, author_keywords = {Heating ventilation and air conditioning system; Internet of things; Model predictive control; Predicted mean vote; Smart buildings}, keywords = {Air conditioning; Closed loop control systems; Energy conservation; Energy utilization; Heat pump systems; HVAC; Intelligent buildings; Model predictive control; Predictive control systems; Thermal comfort; Closed-loop control; Control architecture; Efficient managements; Heating ventilation and air conditioning; Indoor thermal comfort; Internet of thing (IOT); Predicted mean vote; Sensors and actuators; Internet of things}, type = {Article}, publication_stage = {Final}, source = {Scopus}, note = {Cited by: 96; All Open Access, Gold Open Access} }
- Carli, R., Dotoli, M., Digiesi, S., Facchini, F. & Mossa, G. (2020) Sustainable scheduling of material handling activities in labor-intensive warehouses: A decision and control model. IN Sustainability (Switzerland), 12.. doi:10.3390/SU12083111
[BibTeX] [Abstract] [Download PDF]In recent years, the continuous increase of greenhouse gas emissions has led many companies to investigate the activities that have the greatest impact on the environment. Recent studies estimate that around 10% of worldwide CO2 emissions derive from logistical supply chains. The considerable amount of energy required for heating, cooling, and lighting as well as material handling equipment (MHE) in warehouses represents about 20% of the overall logistical costs. The reduction of warehouses’ energy consumption would thus lead to a significant benefit from an environmental point of view. In this context, sustainable strategies allowing the minimization of the cost of energy consumption due to MHE represent a new challenge in warehouse management. Consistent with this purpose, a two-step optimization model based on integer programming is developed in this paper to automatically identify an optimal schedule of the material handling activities of electric mobile MHEs (MMHEs) (i.e., forklifts) in labor-intensive warehouses from profit and sustainability perspectives. The resulting scheduling aims at minimizing the total cost, which is the sum of the penalty cost related to the makespan of the material handling activities and the total electricity cost of charging batteries. The approach ensures that jobs are executed in accordance with priority queuing and that the completion time of battery recharging is minimized. Realistic numerical experiments are conducted to evaluate the effects of integrating the scheduling of electric loads into the scheduling of material handling operations. The obtained results show the effectiveness of the model in identifying the optimal battery-charging schedule for a fleet of electric MMHEs from economic and environmental perspectives simultaneously. © 2020 by the authors.
@ARTICLE{Carli2020, author = {Carli, Raffaele and Dotoli, Mariagrazia and Digiesi, Salvatore and Facchini, Francesco and Mossa, Giorgio}, title = {Sustainable scheduling of material handling activities in labor-intensive warehouses: A decision and control model}, year = {2020}, journal = {Sustainability (Switzerland)}, volume = {12}, number = {8}, doi = {10.3390/SU12083111}, url = {https://www.scopus.com/inward/record.uri?eid=2-s2.0-85084607073&doi=10.3390%2fSU12083111&partnerID=40&md5=225791095c6b1ea49a9be39370e2fff5}, abstract = {In recent years, the continuous increase of greenhouse gas emissions has led many companies to investigate the activities that have the greatest impact on the environment. Recent studies estimate that around 10% of worldwide CO2 emissions derive from logistical supply chains. The considerable amount of energy required for heating, cooling, and lighting as well as material handling equipment (MHE) in warehouses represents about 20% of the overall logistical costs. The reduction of warehouses' energy consumption would thus lead to a significant benefit from an environmental point of view. In this context, sustainable strategies allowing the minimization of the cost of energy consumption due to MHE represent a new challenge in warehouse management. Consistent with this purpose, a two-step optimization model based on integer programming is developed in this paper to automatically identify an optimal schedule of the material handling activities of electric mobile MHEs (MMHEs) (i.e., forklifts) in labor-intensive warehouses from profit and sustainability perspectives. The resulting scheduling aims at minimizing the total cost, which is the sum of the penalty cost related to the makespan of the material handling activities and the total electricity cost of charging batteries. The approach ensures that jobs are executed in accordance with priority queuing and that the completion time of battery recharging is minimized. Realistic numerical experiments are conducted to evaluate the effects of integrating the scheduling of electric loads into the scheduling of material handling operations. The obtained results show the effectiveness of the model in identifying the optimal battery-charging schedule for a fleet of electric MMHEs from economic and environmental perspectives simultaneously. © 2020 by the authors.}, author_keywords = {Battery charging; Decision and control; Demand-side management; Green warehouse; Material handling activity; Mobile material handling equipment; Optimization; Sustainable scheduling; Warehouse energy management}, keywords = {carbon dioxide; carbon emission; cooling; cost analysis; decision making; environmental economics; environmental impact; equipment; fuel consumption; greenhouse gas; heating; optimization; strategic approach; supply chain management; sustainability}, type = {Article}, publication_stage = {Final}, source = {Scopus}, note = {Cited by: 27; All Open Access, Gold Open Access} }
- Scarabaggio, P., Carli, R. & Dotoli, M. (2020) A game-theoretic control approach for the optimal energy storage under power flow constraints in distribution networks IN IEEE International Conference on Automation Science and Engineering., 1281 – 1286. doi:10.1109/CASE48305.2020.9216800
[BibTeX] [Abstract] [Download PDF]Traditionally, the management of power distribution networks relies on the centralized implementation of the optimal power flow and, in particular, the minimization of the generation cost and transmission losses. Nevertheless, the increasing penetration of both renewable energy sources and independent players such as ancillary service providers in modern networks have made this centralized framework inadequate. Against this background, we propose a noncooperative game-theoretic framework for optimally controlling energy storage systems (ESSs) in power distribution networks. Specifically, in this paper we address a power grid model that comprehends traditional loads, distributed generation sources and several independent energy storage providers, each owning an individual ESS. Through a rolling-horizon approach, the latter participate in the grid optimization process, aiming both at increasing the penetration of distributed generation and leveling the power injection from the transmission grid. Our framework incorporates not only economic factors but also grid stability aspects, including the power flow constraints. The paper fully describes the distribution grid model as well as the underlying market hypotheses and policies needed to force the energy storage providers to find a feasible equilibrium for the network. Numerical experiments based on the IEEE 33-bus system confirm the effectiveness and resiliency of the proposed framework. © 2020 IEEE.
@CONFERENCE{Scarabaggio20201281, author = {Scarabaggio, Paolo and Carli, Raffaele and Dotoli, Mariagrazia}, title = {A game-theoretic control approach for the optimal energy storage under power flow constraints in distribution networks}, year = {2020}, journal = {IEEE International Conference on Automation Science and Engineering}, volume = {2020-August}, pages = {1281 – 1286}, doi = {10.1109/CASE48305.2020.9216800}, url = {https://www.scopus.com/inward/record.uri?eid=2-s2.0-85094128798&doi=10.1109%2fCASE48305.2020.9216800&partnerID=40&md5=e4802c482f96ac90afd6c7a2c7f2b199}, abstract = {Traditionally, the management of power distribution networks relies on the centralized implementation of the optimal power flow and, in particular, the minimization of the generation cost and transmission losses. Nevertheless, the increasing penetration of both renewable energy sources and independent players such as ancillary service providers in modern networks have made this centralized framework inadequate. Against this background, we propose a noncooperative game-theoretic framework for optimally controlling energy storage systems (ESSs) in power distribution networks. Specifically, in this paper we address a power grid model that comprehends traditional loads, distributed generation sources and several independent energy storage providers, each owning an individual ESS. Through a rolling-horizon approach, the latter participate in the grid optimization process, aiming both at increasing the penetration of distributed generation and leveling the power injection from the transmission grid. Our framework incorporates not only economic factors but also grid stability aspects, including the power flow constraints. The paper fully describes the distribution grid model as well as the underlying market hypotheses and policies needed to force the energy storage providers to find a feasible equilibrium for the network. Numerical experiments based on the IEEE 33-bus system confirm the effectiveness and resiliency of the proposed framework. © 2020 IEEE.}, keywords = {Data storage equipment; Distributed power generation; Electric load flow; Electric network analysis; Electric power system economics; Electric power transmission; Energy storage; Game theory; Renewable energy resources; Storage as a service (STaaS); Distributed generation source; Energy Storage Systems (ESSs); Noncooperative game; Numerical experiments; Optimal power flows; Power distribution network; Renewable energy source; Transmission grids; Electric power transmission networks}, type = {Conference paper}, publication_stage = {Final}, source = {Scopus}, note = {Cited by: 11} }
- Carli, R., Cavone, G., Epicoco, N., Di Ferdinando, M., Scarabaggio, P. & Dotoli, M. (2020) Consensus-Based Algorithms for Controlling Swarms of Unmanned Aerial Vehicles. IN Lecture Notes in Computer Science (including subseries Lecture Notes in Artificial Intelligence and Lecture Notes in Bioinformatics), 12338 LNCS.84 – 99. doi:10.1007/978-3-030-61746-2_7
[BibTeX] [Abstract] [Download PDF]Multiple Unmanned Aerial Vehicles (multi-UAVs) applications are recently growing in several fields, ranging from military and rescue missions, remote sensing, and environmental surveillance, to meteorology, logistics, and farming. Overcoming the limitations on battery lifespan and on-board processor capabilities, the coordinated use of multi-UAVs is indeed more suitable than employing a single UAV in certain tasks. Hence, the research on swarm of UAVs is receiving increasing attention, including multidisciplinary aspects, such as coordination, aggregation, network communication, path planning, information sensing, and data fusion. The focus of this paper is on defining novel control strategies for the deployment of multi-UAV systems in a distributed time-varying set-up, where UAVs rely on local communication and computation. In particular, modeling the dynamics of each UAV by a discrete-time integrator, we analyze the main swarm intelligence strategies, namely flight formation, swarm tracking, and social foraging. First, we define a distributed control strategy for steering the agents of the swarm towards a collection point. Then, we cope with the formation control, defining a procedure to arrange agents in a family of geometric formations, where the distance between each pair of UAVs is predefined. Subsequently, we focus on swarm tracking, defining a distributed mechanism based on the so-called leader-following consensus to move the entire swarm in accordance with a predefined trajectory. Moreover, we define a social foraging strategy that allows agents to avoid obstacles, by imposing on-line a time-varying formation pattern. Finally, through numerical simulations we show the effectiveness of the proposed algorithms. © 2020, Springer Nature Switzerland AG.
@ARTICLE{Carli202084, author = {Carli, Raffaele and Cavone, Graziana and Epicoco, Nicola and Di Ferdinando, Mario and Scarabaggio, Paolo and Dotoli, Mariagrazia}, title = {Consensus-Based Algorithms for Controlling Swarms of Unmanned Aerial Vehicles}, year = {2020}, journal = {Lecture Notes in Computer Science (including subseries Lecture Notes in Artificial Intelligence and Lecture Notes in Bioinformatics)}, volume = {12338 LNCS}, pages = {84 – 99}, doi = {10.1007/978-3-030-61746-2_7}, url = {https://www.scopus.com/inward/record.uri?eid=2-s2.0-85093852465&doi=10.1007%2f978-3-030-61746-2_7&partnerID=40&md5=1c7da6000e4015880227c1eafe608f20}, abstract = {Multiple Unmanned Aerial Vehicles (multi-UAVs) applications are recently growing in several fields, ranging from military and rescue missions, remote sensing, and environmental surveillance, to meteorology, logistics, and farming. Overcoming the limitations on battery lifespan and on-board processor capabilities, the coordinated use of multi-UAVs is indeed more suitable than employing a single UAV in certain tasks. Hence, the research on swarm of UAVs is receiving increasing attention, including multidisciplinary aspects, such as coordination, aggregation, network communication, path planning, information sensing, and data fusion. The focus of this paper is on defining novel control strategies for the deployment of multi-UAV systems in a distributed time-varying set-up, where UAVs rely on local communication and computation. In particular, modeling the dynamics of each UAV by a discrete-time integrator, we analyze the main swarm intelligence strategies, namely flight formation, swarm tracking, and social foraging. First, we define a distributed control strategy for steering the agents of the swarm towards a collection point. Then, we cope with the formation control, defining a procedure to arrange agents in a family of geometric formations, where the distance between each pair of UAVs is predefined. Subsequently, we focus on swarm tracking, defining a distributed mechanism based on the so-called leader-following consensus to move the entire swarm in accordance with a predefined trajectory. Moreover, we define a social foraging strategy that allows agents to avoid obstacles, by imposing on-line a time-varying formation pattern. Finally, through numerical simulations we show the effectiveness of the proposed algorithms. © 2020, Springer Nature Switzerland AG.}, author_keywords = {Swarm intelligence; Trajectory control; Unmanned Aerial Vehicles}, keywords = {Aircraft detection; Antennas; Data fusion; Distributed parameter control systems; Military applications; Military vehicles; Remote sensing; Unmanned aerial vehicles (UAV); Control strategies; Discrete-time integrators; Distributed control strategy; Environmental surveillance; Local communications; Network communications; Onboard processors; Time-varying formations; Mobile ad hoc networks}, type = {Conference paper}, publication_stage = {Final}, source = {Scopus}, note = {Cited by: 19} }
2019
- Hosseini, S. M., Carli, R. & Dotoli, M. (2019) Robust day-ahead energy scheduling of a smart residential user under uncertainty IN 2019 18th European Control Conference, ECC 2019., 935 – 940. doi:10.23919/ECC.2019.8796182
[BibTeX] [Abstract] [Download PDF]This paper develops a robust optimization framework for the day-ahead energy scheduling of a grid-connected residential user. The system incorporates a renewable energy source (RES), a battery energy storage system (BESS) as well as elastic controllable and critical noncontrollable electrical appliances. The proposed approach copes with the fluctuation and intermittence of the RES generation and non-controllable load demand by a tractable robust optimization scheme requiring minimum information on the sources of uncertainty. The main objective is minimizing the total energy payment for the user considering operational/technical constraints and a contractual constraint penalizing the excessive use of energy. The presented framework allows the decision maker to define different robustness levels for uncertain variables, and to flexibly establish an equilibrium between user’s payment and price of robustness. To validate the effectiveness of the proposed framework under uncertainty, we simulate the dynamics of a residential user as a case study. A comparison between the proposed robust approach and the same method with deterministic RES and loads profiles is carried out and discussed. © 2019 EUCA.
@CONFERENCE{Hosseini2019935, author = {Hosseini, Seyed Mohsen and Carli, Raffaele and Dotoli, Mariagrazia}, title = {Robust day-ahead energy scheduling of a smart residential user under uncertainty}, year = {2019}, journal = {2019 18th European Control Conference, ECC 2019}, pages = {935 – 940}, doi = {10.23919/ECC.2019.8796182}, url = {https://www.scopus.com/inward/record.uri?eid=2-s2.0-85071523023&doi=10.23919%2fECC.2019.8796182&partnerID=40&md5=dbaf920bef232ca07b714d294ec35e28}, abstract = {This paper develops a robust optimization framework for the day-ahead energy scheduling of a grid-connected residential user. The system incorporates a renewable energy source (RES), a battery energy storage system (BESS) as well as elastic controllable and critical noncontrollable electrical appliances. The proposed approach copes with the fluctuation and intermittence of the RES generation and non-controllable load demand by a tractable robust optimization scheme requiring minimum information on the sources of uncertainty. The main objective is minimizing the total energy payment for the user considering operational/technical constraints and a contractual constraint penalizing the excessive use of energy. The presented framework allows the decision maker to define different robustness levels for uncertain variables, and to flexibly establish an equilibrium between user's payment and price of robustness. To validate the effectiveness of the proposed framework under uncertainty, we simulate the dynamics of a residential user as a case study. A comparison between the proposed robust approach and the same method with deterministic RES and loads profiles is carried out and discussed. © 2019 EUCA.}, keywords = {Decision making; Optimization; Renewable energy resources; Robustness (control systems); Scheduling; Battery energy storage systems; Controllable loads; Electrical appliances; Minimum information; Renewable energy source; Robust optimization; Sources of uncertainty; Uncertain variables; Housing}, type = {Conference paper}, publication_stage = {Final}, source = {Scopus}, note = {Cited by: 31} }
- Hosseini, S. M., Carli, R. & Dotoli, M. (2019) A residential demand-side management strategy under nonlinear pricing based on robust model predictive control IN Conference Proceedings – IEEE International Conference on Systems, Man and Cybernetics., 3243 – 3248. doi:10.1109/SMC.2019.8913892
[BibTeX] [Abstract] [Download PDF]This paper presents a real-time demand side management framework based on robust model predictive control (RMPC) for residential smart grids. The system incorporates a number of interconnected smart homes, each equipped with controllable and non-controllable loads, as well as a shared energy storage system (ESS). We aim at minimizing the users’ energy payment and limiting the peak-to-average ratio (PAR) of the energy consumption while taking into account all device/comfort/contractual constraints, specifically the feasibility constraints on energy transferred between users and the power grid in presence of load demand uncertainty. We consider a quadratic cost function for energy bought from the grid. Firstly, the energy price and related constraints of the system are modeled. Then, a min-max robust problem is established to optimally schedule energy under an interval-based uncertainty set. We finally adopt model predictive control (MPC) to solve the resulting robust optimization problem iteratively over a finite-horizon time window based on the receding horizon concept. Moreover, the robustness of the proposed real-time approach against the level of conservativeness of the solution is addressed. The effectiveness of the method is validated through a simulated case study. © 2019 IEEE.
@CONFERENCE{Hosseini20193243, author = {Hosseini, Seyed Mohsen and Carli, Raffaele and Dotoli, Mariagrazia}, title = {A residential demand-side management strategy under nonlinear pricing based on robust model predictive control}, year = {2019}, journal = {Conference Proceedings - IEEE International Conference on Systems, Man and Cybernetics}, volume = {2019-October}, pages = {3243 – 3248}, doi = {10.1109/SMC.2019.8913892}, url = {https://www.scopus.com/inward/record.uri?eid=2-s2.0-85076744329&doi=10.1109%2fSMC.2019.8913892&partnerID=40&md5=b8658f66ca7439fa6e7d9b02705e1b91}, abstract = {This paper presents a real-time demand side management framework based on robust model predictive control (RMPC) for residential smart grids. The system incorporates a number of interconnected smart homes, each equipped with controllable and non-controllable loads, as well as a shared energy storage system (ESS). We aim at minimizing the users' energy payment and limiting the peak-to-average ratio (PAR) of the energy consumption while taking into account all device/comfort/contractual constraints, specifically the feasibility constraints on energy transferred between users and the power grid in presence of load demand uncertainty. We consider a quadratic cost function for energy bought from the grid. Firstly, the energy price and related constraints of the system are modeled. Then, a min-max robust problem is established to optimally schedule energy under an interval-based uncertainty set. We finally adopt model predictive control (MPC) to solve the resulting robust optimization problem iteratively over a finite-horizon time window based on the receding horizon concept. Moreover, the robustness of the proposed real-time approach against the level of conservativeness of the solution is addressed. The effectiveness of the method is validated through a simulated case study. © 2019 IEEE.}, keywords = {Automation; Cost functions; Costs; Demand side management; Electric power transmission networks; Electric utilities; Energy utilization; Housing; Intelligent buildings; Iterative methods; Optimization; Predictive control systems; Robust control; Controllable loads; Energy storage systems; Non-linear pricing; Peak to average ratios; Quadratic cost functions; Robust model predictive control; Robust model predictive controls (RMPC); Robust optimization; Model predictive control}, type = {Conference paper}, publication_stage = {Final}, source = {Scopus}, note = {Cited by: 32} }
- Carli, R., Dotoli, M. & Palmisano, V. (2019) A distributed control approach based on game theory for the optimal energy scheduling of a residential microgrid with shared generation and storage IN IEEE International Conference on Automation Science and Engineering., 960 – 965. doi:10.1109/COASE.2019.8843141
[BibTeX] [Abstract] [Download PDF]This paper presents a distributed control approach based on game theory for the energy scheduling of demand-side consumers sharing energy production and storage while purchasing further energy from the grid. The interaction between the controllers of consumers’ loads and the manager of shared energy resources is modeled as a two-level game. The competition among consumers is formulated as a noncooperative game, while the interaction between the consumers’ loads and the shared resources manager is formulated as a cooperative game. optimization problems are stated for each player to determine their own optimal strategies. The algorithms for loads controllers and shared resources’ manager are implemented through a distributed approach. Numerical experiments show the effectiveness of the proposed scheme. © 2019 IEEE.
@CONFERENCE{Carli2019960, author = {Carli, Raffaele and Dotoli, Mariagrazia and Palmisano, Vittorio}, title = {A distributed control approach based on game theory for the optimal energy scheduling of a residential microgrid with shared generation and storage}, year = {2019}, journal = {IEEE International Conference on Automation Science and Engineering}, volume = {2019-August}, pages = {960 – 965}, doi = {10.1109/COASE.2019.8843141}, url = {https://www.scopus.com/inward/record.uri?eid=2-s2.0-85072980491&doi=10.1109%2fCOASE.2019.8843141&partnerID=40&md5=52d9b8791bf4c74bacd4b6c99e48e8b9}, abstract = {This paper presents a distributed control approach based on game theory for the energy scheduling of demand-side consumers sharing energy production and storage while purchasing further energy from the grid. The interaction between the controllers of consumers' loads and the manager of shared energy resources is modeled as a two-level game. The competition among consumers is formulated as a noncooperative game, while the interaction between the consumers' loads and the shared resources manager is formulated as a cooperative game. optimization problems are stated for each player to determine their own optimal strategies. The algorithms for loads controllers and shared resources' manager are implemented through a distributed approach. Numerical experiments show the effectiveness of the proposed scheme. © 2019 IEEE.}, keywords = {Controllers; Energy resources; Managers; Scheduling; Distributed approaches; Distributed control; Energy productions; Noncooperative game; Numerical experiments; Optimal strategies; Optimization problems; Residential microgrid; Game theory}, type = {Conference paper}, publication_stage = {Final}, source = {Scopus}, note = {Cited by: 6} }
- Carli, R., Dotoli, M. & Pellegrino, R. (2019) A multi-period approach for the optimal energy retrofit planning of street lighting systems. IN Applied Sciences (Switzerland), 9.. doi:10.3390/app9051025
[BibTeX] [Abstract] [Download PDF]Investing in the optimal measures for improving the energy efficiency of urban street lighting systems has become strategic for the economic, technological and social development of cities. The decision-making process for the selection of the optimal set of interventions is not so straightforward. Several criticalities-such as difficulties getting access to credit for companies involved in street lighting systems refurbishment, budget constraints of municipalities, and unawareness of the actual energy and economic performance after a retrofitting intervention-require a decision-making approach that supports the city energy manager in selecting the optimal street lighting energy efficiency retrofitting solution while looking not only based on the available budget, but also based on the future savings in energy expenditures. In this context, the purpose of our research is to develop an effective decision-making model supporting the optimal multi-period planning of the street lighting energy efficiency retrofitting, which proves to be more effective and beneficial than the classical single-period approach and has never before been applied to the considered public lighting system context. The proposed methodology is applied to a real street lighting system in the city of Bari, Italy, showing the energy savings and financial benefit obtained through the proposed method. Numerical experiments are used to investigate and quantify the effects of using a multi-period planning approach instead of a single-period approach. © 2019 by the authors.
@ARTICLE{Carli2019, author = {Carli, Raffaele and Dotoli, Mariagrazia and Pellegrino, Roberta}, title = {A multi-period approach for the optimal energy retrofit planning of street lighting systems}, year = {2019}, journal = {Applied Sciences (Switzerland)}, volume = {9}, number = {5}, doi = {10.3390/app9051025}, url = {https://www.scopus.com/inward/record.uri?eid=2-s2.0-85063660288&doi=10.3390%2fapp9051025&partnerID=40&md5=80ca57851d94e4757320cee529983b5f}, abstract = {Investing in the optimal measures for improving the energy efficiency of urban street lighting systems has become strategic for the economic, technological and social development of cities. The decision-making process for the selection of the optimal set of interventions is not so straightforward. Several criticalities-such as difficulties getting access to credit for companies involved in street lighting systems refurbishment, budget constraints of municipalities, and unawareness of the actual energy and economic performance after a retrofitting intervention-require a decision-making approach that supports the city energy manager in selecting the optimal street lighting energy efficiency retrofitting solution while looking not only based on the available budget, but also based on the future savings in energy expenditures. In this context, the purpose of our research is to develop an effective decision-making model supporting the optimal multi-period planning of the street lighting energy efficiency retrofitting, which proves to be more effective and beneficial than the classical single-period approach and has never before been applied to the considered public lighting system context. The proposed methodology is applied to a real street lighting system in the city of Bari, Italy, showing the energy savings and financial benefit obtained through the proposed method. Numerical experiments are used to investigate and quantify the effects of using a multi-period planning approach instead of a single-period approach. © 2019 by the authors.}, author_keywords = {Energy efficiency management; Multi-period planning; Optimization; Street lighting}, type = {Article}, publication_stage = {Final}, source = {Scopus}, note = {Cited by: 11; All Open Access, Gold Open Access, Green Open Access} }
- Carli, R. & Dotoli, M. (2019) Decentralized control for residential energy management of a smart users’ microgrid with renewable energy exchange. IN IEEE/CAA Journal of Automatica Sinica, 6.641 – 656. doi:10.1109/JAS.2019.1911462
[BibTeX] [Abstract] [Download PDF]This paper presents a decentralized control strategy for the scheduling of electrical energy activities of a microgrid composed of smart homes connected to a distributor and exchanging renewable energy produced by individually owned distributed energy resources. The scheduling problem is stated and solved with the aim of reducing the overall energy supply from the grid, by allowing users to exchange the surplus renewable energy and by optimally planning users x02BC controllable loads. We assume that each smart home can both buy x002F sell energy from x002F to the grid taking into account time-varying non-linear pricing signals. Simultaneously, smart homes cooperate and may buy x002F sell locally harvested renewable energy from x002F to other smart homes. The resulting optimization problem is formulated as a non-convex non-linear programming problem with a coupling of decision variables in the constraints. The proposed solution is based on a novel heuristic iterative decentralized scheme algorithm that suitably extends the Alternating Direction Method of Multipliers to a non-convex and decentralized setting. We discuss the conditions that guarantee the convergence of the presented algorithm. Finally, the application of the proposed technique to a case study under several scenarios shows its effectiveness. © 2014 Chinese Association of Automation.
@ARTICLE{Carli2019641, author = {Carli, Raffaele and Dotoli, Mariagrazia}, title = {Decentralized control for residential energy management of a smart users’ microgrid with renewable energy exchange}, year = {2019}, journal = {IEEE/CAA Journal of Automatica Sinica}, volume = {6}, number = {3}, pages = {641 – 656}, doi = {10.1109/JAS.2019.1911462}, url = {https://www.scopus.com/inward/record.uri?eid=2-s2.0-85065583902&doi=10.1109%2fJAS.2019.1911462&partnerID=40&md5=dfb517c8f1147f9d27b6d1082a326908}, abstract = {This paper presents a decentralized control strategy for the scheduling of electrical energy activities of a microgrid composed of smart homes connected to a distributor and exchanging renewable energy produced by individually owned distributed energy resources. The scheduling problem is stated and solved with the aim of reducing the overall energy supply from the grid, by allowing users to exchange the surplus renewable energy and by optimally planning users x02BC controllable loads. We assume that each smart home can both buy x002F sell energy from x002F to the grid taking into account time-varying non-linear pricing signals. Simultaneously, smart homes cooperate and may buy x002F sell locally harvested renewable energy from x002F to other smart homes. The resulting optimization problem is formulated as a non-convex non-linear programming problem with a coupling of decision variables in the constraints. The proposed solution is based on a novel heuristic iterative decentralized scheme algorithm that suitably extends the Alternating Direction Method of Multipliers to a non-convex and decentralized setting. We discuss the conditions that guarantee the convergence of the presented algorithm. Finally, the application of the proposed technique to a case study under several scenarios shows its effectiveness. © 2014 Chinese Association of Automation.}, keywords = {Automation; Decentralized control; Energy resources; Heuristic methods; Intelligent buildings; Microgrids; Nonlinear programming; Scheduling; Alternating direction method of multipliers; Decision variables; Distributed Energy Resources; Nonlinear programming problem; Optimization problems; Renewable energies; Residential energy; Scheduling problem; Iterative methods}, type = {Article}, publication_stage = {Final}, source = {Scopus}, note = {Cited by: 80} }
- Carli, R., Cavone, G., Dotoli, M., Epicoco, N. & Scarabaggio, P. (2019) Model predictive control for thermal comfort optimization in building energy management systems IN Conference Proceedings – IEEE International Conference on Systems, Man and Cybernetics., 2608 – 2613. doi:10.1109/SMC.2019.8914489
[BibTeX] [Abstract] [Download PDF]Model Predictive Control (MPC) has recently gained special attention to efficiently regulate Heating, Ventilation and Air Conditioning (HVAC) systems of buildings, since it explicitly allows energy savings while maintaining thermal comfort criteria. In this paper we propose a MPC algorithm for the on-line optimization of both the indoor thermal comfort and the related energy consumption of buildings. We use Fanger’s Predicted Mean Vote (PMV) as thermal comfort index, while to predict the energy performance of the building, we adopt a simplified thermal model. This allows computing optimal control actions by defining and solving a tractable non-linear optimization problem that incorporates the PMV index into the MPC cost function in addition to a term accounting for energy saving. The proposed MPC approach is implemented on a building automation system deployed in an office building located at the Polytechnic of Bari (Italy). Several on-field tests are performed to assess the applicability and efficacy of the control algorithm in a real environment against classical thermal comfort control approach based on the use of thermostats. © 2019 IEEE.
@CONFERENCE{Carli20192608, author = {Carli, Raffaele and Cavone, Graziana and Dotoli, Mariagrazia and Epicoco, Nicola and Scarabaggio, Paolo}, title = {Model predictive control for thermal comfort optimization in building energy management systems}, year = {2019}, journal = {Conference Proceedings - IEEE International Conference on Systems, Man and Cybernetics}, volume = {2019-October}, pages = {2608 – 2613}, doi = {10.1109/SMC.2019.8914489}, url = {https://www.scopus.com/inward/record.uri?eid=2-s2.0-85076778873&doi=10.1109%2fSMC.2019.8914489&partnerID=40&md5=3c982fb93adbcfb5202b48b60ad0f22d}, abstract = {Model Predictive Control (MPC) has recently gained special attention to efficiently regulate Heating, Ventilation and Air Conditioning (HVAC) systems of buildings, since it explicitly allows energy savings while maintaining thermal comfort criteria. In this paper we propose a MPC algorithm for the on-line optimization of both the indoor thermal comfort and the related energy consumption of buildings. We use Fanger's Predicted Mean Vote (PMV) as thermal comfort index, while to predict the energy performance of the building, we adopt a simplified thermal model. This allows computing optimal control actions by defining and solving a tractable non-linear optimization problem that incorporates the PMV index into the MPC cost function in addition to a term accounting for energy saving. The proposed MPC approach is implemented on a building automation system deployed in an office building located at the Polytechnic of Bari (Italy). Several on-field tests are performed to assess the applicability and efficacy of the control algorithm in a real environment against classical thermal comfort control approach based on the use of thermostats. © 2019 IEEE.}, keywords = {Air conditioning; Automation; Cost functions; Energy conservation; Energy management systems; Energy utilization; Intelligent buildings; Nonlinear programming; Office buildings; Predictive control systems; Thermal comfort; Building automation systems; Energy performance; Indoor thermal comfort; Non-linear optimization problems; Online optimization; Predicted mean vote; Thermal comfort control; Thermal comfort index; Model predictive control}, type = {Conference paper}, publication_stage = {Final}, source = {Scopus}, note = {Cited by: 20} }
- Carli, R., Cavone, G., Dotoli, M., Epicoco, N., Manganiello, C. & Tricarico, L. (2019) ICT-based methodologies for sheet metal forming design: A survey on simulation approaches IN Conference Proceedings – IEEE International Conference on Systems, Man and Cybernetics., 128 – 133. doi:10.1109/SMC.2019.8914082
[BibTeX] [Abstract] [Download PDF]Sheet metal forming processes are widely adopted in manufacturing industries and in the recent years there has been a growing demand for sheet metal items with different shapes and characteristics. However, the traditional process is unable to meet the modern industrial requirements, mainly due to the high costs of dies and the long manufacturing time cycles. On the contrary, developing products with high speed, low cost, and high quality is a key issue. Therefore, new methods and technologies to speed up the sheet metal forming process while keeping costs limited are needed. In particular, a key issue is the proper design of the forming process, which can benefit from the use of Information and Communications Technology (ICT) simulation techniques. This paper investigates the recent trends on ICT-based methodologies for sheet metal forming to identify the foremost research areas whose advancement will lead meeting the modern market’s needs. © 2019 IEEE.
@CONFERENCE{Carli2019128, author = {Carli, Raffaele and Cavone, Graziana and Dotoli, Mariagrazia and Epicoco, Nicola and Manganiello, Claudio and Tricarico, Luigi}, title = {ICT-based methodologies for sheet metal forming design: A survey on simulation approaches}, year = {2019}, journal = {Conference Proceedings - IEEE International Conference on Systems, Man and Cybernetics}, volume = {2019-October}, pages = {128 – 133}, doi = {10.1109/SMC.2019.8914082}, url = {https://www.scopus.com/inward/record.uri?eid=2-s2.0-85076787727&doi=10.1109%2fSMC.2019.8914082&partnerID=40&md5=b067223578db03937c9be68febc5f920}, abstract = {Sheet metal forming processes are widely adopted in manufacturing industries and in the recent years there has been a growing demand for sheet metal items with different shapes and characteristics. However, the traditional process is unable to meet the modern industrial requirements, mainly due to the high costs of dies and the long manufacturing time cycles. On the contrary, developing products with high speed, low cost, and high quality is a key issue. Therefore, new methods and technologies to speed up the sheet metal forming process while keeping costs limited are needed. In particular, a key issue is the proper design of the forming process, which can benefit from the use of Information and Communications Technology (ICT) simulation techniques. This paper investigates the recent trends on ICT-based methodologies for sheet metal forming to identify the foremost research areas whose advancement will lead meeting the modern market's needs. © 2019 IEEE.}, keywords = {Costs; Information use; Metal forming; Metals; Developing product; Different shapes; Industrial requirements; Information and communications technology; Manufacturing industries; Manufacturing time; Simulation approach; Simulation technique; Sheet metal}, type = {Conference paper}, publication_stage = {Final}, source = {Scopus}, note = {Cited by: 3} }
- Hosseini, S. M., Carli, R. & Dotoli, M. (2019) Robust energy scheduling of interconnected smart homes with shared energy storage under quadratic pricing IN IEEE International Conference on Automation Science and Engineering., 966 – 971. doi:10.1109/COASE.2019.8843230
[BibTeX] [Abstract] [Download PDF]In this paper, we propose a novel robust framework for day-ahead energy scheduling of interconnected smart homes with shared energy storage system (ESS), taking into account users’ behavior uncertainty. The objective is minimizing the total energy payment for each user while satisfying the constraint on the feasibility of energy transactions between users and the power grid in presence of data uncertainty. Unlike most existing robust scheduling frameworks that assume a linear cost function for energy purchased from the grid, our design presents a tractable robust optimization scheme to solve the energy scheduling problem with a more realistic quadratic cost function. We model device/comfort constraints as well as contractual obligations imposed by the power grid restricting the users’ energy consumption to a maximum level at each time slot. Thus, in our problem, uncertainty affects both the quadratic objective function and linear contractual constraints. To solve the resulting problem, we first formulate a deterministic model of the scheduling problem, then establish a min-max robust counterpart, and finally apply some mathematical transformations to solve the equivalent problem. We also deal with the conservatism of the robust control algorithm and flexibility of the method for application to different settings. The validity and effectiveness of the proposed approach is verified by simulation results. © 2019 IEEE.
@CONFERENCE{Hosseini2019966, author = {Hosseini, Seyed Mohsen and Carli, Raffaele and Dotoli, Mariagrazia}, title = {Robust energy scheduling of interconnected smart homes with shared energy storage under quadratic pricing}, year = {2019}, journal = {IEEE International Conference on Automation Science and Engineering}, volume = {2019-August}, pages = {966 – 971}, doi = {10.1109/COASE.2019.8843230}, url = {https://www.scopus.com/inward/record.uri?eid=2-s2.0-85072987292&doi=10.1109%2fCOASE.2019.8843230&partnerID=40&md5=6989d41f45c9a005b8a3dd9ebcf6bbfd}, abstract = {In this paper, we propose a novel robust framework for day-ahead energy scheduling of interconnected smart homes with shared energy storage system (ESS), taking into account users' behavior uncertainty. The objective is minimizing the total energy payment for each user while satisfying the constraint on the feasibility of energy transactions between users and the power grid in presence of data uncertainty. Unlike most existing robust scheduling frameworks that assume a linear cost function for energy purchased from the grid, our design presents a tractable robust optimization scheme to solve the energy scheduling problem with a more realistic quadratic cost function. We model device/comfort constraints as well as contractual obligations imposed by the power grid restricting the users' energy consumption to a maximum level at each time slot. Thus, in our problem, uncertainty affects both the quadratic objective function and linear contractual constraints. To solve the resulting problem, we first formulate a deterministic model of the scheduling problem, then establish a min-max robust counterpart, and finally apply some mathematical transformations to solve the equivalent problem. We also deal with the conservatism of the robust control algorithm and flexibility of the method for application to different settings. The validity and effectiveness of the proposed approach is verified by simulation results. © 2019 IEEE.}, keywords = {Automation; Cost functions; Digital storage; Electric power transmission networks; Energy storage; Energy utilization; Intelligent buildings; Mathematical transformations; Optimization; Robust control; Scheduling; Contractual obligations; Deterministic modeling; Energy storage systems; Linear cost functions; Quadratic cost functions; Quadratic objective functions; Robust optimization; Scheduling problem; Costs}, type = {Conference paper}, publication_stage = {Final}, source = {Scopus}, note = {Cited by: 10} }
2018
- Carli, R., Dotoli, M. & Pellegrino, R. (2018) A decision-making tool for energy efficiency optimization of street lighting. IN Computers and Operations Research, 96.223 – 235. doi:10.1016/j.cor.2017.11.016
[BibTeX] [Abstract] [Download PDF]This paper develops a multi-criteria decision making tool to support the public decision maker in optimizing energy retrofit interventions on existing public street lighting systems. The related literature analysis clearly highlights that, to date, only a few number of studies deal with the definition of optimal decision strategies complying with multiple and conflicting objectives in the planning of street lighting refurbishment. To fill this gap, we propose a decision making tool that allows deciding, in an integrated way, the optimal energy retrofit plan in order to simultaneously reduce energy consumption, maintain comfort, protect the environment, and optimize the distribution of actions in subsystems, while ensuring an efficient use of public funds. The presented tool is applied to a real street lighting system of a wide urban area in Bari, Italy. The obtained results highlight that the approach effectively supports the city energy manager in the refurbishment of the street lighting systems. © 2017 Elsevier Ltd
@ARTICLE{Carli2018223, author = {Carli, Raffaele and Dotoli, Mariagrazia and Pellegrino, Roberta}, title = {A decision-making tool for energy efficiency optimization of street lighting}, year = {2018}, journal = {Computers and Operations Research}, volume = {96}, pages = {223 – 235}, doi = {10.1016/j.cor.2017.11.016}, url = {https://www.scopus.com/inward/record.uri?eid=2-s2.0-85044511260&doi=10.1016%2fj.cor.2017.11.016&partnerID=40&md5=f655fabb0054cefa3a05bcc0c63059f4}, abstract = {This paper develops a multi-criteria decision making tool to support the public decision maker in optimizing energy retrofit interventions on existing public street lighting systems. The related literature analysis clearly highlights that, to date, only a few number of studies deal with the definition of optimal decision strategies complying with multiple and conflicting objectives in the planning of street lighting refurbishment. To fill this gap, we propose a decision making tool that allows deciding, in an integrated way, the optimal energy retrofit plan in order to simultaneously reduce energy consumption, maintain comfort, protect the environment, and optimize the distribution of actions in subsystems, while ensuring an efficient use of public funds. The presented tool is applied to a real street lighting system of a wide urban area in Bari, Italy. The obtained results highlight that the approach effectively supports the city energy manager in the refurbishment of the street lighting systems. © 2017 Elsevier Ltd}, author_keywords = {Energy efficiency management; Multi-criteria optimization; Public street lighting}, keywords = {Decision making; Energy utilization; Lighting fixtures; Multiobjective optimization; Retrofitting; Street lighting; Urban planning; Conflicting objectives; Efficiency managements; Energy efficiency optimizations; Multi criteria decision making; Multicriteria optimization; Optimal decision strategy; Reduce energy consumption; Street lighting system; Energy efficiency}, type = {Article}, publication_stage = {Final}, source = {Scopus}, note = {Cited by: 70} }
- Carli, R. & Dotoli, M. (2018) A Distributed Control Algorithm for Optimal Charging of Electric Vehicle Fleets with Congestion Management , 373 – 378. doi:10.1016/j.ifacol.2018.07.061
[BibTeX] [Abstract] [Download PDF]This paper proposes a novel distributed control strategy for the optimal charging of a fleet of Electric Vehicles (EVs) in case of limited overall capacity of the electrical distribution network. The optimal charging is obtained as the solution of a scheduling problem aiming at a cost-optimal profile of the aggregated energy demand. The resulting optimization problem is formulated as a quadratic programming problem with a coupling of decision variables both in the objective function and in the constraint. We assume a minimal information structure, where users locally communicate only with their neighbors, without relying on a central decision maker. The solution approach relies on an iterative distributed algorithm based on duality, proximity, and consensus theory. A simulated case study demonstrates that the approach allows achieving the global optimum. © 2018
@CONFERENCE{Carli2018373, author = {Carli, Raffaele and Dotoli, Mariagrazia}, title = {A Distributed Control Algorithm for Optimal Charging of Electric Vehicle Fleets with Congestion Management}, year = {2018}, volume = {51}, number = {9}, pages = {373 – 378}, doi = {10.1016/j.ifacol.2018.07.061}, url = {https://www.scopus.com/inward/record.uri?eid=2-s2.0-85050093123&doi=10.1016%2fj.ifacol.2018.07.061&partnerID=40&md5=87f63aa0bd1f3aa33b573169b8a48e32}, abstract = {This paper proposes a novel distributed control strategy for the optimal charging of a fleet of Electric Vehicles (EVs) in case of limited overall capacity of the electrical distribution network. The optimal charging is obtained as the solution of a scheduling problem aiming at a cost-optimal profile of the aggregated energy demand. The resulting optimization problem is formulated as a quadratic programming problem with a coupling of decision variables both in the objective function and in the constraint. We assume a minimal information structure, where users locally communicate only with their neighbors, without relying on a central decision maker. The solution approach relies on an iterative distributed algorithm based on duality, proximity, and consensus theory. A simulated case study demonstrates that the approach allows achieving the global optimum. © 2018}, author_keywords = {Decentralized; Distributed Control; Electric Vehicles; Large scale optimization problems; Scheduling algorithms}, keywords = {Charging (batteries); Decision making; Electric vehicles; Fleet operations; Iterative methods; Quadratic programming; Scheduling algorithms; Decentralized; Distributed control; Distributed control algorithms; Distributed control strategy; Electric Vehicles (EVs); Electrical distribution networks; Large-scale optimization; Quadratic programming problems; Traffic congestion}, type = {Conference paper}, publication_stage = {Final}, source = {Scopus}, note = {Cited by: 37; All Open Access, Gold Open Access} }
- Hosseini, S. M., Carli, R. & Dotoli, M. (2018) Model Predictive Control for Real-Time Residential Energy Scheduling under Uncertainties IN Proceedings – 2018 IEEE International Conference on Systems, Man, and Cybernetics, SMC 2018., 1386 – 1391. doi:10.1109/SMC.2018.00242
[BibTeX] [Abstract] [Download PDF]This paper proposes a real-time strategy based on Model Predictive Control (MPC) for the energy scheduling of a grid-connected smart residential user equipped with deferrable and non-deferrable electrical appliances, a renewable energy source (RES), and an electrical energy storage system (EESS). The proposed control scheme relies on an iterative finite horizon on-line optimization, implementing a quadratic cost function to minimize the electricity bill of the user’s load demand and to limit the peak-to-average ratio (PAR) of the energy consumption profile whilst considering operational constraints. At each time step, the optimization problem is solved providing the cost-optimal energy consumption profile for the user’s deferrable loads and the optimal charging/discharging profile for the EESS, taking into account forecast uncertainties by using the most updated predicted values of local RES generation and non-deferrable loads consumption. The performance and effectiveness of the proposed framework are evaluated for a case study where the dynamics of the considered residential energy system is simulated under uncertainties both in the forecast of the RES generation and the non-deferrable loads energy consumption. © 2018 IEEE.
@CONFERENCE{Hosseini20181386, author = {Hosseini, Seyed Mohsen and Carli, Raffaele and Dotoli, Mariagrazia}, title = {Model Predictive Control for Real-Time Residential Energy Scheduling under Uncertainties}, year = {2018}, journal = {Proceedings - 2018 IEEE International Conference on Systems, Man, and Cybernetics, SMC 2018}, pages = {1386 – 1391}, doi = {10.1109/SMC.2018.00242}, url = {https://www.scopus.com/inward/record.uri?eid=2-s2.0-85062230383&doi=10.1109%2fSMC.2018.00242&partnerID=40&md5=2f024e90b4a067ff3c318f9e0a035f00}, abstract = {This paper proposes a real-time strategy based on Model Predictive Control (MPC) for the energy scheduling of a grid-connected smart residential user equipped with deferrable and non-deferrable electrical appliances, a renewable energy source (RES), and an electrical energy storage system (EESS). The proposed control scheme relies on an iterative finite horizon on-line optimization, implementing a quadratic cost function to minimize the electricity bill of the user's load demand and to limit the peak-to-average ratio (PAR) of the energy consumption profile whilst considering operational constraints. At each time step, the optimization problem is solved providing the cost-optimal energy consumption profile for the user's deferrable loads and the optimal charging/discharging profile for the EESS, taking into account forecast uncertainties by using the most updated predicted values of local RES generation and non-deferrable loads consumption. The performance and effectiveness of the proposed framework are evaluated for a case study where the dynamics of the considered residential energy system is simulated under uncertainties both in the forecast of the RES generation and the non-deferrable loads energy consumption. © 2018 IEEE.}, author_keywords = {energy scheduling; model predictive control (MPC); residential energy management; uncertainties}, keywords = {Cost functions; Electric energy storage; Energy utilization; Housing; Predictive control systems; Renewable energy resources; Scheduling; Electrical energy storage systems; Energy; Energy scheduling; Energy-consumption; Model predictive control; Model-predictive control; Renewable energy source; Residential energy; Residential energy management; Uncertainty; Model predictive control}, type = {Conference paper}, publication_stage = {Final}, source = {Scopus}, note = {Cited by: 31} }
- Carli, R., Dotoli, M. & Epicoco, N. (2018) Cost-Optimal Energy Scheduling of a Smart Home under Uncertainty IN 2018 IEEE Conference on Control Technology and Applications, CCTA 2018., 1668 – 1673. doi:10.1109/CCTA.2018.8511345
[BibTeX] [Abstract] [Download PDF]We present a novel energy scheduling approach under uncertain data for smart homes taking into account the presence of controllable electrical loads, renewable energy sources, dispatchable energy generators, and energy storage systems. The problem is stated as a fuzzy linear programming and is aimed at minimizing energy costs. The proposed approach allows managing the use of electrical devices, plan the energy production and supplying, and program the storage charging and discharging profiles under uncertain data. The method is validated through a literature case study showing its effectiveness in exploiting the potential of local energy generation and storage and in reducing the energy consumption costs, while limiting the peak average ratio of the energy profiles and complying with the user’s energy needs. © 2018 IEEE.
@CONFERENCE{Carli20181668, author = {Carli, R. and Dotoli, M. and Epicoco, N.}, title = {Cost-Optimal Energy Scheduling of a Smart Home under Uncertainty}, year = {2018}, journal = {2018 IEEE Conference on Control Technology and Applications, CCTA 2018}, pages = {1668 – 1673}, doi = {10.1109/CCTA.2018.8511345}, url = {https://www.scopus.com/inward/record.uri?eid=2-s2.0-85056899368&doi=10.1109%2fCCTA.2018.8511345&partnerID=40&md5=df2f65f9300879823bcc53a5aada2f14}, abstract = {We present a novel energy scheduling approach under uncertain data for smart homes taking into account the presence of controllable electrical loads, renewable energy sources, dispatchable energy generators, and energy storage systems. The problem is stated as a fuzzy linear programming and is aimed at minimizing energy costs. The proposed approach allows managing the use of electrical devices, plan the energy production and supplying, and program the storage charging and discharging profiles under uncertain data. The method is validated through a literature case study showing its effectiveness in exploiting the potential of local energy generation and storage and in reducing the energy consumption costs, while limiting the peak average ratio of the energy profiles and complying with the user's energy needs. © 2018 IEEE.}, keywords = {Automation; Energy utilization; Intelligent buildings; Linear programming; Renewable energy resources; Scheduling; Dispatchable energies; Electrical devices; Electrical load; Energy productions; Energy storage systems; Fuzzy linear programming; Minimizing energy; Renewable energy source; Digital storage}, type = {Conference paper}, publication_stage = {Final}, source = {Scopus}, note = {Cited by: 2} }
- Carli, R., Dotoli, M. & Epicoco, N. (2018) Monitoring traffic congestion in urban areas through probe vehicles: A case study analysis. IN Internet Technology Letters, 1.. doi:10.1002/ITL2.5
[BibTeX] [Abstract] [Download PDF]We present a probe vehicles approach to evaluate traffic congestion in urban areas. The method is illustrated by a real case study in Bari, Italy. Global Positioning System (GPS) data generated by buses are transmitted via General Packet Radio Service (GPRS) to a control station, where they are used to analyze traffic conditions. Traffic indices are evaluated to provide an exhaustive view of roads congestion and imple-ment suitable control strategies. Thus, traffic administrators can efficiently manage sustainable mobility in cities and people can acquire awareness on traffic and public transport performance. © 2017 John Wiley & Sons, Ltd.
@ARTICLE{Carli2018, author = {Carli, Raffaele and Dotoli, Mariagrazia and Epicoco, Nicola}, title = {Monitoring traffic congestion in urban areas through probe vehicles: A case study analysis}, year = {2018}, journal = {Internet Technology Letters}, volume = {1}, number = {4}, doi = {10.1002/ITL2.5}, url = {https://www.scopus.com/inward/record.uri?eid=2-s2.0-85067574084&doi=10.1002%2fITL2.5&partnerID=40&md5=ca6e723b32a9bcef3ba73f175d805843}, abstract = {We present a probe vehicles approach to evaluate traffic congestion in urban areas. The method is illustrated by a real case study in Bari, Italy. Global Positioning System (GPS) data generated by buses are transmitted via General Packet Radio Service (GPRS) to a control station, where they are used to analyze traffic conditions. Traffic indices are evaluated to provide an exhaustive view of roads congestion and imple-ment suitable control strategies. Thus, traffic administrators can efficiently manage sustainable mobility in cities and people can acquire awareness on traffic and public transport performance. © 2017 John Wiley & Sons, Ltd.}, author_keywords = {bus as a probe; traffic monitoring; vehicles to internet}, type = {Letter}, publication_stage = {Final}, source = {Scopus}, note = {Cited by: 11; All Open Access, Bronze Open Access} }
- Carli, R. & Dotoli, M. (2018) A Decentralized Control Strategy for the Energy Management of Smart Homes with Renewable Energy Exchange IN 2018 IEEE Conference on Control Technology and Applications, CCTA 2018., 1662 – 1667. doi:10.1109/CCTA.2018.8511617
[BibTeX] [Abstract] [Download PDF]This paper presents a decentralized control strategy for the scheduling of energy activities of interconnected smart homes that purchase energy from a supplier while exchanging renewable energy produced by individually owned distributed energy resources. The scheduling problem is solved with a twofold design objective. First, the model aims at reducing the overall energy supply from the grid, by allowing users to borrow/lend some amount of renewable energy from/to other users. Second, the problem is formulated to optimally plan users’ controllable loads. We assume a time-varying quadratic pricing of the energy purchased from the distribution network. The proposed solution is based on a decentralized optimization algorithm combining parametric optimization with the proximal Jacobian Alternating Direction Method of Multipliers. The application of the proposed technique to a simulated case study under several scenarios shows its effectiveness. © 2018 IEEE.
@CONFERENCE{Carli20181662, author = {Carli, Raffaele and Dotoli, Mariagrazia}, title = {A Decentralized Control Strategy for the Energy Management of Smart Homes with Renewable Energy Exchange}, year = {2018}, journal = {2018 IEEE Conference on Control Technology and Applications, CCTA 2018}, pages = {1662 – 1667}, doi = {10.1109/CCTA.2018.8511617}, url = {https://www.scopus.com/inward/record.uri?eid=2-s2.0-85056821403&doi=10.1109%2fCCTA.2018.8511617&partnerID=40&md5=85a9dde2ca8fa544e4b4a5901580188f}, abstract = {This paper presents a decentralized control strategy for the scheduling of energy activities of interconnected smart homes that purchase energy from a supplier while exchanging renewable energy produced by individually owned distributed energy resources. The scheduling problem is solved with a twofold design objective. First, the model aims at reducing the overall energy supply from the grid, by allowing users to borrow/lend some amount of renewable energy from/to other users. Second, the problem is formulated to optimally plan users' controllable loads. We assume a time-varying quadratic pricing of the energy purchased from the distribution network. The proposed solution is based on a decentralized optimization algorithm combining parametric optimization with the proximal Jacobian Alternating Direction Method of Multipliers. The application of the proposed technique to a simulated case study under several scenarios shows its effectiveness. © 2018 IEEE.}, keywords = {Automation; Energy resources; Intelligent buildings; Scheduling; Alternating direction method of multipliers; Controllable loads; Decentralized optimization; Design objectives; Distributed Energy Resources; Parametric optimization; Renewable energies; Scheduling problem; Decentralized control}, type = {Conference paper}, publication_stage = {Final}, source = {Scopus}, note = {Cited by: 4} }
- Carli, R. & Dotoli, M. (2018) Distributed Control for Waterfilling of Networked Control Systems with Coupling Constraints IN Proceedings of the IEEE Conference on Decision and Control., 3710 – 3715. doi:10.1109/CDC.2018.8619425
[BibTeX] [Abstract] [Download PDF]In this paper we present a distributed control approach for the multi-user multi-constrained waterfilling. This a specific category of distributed optimization for Networked Control Systems (NCSs), where agents aim at optimizing a non-separable global objective function while satisfying both local constraints and coupling constraints. Differently from the existing literature, in the considered setting we adopt a fully distributed mechanism where communication is allowed between neighbors only. First, we formulate a general multi-user waterfilling-structured optimization problem including coupling constraints, which may represent many engineering distributed control problems. Successively, we define a low-complexity iterative distributed algorithm based on duality, consensus and fixed point mapping theory. Finally, applying the technique to a simulated case referring to the electric vehicles optimal charging problem, we show its effectiveness. © 2018 IEEE.
@CONFERENCE{Carli20183710, author = {Carli, Raffaele and Dotoli, Mariagrazia}, title = {Distributed Control for Waterfilling of Networked Control Systems with Coupling Constraints}, year = {2018}, journal = {Proceedings of the IEEE Conference on Decision and Control}, volume = {2018-December}, pages = {3710 – 3715}, doi = {10.1109/CDC.2018.8619425}, url = {https://www.scopus.com/inward/record.uri?eid=2-s2.0-85062189943&doi=10.1109%2fCDC.2018.8619425&partnerID=40&md5=3dfd050026baf187539419a0a975db57}, abstract = {In this paper we present a distributed control approach for the multi-user multi-constrained waterfilling. This a specific category of distributed optimization for Networked Control Systems (NCSs), where agents aim at optimizing a non-separable global objective function while satisfying both local constraints and coupling constraints. Differently from the existing literature, in the considered setting we adopt a fully distributed mechanism where communication is allowed between neighbors only. First, we formulate a general multi-user waterfilling-structured optimization problem including coupling constraints, which may represent many engineering distributed control problems. Successively, we define a low-complexity iterative distributed algorithm based on duality, consensus and fixed point mapping theory. Finally, applying the technique to a simulated case referring to the electric vehicles optimal charging problem, we show its effectiveness. © 2018 IEEE.}, keywords = {Computational complexity; Distributed parameter control systems; Iterative methods; Coupling constraints; Distributed control; Distributed control problems; Distributed optimization; Global objective functions; Local constraints; Networked Control Systems (NCSs); Structured optimization problem; Networked control systems}, type = {Conference paper}, publication_stage = {Final}, source = {Scopus}, note = {Cited by: 4} }
- Carli, R., Dotoli, M. & Pellegrino, R. (2018) Multi-criteria decision-making for sustainable metropolitan cities assessment. IN Journal of Environmental Management, 226.46 – 61. doi:10.1016/j.jenvman.2018.07.075
[BibTeX] [Abstract] [Download PDF]The recent development of metropolitan cities, especially in Europe, requires an effective integrated management of city services, infrastructure, and communication networks at a metropolitan level. A preliminary step towards a proper organizational and management strategy of the metropolitan city is the analysis, benchmarking and optimization of the metropolitan areas through a set of indicators coherent with the overall sustainability objective of the metropolitan city. This paper proposes the use of the Analytic Hierarchy Process multi-criteria decision making technique for application in the smart metropolitan city context, with the aim of analysing the sustainable development of energy, water and environmental systems, through a set of objective performance indicators. Specifically, the 35 indicators defined for the Sustainable Development of Energy, Water and Environment Systems Index framework are used. The application of the approach to the real case study of four metropolitan areas (Bari, Bitonto, Mola, and Molfetta) in the city of Bari (Italy) shows its usefulness for the local government in benchmarking metropolitan areas and providing decision indications on how to formulate the sustainable development strategy of the metropolitan city. Based on the Analytic Hierarchy Process characteristics, the results highlight that although one specific area (Mola in the considered case) is globally ranked at the first place, it is only ranked first with respect to some dimensions. Such a result has strong implications for the metropolitan city’s manager who has the possibility to identify and implement targeted actions, which may be designed ad hoc to improve specific dimensions based on the current state of the city, thus maximizing the efficiency and effectiveness of the actions undertaken for the sustainable development of energy, water and environmental systems of the whole metropolitan city. © 2018 Elsevier Ltd
@ARTICLE{Carli201846, author = {Carli, Raffaele and Dotoli, Mariagrazia and Pellegrino, Roberta}, title = {Multi-criteria decision-making for sustainable metropolitan cities assessment}, year = {2018}, journal = {Journal of Environmental Management}, volume = {226}, pages = {46 – 61}, doi = {10.1016/j.jenvman.2018.07.075}, url = {https://www.scopus.com/inward/record.uri?eid=2-s2.0-85051362383&doi=10.1016%2fj.jenvman.2018.07.075&partnerID=40&md5=57606eb68995a362a44497e2b3089e36}, abstract = {The recent development of metropolitan cities, especially in Europe, requires an effective integrated management of city services, infrastructure, and communication networks at a metropolitan level. A preliminary step towards a proper organizational and management strategy of the metropolitan city is the analysis, benchmarking and optimization of the metropolitan areas through a set of indicators coherent with the overall sustainability objective of the metropolitan city. This paper proposes the use of the Analytic Hierarchy Process multi-criteria decision making technique for application in the smart metropolitan city context, with the aim of analysing the sustainable development of energy, water and environmental systems, through a set of objective performance indicators. Specifically, the 35 indicators defined for the Sustainable Development of Energy, Water and Environment Systems Index framework are used. The application of the approach to the real case study of four metropolitan areas (Bari, Bitonto, Mola, and Molfetta) in the city of Bari (Italy) shows its usefulness for the local government in benchmarking metropolitan areas and providing decision indications on how to formulate the sustainable development strategy of the metropolitan city. Based on the Analytic Hierarchy Process characteristics, the results highlight that although one specific area (Mola in the considered case) is globally ranked at the first place, it is only ranked first with respect to some dimensions. Such a result has strong implications for the metropolitan city's manager who has the possibility to identify and implement targeted actions, which may be designed ad hoc to improve specific dimensions based on the current state of the city, thus maximizing the efficiency and effectiveness of the actions undertaken for the sustainable development of energy, water and environmental systems of the whole metropolitan city. © 2018 Elsevier Ltd}, author_keywords = {Analytic hierarchy process; Multi-criteria decision making; Performance evaluation; Planning; Sustainable development}, keywords = {Cities; Conservation of Natural Resources; Decision Making; Europe; Italy; Bari [Bari (ADS)]; Bari [Puglia]; Italy; Puglia; Analytic hierarchy process; Benchmarking; Decision making; Energy systems; Environmental systems; Integrated management; Metropolitan area; Metropolitan cities; Multi criteria decision-making; Multicriteria decision-making; Multicriterion decision makings; Performances evaluation; Water system; analytical hierarchy process; assessment method; benchmarking; decision making; development strategy; local government; metropolitan area; multicriteria analysis; optimization; performance assessment; sustainability; sustainable development; urban planning; Article; benchmarking; city; decision making; energy resource; environmental sustainability; government; Italy; sustainable development; water supply; city; environmental protection; Europe; Sustainable development}, type = {Article}, publication_stage = {Final}, source = {Scopus}, note = {Cited by: 93} }
2017
- Carli, R., Dotoli, M., Garramone, R., Andria, G. & Lanzolla, A. M. L. (2017) An average consensus approach for the optimal allocation of a shared renewable energy source IN 2016 IEEE International Conference on Systems, Man, and Cybernetics, SMC 2016 – Conference Proceedings., 270 – 275. doi:10.1109/SMC.2016.7844253
[BibTeX] [Abstract] [Download PDF]This paper investigates the problem of optimally distributing the energy produced by a shared renewable energy source among users, without relying on a centralized decision maker. We assume that each user is only allowed to communicate with his neighbors and buys energy from a producer under non-linear pricing. We formulate a quadratic programming problem aimed at ensuring a social welfare-optimal allocation of the shared resource. We propose a low-complexity distributed algorithm that relies on average consensus. We show the convergence of the proposed algorithm to the unique optimal solution of the resource allocation problem. We also provide numerical simulations demonstrating that the approach allows exploiting the potential of renewable energy sources’ sharing to reduce users’ energy consumption costs. © 2016 IEEE.
@CONFERENCE{Carli2017270, author = {Carli, Raffaele and Dotoli, Mariagrazia and Garramone, Raffaele and Andria, Gregorio and Lanzolla, Anna Maria Lucia}, title = {An average consensus approach for the optimal allocation of a shared renewable energy source}, year = {2017}, journal = {2016 IEEE International Conference on Systems, Man, and Cybernetics, SMC 2016 - Conference Proceedings}, pages = {270 – 275}, doi = {10.1109/SMC.2016.7844253}, url = {https://www.scopus.com/inward/record.uri?eid=2-s2.0-85015803915&doi=10.1109%2fSMC.2016.7844253&partnerID=40&md5=8769614afb1c81c7bc718b4190b95117}, abstract = {This paper investigates the problem of optimally distributing the energy produced by a shared renewable energy source among users, without relying on a centralized decision maker. We assume that each user is only allowed to communicate with his neighbors and buys energy from a producer under non-linear pricing. We formulate a quadratic programming problem aimed at ensuring a social welfare-optimal allocation of the shared resource. We propose a low-complexity distributed algorithm that relies on average consensus. We show the convergence of the proposed algorithm to the unique optimal solution of the resource allocation problem. We also provide numerical simulations demonstrating that the approach allows exploiting the potential of renewable energy sources' sharing to reduce users' energy consumption costs. © 2016 IEEE.}, author_keywords = {Average consensus; Distributed optimization; Energy management; Multi-period resource allocation; Renewable energy sources}, keywords = {Computational complexity; Cybernetics; Decision making; Economics; Energy management; Energy utilization; Natural resources; Optimization; Quadratic programming; Resource allocation; Average consensus; Distributed optimization; Multi-period; Non-linear pricing; Optimal allocation; Quadratic programming problems; Renewable energy source; Resource allocation problem; Renewable energy resources}, type = {Conference paper}, publication_stage = {Final}, source = {Scopus}, note = {Cited by: 1} }
- Carli, R. & Dotoli, M. (2017) Cooperative Distributed Control for the Energy Scheduling of Smart Homes with Shared Energy Storage and Renewable Energy Source IN IFAC-PapersOnLine., 8867 – 8872. doi:10.1016/j.ifacol.2017.08.1544
[BibTeX] [Abstract] [Download PDF]This paper presents a distributed control technique for the energy scheduling of a group of interconnected smart city residential users. The proposed model aims at a simultaneous cost-optimal planning of users’ controllable appliances and of the shared storage system charge/discharge and renewable energy source. The distributed control algorithm is based on an iterative procedure combining parametric optimization with the block coordinate descent method. A realistic case study simulated in different scenarios demonstrates that the approach allows fully exploiting the potential of storage systems sharing to reduce individual users’ energy consumption costs and limit the peak average ratio of the energy profiles. © 2017
@CONFERENCE{Carli20178867, author = {Carli, Raffaele and Dotoli, Mariagrazia}, title = {Cooperative Distributed Control for the Energy Scheduling of Smart Homes with Shared Energy Storage and Renewable Energy Source}, year = {2017}, journal = {IFAC-PapersOnLine}, volume = {50}, number = {1}, pages = {8867 – 8872}, doi = {10.1016/j.ifacol.2017.08.1544}, url = {https://www.scopus.com/inward/record.uri?eid=2-s2.0-85031787804&doi=10.1016%2fj.ifacol.2017.08.1544&partnerID=40&md5=9d6a82e7d9f16756a8d263254e537f45}, abstract = {This paper presents a distributed control technique for the energy scheduling of a group of interconnected smart city residential users. The proposed model aims at a simultaneous cost-optimal planning of users’ controllable appliances and of the shared storage system charge/discharge and renewable energy source. The distributed control algorithm is based on an iterative procedure combining parametric optimization with the block coordinate descent method. A realistic case study simulated in different scenarios demonstrates that the approach allows fully exploiting the potential of storage systems sharing to reduce individual users’ energy consumption costs and limit the peak average ratio of the energy profiles. © 2017}, author_keywords = {Decentralized; Distributed Control; Distribution Management Systems; Energy; Energy Storage Operation; Large scale optimization problems; Planning; Smart Grids}, keywords = {Automation; Distributed parameter control systems; Energy storage; Energy utilization; Intelligent buildings; Iterative methods; Natural resources; Planning; Renewable energy resources; Scheduling; Decentralized; Distributed control; Distribution management systems; Energy; Large-scale optimization; Smart grid; Storage operations; Smart power grids}, type = {Conference paper}, publication_stage = {Final}, source = {Scopus}, note = {Cited by: 37; All Open Access, Gold Open Access} }
- Carli, R. & Dotoli, M. (2017) A decentralized control strategy for energy retrofit planning of large-scale street lighting systems using dynamic programming IN IEEE International Conference on Automation Science and Engineering., 1196 – 1200. doi:10.1109/COASE.2017.8256266
[BibTeX] [Abstract] [Download PDF]This paper addresses the energy management of large-scale urban street lighting systems. We propose a multi-stage decision-making procedure that supports the energy manager in determining the optimal energy retrofit plan of an existing public street lighting system. The problem statement is based on a quadratic integer programming formulation and aims at simultaneously reducing the energy consumption, ensuring an optimal allocation of the retrofit actions, and efficiently using the available budget. The proposed solution relies on a decentralized optimization algorithm that is based on discrete dynamic programming. The methodology is applied to a real street lighting system in the city of Bari, Italy. © 2017 IEEE.
@CONFERENCE{Carli20171196, author = {Carli, Raffaele and Dotoli, Mariagrazia}, title = {A decentralized control strategy for energy retrofit planning of large-scale street lighting systems using dynamic programming}, year = {2017}, journal = {IEEE International Conference on Automation Science and Engineering}, volume = {2017-August}, pages = {1196 – 1200}, doi = {10.1109/COASE.2017.8256266}, url = {https://www.scopus.com/inward/record.uri?eid=2-s2.0-85044970276&doi=10.1109%2fCOASE.2017.8256266&partnerID=40&md5=7f8ba32dffa1946d990b4fa9bdfa7a59}, abstract = {This paper addresses the energy management of large-scale urban street lighting systems. We propose a multi-stage decision-making procedure that supports the energy manager in determining the optimal energy retrofit plan of an existing public street lighting system. The problem statement is based on a quadratic integer programming formulation and aims at simultaneously reducing the energy consumption, ensuring an optimal allocation of the retrofit actions, and efficiently using the available budget. The proposed solution relies on a decentralized optimization algorithm that is based on discrete dynamic programming. The methodology is applied to a real street lighting system in the city of Bari, Italy. © 2017 IEEE.}, keywords = {Budget control; Decision making; Energy management; Energy management systems; Energy utilization; Integer programming; Lighting fixtures; Retrofitting; Street lighting; Decentralized optimization; Decision making procedure; Energy managers; Optimal allocation; Problem statement; Quadratic integer programming; Retrofit actions; Street lighting system; Dynamic programming}, type = {Conference paper}, publication_stage = {Final}, source = {Scopus}, note = {Cited by: 2} }
- Carli, R., Dotoli, M. & Pellegrino, R. (2017) A Hierarchical Decision-Making Strategy for the Energy Management of Smart Cities. IN IEEE Transactions on Automation Science and Engineering, 14.505 – 523. doi:10.1109/TASE.2016.2593101
[BibTeX] [Abstract] [Download PDF]This paper presents a hierarchical decision-making strategy for the energy management of a smart city. The proposed decision process supports the city energy manager and local policy makers in taking energy retrofit decisions on different urban sectors by an integrated, structured, and transparent management. To this aim, in the proposed decision strategy, a bilevel programming model integrates several local decision-making units, each focusing on the energy retrofit optimization of a specific urban subsystem, and a central decision unit. We solve the hierarchical decision problem by a game theoretic distributed algorithm. We apply the developed decision model to the case study of the city of Bari (Italy), where a smart city program has recently been launched. © 2015 IEEE.
@ARTICLE{Carli2017505, author = {Carli, Raffaele and Dotoli, Mariagrazia and Pellegrino, Roberta}, title = {A Hierarchical Decision-Making Strategy for the Energy Management of Smart Cities}, year = {2017}, journal = {IEEE Transactions on Automation Science and Engineering}, volume = {14}, number = {2}, pages = {505 – 523}, doi = {10.1109/TASE.2016.2593101}, url = {https://www.scopus.com/inward/record.uri?eid=2-s2.0-85027724511&doi=10.1109%2fTASE.2016.2593101&partnerID=40&md5=b6d44e7085576476833b789dae66c70a}, abstract = {This paper presents a hierarchical decision-making strategy for the energy management of a smart city. The proposed decision process supports the city energy manager and local policy makers in taking energy retrofit decisions on different urban sectors by an integrated, structured, and transparent management. To this aim, in the proposed decision strategy, a bilevel programming model integrates several local decision-making units, each focusing on the energy retrofit optimization of a specific urban subsystem, and a central decision unit. We solve the hierarchical decision problem by a game theoretic distributed algorithm. We apply the developed decision model to the case study of the city of Bari (Italy), where a smart city program has recently been launched. © 2015 IEEE.}, author_keywords = {Bilevel programming; energy efficiency; energy management; game theory; hierarchical decision making; optimization; smart city}, keywords = {Decision theory; Energy efficiency; Energy management; Game theory; Optimization; Retrofitting; Smart city; Bi-level programming; Bilevel programming models; Decision modeling; Decision process; Decision strategy; Energy retrofit; Hierarchical decisions; Local decision-making; Decision making}, type = {Article}, publication_stage = {Final}, source = {Scopus}, note = {Cited by: 69} }
- Carli, R. & Dotoli, M. (2017) A decentralized control strategy for optimal charging of electric vehicle fleets with congestion management IN Proceedings – 2017 IEEE International Conference on Service Operations and Logistics, and Informatics, SOLI 2017., 63 – 67. doi:10.1109/SOLI.2017.8120971
[BibTeX] [Abstract] [Download PDF]This paper proposes a novel decentralized control strategy for the optimal charging of a large-scale fleet of Electric Vehicles (EVs). The scheduling problem aims at ensuring a cost-optimal profile of the aggregated energy demand and at satisfying the resource constraints depending both on power grid components capacity and EV locations in the distribution network. The resulting optimization problem is formulated as a quadratic programming problem with a coupling of decision variables both in the objective function and in the inequality constraints. The solution approach relies on a decentralized optimization algorithm that is based on a variant of ADMM (Alternating Direction Method of Multipliers), adapted to take into account the inequality constraints and the non-separated objective function. A simulated case study demonstrates that the approach allows achieving both the overall fleet and individual EV goals, while complying with the power grid congestion limits. © 2017 IEEE.
@CONFERENCE{Carli201763, author = {Carli, Raffaele and Dotoli, Mariagrazia}, title = {A decentralized control strategy for optimal charging of electric vehicle fleets with congestion management}, year = {2017}, journal = {Proceedings - 2017 IEEE International Conference on Service Operations and Logistics, and Informatics, SOLI 2017}, volume = {2017-January}, pages = {63 – 67}, doi = {10.1109/SOLI.2017.8120971}, url = {https://www.scopus.com/inward/record.uri?eid=2-s2.0-85046260212&doi=10.1109%2fSOLI.2017.8120971&partnerID=40&md5=2ced08ace41770b457c6f07d8cbe98b5}, abstract = {This paper proposes a novel decentralized control strategy for the optimal charging of a large-scale fleet of Electric Vehicles (EVs). The scheduling problem aims at ensuring a cost-optimal profile of the aggregated energy demand and at satisfying the resource constraints depending both on power grid components capacity and EV locations in the distribution network. The resulting optimization problem is formulated as a quadratic programming problem with a coupling of decision variables both in the objective function and in the inequality constraints. The solution approach relies on a decentralized optimization algorithm that is based on a variant of ADMM (Alternating Direction Method of Multipliers), adapted to take into account the inequality constraints and the non-separated objective function. A simulated case study demonstrates that the approach allows achieving both the overall fleet and individual EV goals, while complying with the power grid congestion limits. © 2017 IEEE.}, author_keywords = {Alternating direction method of multipliers; Congestion management; Coupled objective function; Decentralized optimization; Electric vehicle charging; Sharing}, keywords = {Charging (batteries); Constraint satisfaction problems; Constraint theory; Decentralized control; Electric power transmission networks; Electric vehicles; Fleet operations; Quadratic programming; Traffic congestion; Alternating direction method of multipliers; Congestion management; Decentralized optimization; Electric vehicle charging; Objective functions; Sharing; Electric machine control}, type = {Conference paper}, publication_stage = {Final}, source = {Scopus}, note = {Cited by: 12} }
- Carli, R. & Dotoli, M. (2017) Using the distributed proximal alternating direction method of multipliers for smart grid monitoring IN IEEE International Conference on Automation Science and Engineering., 418 – 423. doi:10.1109/COASE.2017.8256140
[BibTeX] [Abstract] [Download PDF]Efficient and effective monitoring represents the starting point for a reliable and secure smart grid. Given the increasing size and complexity of power networks and the pressing concerns on privacy and robustness, the development of intelligent and flexible distributed monitoring systems represents a crucial issue in both structuring and operating future grids. In this context, this paper presents a distributed optimization framework for use in smart grid monitoring. We propose a distributed algorithm based on ADMM (Alternating Direction Method of Multipliers) for use in large scale optimization problems in smart grid monitoring. The proposed solution is based upon a local-based optimization process, where a limited amount of information is exchanged only between neighboring nodes in a locally broadcast fashion. Applying the approach to two illustrating examples demonstrates it allows exploiting the scalability and efficiency of distributed ADMM for distributed smart grid monitoring. © 2017 IEEE.
@CONFERENCE{Carli2017418, author = {Carli, Raffaele and Dotoli, Mariagrazia}, title = {Using the distributed proximal alternating direction method of multipliers for smart grid monitoring}, year = {2017}, journal = {IEEE International Conference on Automation Science and Engineering}, volume = {2017-August}, pages = {418 – 423}, doi = {10.1109/COASE.2017.8256140}, url = {https://www.scopus.com/inward/record.uri?eid=2-s2.0-85044966539&doi=10.1109%2fCOASE.2017.8256140&partnerID=40&md5=dbecf71fd06d5b7c380c0ef1f2547de4}, abstract = {Efficient and effective monitoring represents the starting point for a reliable and secure smart grid. Given the increasing size and complexity of power networks and the pressing concerns on privacy and robustness, the development of intelligent and flexible distributed monitoring systems represents a crucial issue in both structuring and operating future grids. In this context, this paper presents a distributed optimization framework for use in smart grid monitoring. We propose a distributed algorithm based on ADMM (Alternating Direction Method of Multipliers) for use in large scale optimization problems in smart grid monitoring. The proposed solution is based upon a local-based optimization process, where a limited amount of information is exchanged only between neighboring nodes in a locally broadcast fashion. Applying the approach to two illustrating examples demonstrates it allows exploiting the scalability and efficiency of distributed ADMM for distributed smart grid monitoring. © 2017 IEEE.}, author_keywords = {ADMM; distributed optimization; monitoring; sensors network; smart grid}, keywords = {Electric power transmission networks; Monitoring; ADMM; Alternating direction method of multipliers; Amount of information; Distributed monitoring systems; Distributed optimization; Large-scale optimization; Sensors network; Smart grid; Smart power grids}, type = {Conference paper}, publication_stage = {Final}, source = {Scopus}, note = {Cited by: 2} }
- Carli, R., Dotoli, M., Pellegrino, R. & Ranieri, L. (2017) A Decision Making Technique to Optimize a Buildings’ Stock Energy Efficiency. IN IEEE Transactions on Systems, Man, and Cybernetics: Systems, 47.794 – 807. doi:10.1109/TSMC.2016.2521836
[BibTeX] [Abstract] [Download PDF]This paper focuses on applying multicriteria decision making tools to determine an optimal energy retrofit plan for a portfolio of buildings. We present a two-step decision making technique employing a multiobjective optimization algorithm followed by a multiattribute ranking procedure. The method aims at deciding, in an integrated way, the optimal energy retrofit plan for a whole stock of buildings, optimizing efficiency, sustainability, and comfort, while effectively allocating the available financial resources to the buildings. The proposed methodology is applied to a real stock of public buildings in Bari, Italy. The obtained results demonstrate that the approach effectively supports the city governance in making decisions for the optimal management of the buildings’ energy efficiency. © 2016 IEEE.
@ARTICLE{Carli2017794, author = {Carli, Raffaele and Dotoli, Mariagrazia and Pellegrino, Roberta and Ranieri, Luigi}, title = {A Decision Making Technique to Optimize a Buildings' Stock Energy Efficiency}, year = {2017}, journal = {IEEE Transactions on Systems, Man, and Cybernetics: Systems}, volume = {47}, number = {5}, pages = {794 – 807}, doi = {10.1109/TSMC.2016.2521836}, url = {https://www.scopus.com/inward/record.uri?eid=2-s2.0-85018489196&doi=10.1109%2fTSMC.2016.2521836&partnerID=40&md5=db70236a891233a4576656e616023e08}, abstract = {This paper focuses on applying multicriteria decision making tools to determine an optimal energy retrofit plan for a portfolio of buildings. We present a two-step decision making technique employing a multiobjective optimization algorithm followed by a multiattribute ranking procedure. The method aims at deciding, in an integrated way, the optimal energy retrofit plan for a whole stock of buildings, optimizing efficiency, sustainability, and comfort, while effectively allocating the available financial resources to the buildings. The proposed methodology is applied to a real stock of public buildings in Bari, Italy. The obtained results demonstrate that the approach effectively supports the city governance in making decisions for the optimal management of the buildings' energy efficiency. © 2016 IEEE.}, author_keywords = {Building management; energy efficiency; multiattribute analysis; multicriteria decision making; multiobjective optimization (MOO); optimization algorithms}, keywords = {Buildings; Energy efficiency; Multiobjective optimization; Optimization; Retrofitting; Building management; Financial resources; Multi criteria decision making; Multi-attribute analysis; Multiattribute rankings; Optimal management; Optimization algorithms; Public buildings; Decision making}, type = {Article}, publication_stage = {Final}, source = {Scopus}, note = {Cited by: 53} }
- Carli, R. & Dotoli, M. (2017) Bi-level programming for the energy retrofit planning of street lighting systems IN Proceedings of the 2017 IEEE 14th International Conference on Networking, Sensing and Control, ICNSC 2017., 543 – 548. doi:10.1109/ICNSC.2017.8000150
[BibTeX] [Abstract] [Download PDF]This paper addresses strategic decision making issues for the energy management of urban street lighting. We propose a hierarchical decision procedure that supports the energy manager in determining the optimal energy retrofit plan of an existing public street lighting system throughout a wide urban area. A bi-level programming model integrates several decision making units, each focusing on the energy optimization of a specific limited zone lighting system, and a central decision unit, aiming at a fair distribution of actions among these various systems, while ensuring an efficient use of public funds. We apply the technique to the case study of the city of Bari (Italy), to demonstrate the applicability and efficiency of the proposed optimization model. © 2017 IEEE.
@CONFERENCE{Carli2017543, author = {Carli, Raffaele and Dotoli, Mariagrazia}, title = {Bi-level programming for the energy retrofit planning of street lighting systems}, year = {2017}, journal = {Proceedings of the 2017 IEEE 14th International Conference on Networking, Sensing and Control, ICNSC 2017}, pages = {543 – 548}, doi = {10.1109/ICNSC.2017.8000150}, url = {https://www.scopus.com/inward/record.uri?eid=2-s2.0-85028509849&doi=10.1109%2fICNSC.2017.8000150&partnerID=40&md5=7aa2cad3d9d4ab8f45ecac5cd40a808f}, abstract = {This paper addresses strategic decision making issues for the energy management of urban street lighting. We propose a hierarchical decision procedure that supports the energy manager in determining the optimal energy retrofit plan of an existing public street lighting system throughout a wide urban area. A bi-level programming model integrates several decision making units, each focusing on the energy optimization of a specific limited zone lighting system, and a central decision unit, aiming at a fair distribution of actions among these various systems, while ensuring an efficient use of public funds. We apply the technique to the case study of the city of Bari (Italy), to demonstrate the applicability and efficiency of the proposed optimization model. © 2017 IEEE.}, author_keywords = {Bilevel programming; Decision support system; Energy efficiency; Energy management; Hierarchical optimization; Street lighting}, keywords = {Artificial intelligence; Decision making; Decision support systems; Energy efficiency; Energy management; Lighting; Lighting fixtures; Optimization; Retrofitting; Street lighting; Urban planning; Bi-level programming; Bilevel programming models; Decision making unit; Hierarchical decisions; Hierarchical optimization; Optimization modeling; Strategic decision making; Street lighting system; Energy management systems}, type = {Conference paper}, publication_stage = {Final}, source = {Scopus}, note = {Cited by: 1} }
- Carli, R., Dotoli, M. & Cianci, E. (2017) An optimization tool for energy efficiency of street lighting systems in smart cities IN IFAC-PapersOnLine., 14460 – 14464. doi:10.1016/j.ifacol.2017.08.2292
[BibTeX] [Abstract] [Download PDF]This paper develops a decision making tool to support the public decision maker in selecting the optimal energy retrofit interventions on an existing street lighting system. The problem statement is based on a quadratic integer programming formulation and deals with simultaneously reducing the energy consumption and ensuring an optimal allocation of the retrofit actions among the street lighting subsystems. The methodology is applied to a real street lighting system in Bari, Italy. The obtained results demonstrate that the approach effectively supports the city governance in making decisions for the optimal energy management of the street lighting. © 2017
@CONFERENCE{Carli201714460, author = {Carli, Raffaele and Dotoli, Mariagrazia and Cianci, Edmondo}, title = {An optimization tool for energy efficiency of street lighting systems in smart cities}, year = {2017}, journal = {IFAC-PapersOnLine}, volume = {50}, number = {1}, pages = {14460 – 14464}, doi = {10.1016/j.ifacol.2017.08.2292}, url = {https://www.scopus.com/inward/record.uri?eid=2-s2.0-85042493832&doi=10.1016%2fj.ifacol.2017.08.2292&partnerID=40&md5=b1ee03a53ee4a57450cc0e590002a9e6}, abstract = {This paper develops a decision making tool to support the public decision maker in selecting the optimal energy retrofit interventions on an existing street lighting system. The problem statement is based on a quadratic integer programming formulation and deals with simultaneously reducing the energy consumption and ensuring an optimal allocation of the retrofit actions among the street lighting subsystems. The methodology is applied to a real street lighting system in Bari, Italy. The obtained results demonstrate that the approach effectively supports the city governance in making decisions for the optimal energy management of the street lighting. © 2017}, author_keywords = {Decision Making; Distribution Management Systems; Energy; Optimization; Smart Cities; Street Lighting}, keywords = {Energy efficiency; Energy utilization; Integer programming; Lighting fixtures; Optimization; Retrofitting; Smart city; Street lighting; Decision making tool; Distribution management systems; Energy; Optimal allocation; Optimization tools; Problem statement; Quadratic integer programming; Street lighting system; Decision making}, type = {Conference paper}, publication_stage = {Final}, source = {Scopus}, note = {Cited by: 44; All Open Access, Gold Open Access} }
- Carli, R. & Dotoli, M. (2017) A distributed control algorithm for waterfilling of networked control systems via consensus. IN IEEE Control Systems Letters, 1.334 – 339. doi:10.1109/LCSYS.2017.2716190
[BibTeX] [Abstract] [Download PDF]This letter presents a distributed waterfilling algorithm for networked control systems where users communicate with neighbors only. Waterfilling—a well-known optimization approach in communication systems—has inspired practical resolution methods for several control engineering and decision-making problems. This letter proposes a fully distributed solution for waterfilling of networked control systems. We consider multiple coupled waterlevels among users that locally communicate only with neighbors, without a central decision maker. We define two alternative versions (an exact one and an approximated one) of a novel distributed algorithm combining consensus, proximity, and the fixed point mapping theory, and show its convergence. We illustrate the technique by a case study on the charging of a fleet of electric vehicles. © 2017 IEEE.
@ARTICLE{Carli2017334, author = {Carli, Raffaele and Dotoli, Mariagrazia}, title = {A distributed control algorithm for waterfilling of networked control systems via consensus}, year = {2017}, journal = {IEEE Control Systems Letters}, volume = {1}, number = {2}, pages = {334 – 339}, doi = {10.1109/LCSYS.2017.2716190}, url = {https://www.scopus.com/inward/record.uri?eid=2-s2.0-85050079250&doi=10.1109%2fLCSYS.2017.2716190&partnerID=40&md5=595d4be8879ccf3a42f0de75793d9038}, abstract = {This letter presents a distributed waterfilling algorithm for networked control systems where users communicate with neighbors only. Waterfilling—a well-known optimization approach in communication systems—has inspired practical resolution methods for several control engineering and decision-making problems. This letter proposes a fully distributed solution for waterfilling of networked control systems. We consider multiple coupled waterlevels among users that locally communicate only with neighbors, without a central decision maker. We define two alternative versions (an exact one and an approximated one) of a novel distributed algorithm combining consensus, proximity, and the fixed point mapping theory, and show its convergence. We illustrate the technique by a case study on the charging of a fleet of electric vehicles. © 2017 IEEE.}, author_keywords = {Distributed control; Networked control systems; Optimization}, keywords = {Control theory; Decision making; Distributed parameter control systems; Fleet operations; Optimization; Decision makers; Decision-making problem; Distributed control; Distributed control algorithms; Distributed solutions; Optimization approach; Resolution methods; Water-filling algorithm; Networked control systems}, type = {Article}, publication_stage = {Final}, source = {Scopus}, note = {Cited by: 30} }
2016
- Carli, R., Dotoli, M., Andria, G. & Lanzolla, A. M. L. (2016) Bi-level programming for the strategic energy management of a smart city IN EESMS 2016 – 2016 IEEE Workshop on Environmental, Energy, and Structural Monitoring Systems, Proceedings.. doi:10.1109/EESMS.2016.7504820
[BibTeX] [Abstract] [Download PDF]This paper addresses the emerging need for tools devoted to the strategic energy management of smart cities. We propose a novel decision model that supports the energy manager in governing the smart city while addressing different urban sectors with an integrated and structured energy retrofit planning. A bi-level programming model integrates several decision making units (decision panels), each focusing on the energy optimization of a specific urban subsystem, and a central decision unit. We solve the bi-level decision problem by a game theoretic distributed approach. We apply the developed decision model to the case study of the city of Bari (Italy), where a smart city program has recently been launched. © 2016 IEEE.
@CONFERENCE{Carli2016, author = {Carli, Raffaele and Dotoli, Mariagrazia and Andria, Gregorio and Lanzolla, Anna Maria Lucia}, title = {Bi-level programming for the strategic energy management of a smart city}, year = {2016}, journal = {EESMS 2016 - 2016 IEEE Workshop on Environmental, Energy, and Structural Monitoring Systems, Proceedings}, doi = {10.1109/EESMS.2016.7504820}, url = {https://www.scopus.com/inward/record.uri?eid=2-s2.0-84980371756&doi=10.1109%2fEESMS.2016.7504820&partnerID=40&md5=645711d93f3fee6a0e376fe0decdd093}, abstract = {This paper addresses the emerging need for tools devoted to the strategic energy management of smart cities. We propose a novel decision model that supports the energy manager in governing the smart city while addressing different urban sectors with an integrated and structured energy retrofit planning. A bi-level programming model integrates several decision making units (decision panels), each focusing on the energy optimization of a specific urban subsystem, and a central decision unit. We solve the bi-level decision problem by a game theoretic distributed approach. We apply the developed decision model to the case study of the city of Bari (Italy), where a smart city program has recently been launched. © 2016 IEEE.}, author_keywords = {bi-level programming; decision support system; distributed optimization; energy efficiency; energy management; smart city}, keywords = {Artificial intelligence; Decision support systems; Distributed computer systems; Energy efficiency; Energy management; Game theory; Monitoring; Bi-level programming; Bilevel programming models; Decision making unit; Distributed approaches; Distributed optimization; Energy optimization; Smart cities; Strategic energy management; Decision making}, type = {Conference paper}, publication_stage = {Final}, source = {Scopus}, note = {Cited by: 6} }
2015
- Carli, R., Dotoli, M., Epicoco, N., Angelico, B. & Vinciullo, A. (2015) Automated evaluation of urban traffic congestion using bus as a probe IN IEEE International Conference on Automation Science and Engineering., 967 – 972. doi:10.1109/CoASE.2015.7294224
[BibTeX] [Abstract] [Download PDF]This paper presents an algorithm for the automated analysis and evaluation of vehicular traffic congestion in urban areas. The proposed approach is based on the concept of bus as a probe and makes use of GPS-generated data provided by a local transit bus tracking system. Archived GPS pulses are analyzed offline to extract valuable indices related to general urban traffic characteristics and aimed at generating a detailed view of the urban roads congestion. This information is useful both for policy makers, to effectively address the management of sustainable mobility in urban areas, and for citizens, to acquire awareness about congestion times and location zones. The presented algorithm is applied to a part of the urban road network of the municipality of Bari (Italy). © 2015 IEEE.
@CONFERENCE{Carli2015967, author = {Carli, Raffaele and Dotoli, Mariagrazia and Epicoco, Nicola and Angelico, Biagio and Vinciullo, Antonio}, title = {Automated evaluation of urban traffic congestion using bus as a probe}, year = {2015}, journal = {IEEE International Conference on Automation Science and Engineering}, volume = {2015-October}, pages = {967 – 972}, doi = {10.1109/CoASE.2015.7294224}, url = {https://www.scopus.com/inward/record.uri?eid=2-s2.0-84952762623&doi=10.1109%2fCoASE.2015.7294224&partnerID=40&md5=c2588eba274a644410a09da25d3699b5}, abstract = {This paper presents an algorithm for the automated analysis and evaluation of vehicular traffic congestion in urban areas. The proposed approach is based on the concept of bus as a probe and makes use of GPS-generated data provided by a local transit bus tracking system. Archived GPS pulses are analyzed offline to extract valuable indices related to general urban traffic characteristics and aimed at generating a detailed view of the urban roads congestion. This information is useful both for policy makers, to effectively address the management of sustainable mobility in urban areas, and for citizens, to acquire awareness about congestion times and location zones. The presented algorithm is applied to a part of the urban road network of the municipality of Bari (Italy). © 2015 IEEE.}, keywords = {Algorithms; Automation; Buses; Motor transportation; Probes; Automated analysis; Automated evaluation; Policy makers; Sustainable mobility; Tracking system; Urban road networks; Urban traffic; Urban traffic congestion; Traffic congestion}, type = {Conference paper}, publication_stage = {Final}, source = {Scopus}, note = {Cited by: 32} }
- Carli, R., Albino, V., Dotoli, M., Mummolo, G. & Savino, M. (2015) A dashboard and decision support tool for the energy governance of smart cities IN 2015 IEEE Workshop on Environmental, Energy, and Structural Monitoring Systems, EESMS 2015 – Proceedings., 23 – 28. doi:10.1109/EESMS.2015.7175846
[BibTeX] [Abstract] [Download PDF]The paper addresses the findings of the research activities conducted in the framework of the RES NOVAE project for the design and development of the Urban Control Center (UCC), a control room of the smart city that allows the Public Administration to analyze the city dynamics and citizens to receive information on the performance of urban infrastructure and services. With a specific focus on energy efficiency and environmental sustainability, we present the architecture of an innovative dashboard and decision support tool for efficient urban governance. We investigate solutions to effectively measure the city energy performance and proficiently support the decision maker in determining the optimal action plan for implementing smartness strategies in the city energy governance. © 2015 IEEE.
@CONFERENCE{Carli201523, author = {Carli, Raffaele and Albino, Vito and Dotoli, Mariagrazia and Mummolo, Giovanni and Savino, Mario}, title = {A dashboard and decision support tool for the energy governance of smart cities}, year = {2015}, journal = {2015 IEEE Workshop on Environmental, Energy, and Structural Monitoring Systems, EESMS 2015 - Proceedings}, pages = {23 – 28}, doi = {10.1109/EESMS.2015.7175846}, url = {https://www.scopus.com/inward/record.uri?eid=2-s2.0-84950984321&doi=10.1109%2fEESMS.2015.7175846&partnerID=40&md5=675d5e25da8794e638be9ff52797dd47}, abstract = {The paper addresses the findings of the research activities conducted in the framework of the RES NOVAE project for the design and development of the Urban Control Center (UCC), a control room of the smart city that allows the Public Administration to analyze the city dynamics and citizens to receive information on the performance of urban infrastructure and services. With a specific focus on energy efficiency and environmental sustainability, we present the architecture of an innovative dashboard and decision support tool for efficient urban governance. We investigate solutions to effectively measure the city energy performance and proficiently support the decision maker in determining the optimal action plan for implementing smartness strategies in the city energy governance. © 2015 IEEE.}, author_keywords = {decision support system; energy efficiency; indicators dashboard; information and communication technologies; management; monitoring; multi-attribute analysis; multi-objective optimization; optimization; smart cities}, keywords = {Artificial intelligence; Decision making; Decision support systems; Information management; Management; Monitoring; Multiobjective optimization; Optimization; Public administration; Sustainable development; Decision support tools; Design and Development; Environmental sustainability; Information and Communication Technologies; Multi-attribute analysis; Research activities; Smart cities; Urban infrastructure; Energy efficiency}, type = {Conference paper}, publication_stage = {Final}, source = {Scopus}, note = {Cited by: 10} }
- Carli, R. & Dotoli, M. (2015) A decentralized resource allocation approach for sharing renewable energy among interconnected smart homes IN Proceedings of the IEEE Conference on Decision and Control., 5903 – 5908. doi:10.1109/CDC.2015.7403147
[BibTeX] [Abstract] [Download PDF]The paper deals with the scheduling of energy activities of a group of interconnected users that buy energy from a producer and share a renewable energy source. The scheduling problem is stated and solved with a twofold goal. First, the model is formulated to ensure social welfare-optimal allocation of the energy produced from the shared renewable energy generator. Second, the model aims at cost-optimal planning of users’ controllable appliances taking into account a realistic time-varying quadratic pricing of the energy bought from the distribution network. The solution approach relies on a decentralized optimization algorithm that is composed by a two-level iterative procedure combining Gauss-Seidel decomposition with competitive game formulation. A case study simulated in different scenarios demonstrates that the approach allows exploiting the potential of renewable energy sources’ sharing to reduce individual users’ energy consumption costs, limiting the peak average ratio of energy profiles and complying with the customer’s energy needs. © 2015 IEEE.
@CONFERENCE{Carli20155903, author = {Carli, Raffaele and Dotoli, Mariagrazia}, title = {A decentralized resource allocation approach for sharing renewable energy among interconnected smart homes}, year = {2015}, journal = {Proceedings of the IEEE Conference on Decision and Control}, volume = {54rd IEEE Conference on Decision and Control,CDC 2015}, pages = {5903 – 5908}, doi = {10.1109/CDC.2015.7403147}, url = {https://www.scopus.com/inward/record.uri?eid=2-s2.0-84962024908&doi=10.1109%2fCDC.2015.7403147&partnerID=40&md5=194027b41fd3ace430f8f40254ace4de}, abstract = {The paper deals with the scheduling of energy activities of a group of interconnected users that buy energy from a producer and share a renewable energy source. The scheduling problem is stated and solved with a twofold goal. First, the model is formulated to ensure social welfare-optimal allocation of the energy produced from the shared renewable energy generator. Second, the model aims at cost-optimal planning of users' controllable appliances taking into account a realistic time-varying quadratic pricing of the energy bought from the distribution network. The solution approach relies on a decentralized optimization algorithm that is composed by a two-level iterative procedure combining Gauss-Seidel decomposition with competitive game formulation. A case study simulated in different scenarios demonstrates that the approach allows exploiting the potential of renewable energy sources' sharing to reduce individual users' energy consumption costs, limiting the peak average ratio of energy profiles and complying with the customer's energy needs. © 2015 IEEE.}, author_keywords = {Energy consumption; Games; Home appliances; Optimization; Pricing; Renewable energy sources; Resource management}, keywords = {Automation; Costs; Domestic appliances; Economics; Energy utilization; Intelligent buildings; Iterative methods; Natural resources; Optimization; Resource allocation; Scheduling; Decentralized optimization; Decentralized resource allocation; Games; Optimal allocation; Renewable energies; Renewable energy generators; Renewable energy source; Resource management; Renewable energy resources}, type = {Conference paper}, publication_stage = {Final}, source = {Scopus}, note = {Cited by: 37} }
- Carli, R., Dotoli, M., Pellegrino, R. & Ranieri, L. (2015) Using multi-objective optimization for the integrated energy efficiency improvement of a smart city public buildings’ portfolio IN IEEE International Conference on Automation Science and Engineering., 21 – 26. doi:10.1109/CoASE.2015.7294035
[BibTeX] [Abstract] [Download PDF]The paper presents a multi-objective optimization algorithm to improve in an integrated and holistic way the building stock energy efficiency, sustainability, and comfort, while efficiently allocating the available budget to the buildings. The developed algorithm determines a set of optimal energy retrofit plans for a portfolio of public buildings in a smart city. An existing stock of public buildings located in the municipality of Bari, Italy is used as case study. The application results demonstrate that the developed algorithm is an effective support tool for the smart city governance in enhancing the energy efficiency performance of a stock of public buildings. © 2015 IEEE.
@CONFERENCE{Carli201521, author = {Carli, Raffaele and Dotoli, Mariagrazia and Pellegrino, Roberta and Ranieri, Luigi}, title = {Using multi-objective optimization for the integrated energy efficiency improvement of a smart city public buildings' portfolio}, year = {2015}, journal = {IEEE International Conference on Automation Science and Engineering}, volume = {2015-October}, pages = {21 – 26}, doi = {10.1109/CoASE.2015.7294035}, url = {https://www.scopus.com/inward/record.uri?eid=2-s2.0-84952780524&doi=10.1109%2fCoASE.2015.7294035&partnerID=40&md5=64470489d4914a6d949700900aa47d44}, abstract = {The paper presents a multi-objective optimization algorithm to improve in an integrated and holistic way the building stock energy efficiency, sustainability, and comfort, while efficiently allocating the available budget to the buildings. The developed algorithm determines a set of optimal energy retrofit plans for a portfolio of public buildings in a smart city. An existing stock of public buildings located in the municipality of Bari, Italy is used as case study. The application results demonstrate that the developed algorithm is an effective support tool for the smart city governance in enhancing the energy efficiency performance of a stock of public buildings. © 2015 IEEE.}, keywords = {Algorithms; Automation; Budget control; Buildings; Multiobjective optimization; Optimization; Building stocks; Energy efficiency improvements; Optimal energy; Public buildings; Smart cities; Support tool; Energy efficiency}, type = {Conference paper}, publication_stage = {Final}, source = {Scopus}, note = {Cited by: 31} }
- Carli, R., Dotoli, M. & Pellegrino, R. (2015) ICT and optimization for the energy management of smart cities: The street lighting decision panel IN IEEE International Conference on Emerging Technologies and Factory Automation, ETFA.. doi:10.1109/ETFA.2015.7301435
[BibTeX] [Abstract] [Download PDF]The paper addresses the emerging need for tools devoted to the energy governance of smart cities. We propose a hierarchical decision process that supports the energy manager in governing the smart city while addressing different urban sectors with an integrated, structured, and transparent planning. Starting from the urban control center proposed in a previous contribution for the urban energy management, a hierarchical strategic decision structure is proposed. More in detail, a two-level decentralized programming model integrates several decision making units (decision panels), each focusing on the energy optimization of a specific urban subsystem. We focus on the presentation of the street lighting decision panel and on its application to the energy management of the public lighting of the city of Bari (Italy), where a smart city program has recently been launched. © 2015 IEEE.
@CONFERENCE{Carli2015, author = {Carli, Raffaele and Dotoli, Mariagrazia and Pellegrino, Roberta}, title = {ICT and optimization for the energy management of smart cities: The street lighting decision panel}, year = {2015}, journal = {IEEE International Conference on Emerging Technologies and Factory Automation, ETFA}, volume = {2015-October}, doi = {10.1109/ETFA.2015.7301435}, url = {https://www.scopus.com/inward/record.uri?eid=2-s2.0-84952882893&doi=10.1109%2fETFA.2015.7301435&partnerID=40&md5=012ab885ea35346c5c280804295955c9}, abstract = {The paper addresses the emerging need for tools devoted to the energy governance of smart cities. We propose a hierarchical decision process that supports the energy manager in governing the smart city while addressing different urban sectors with an integrated, structured, and transparent planning. Starting from the urban control center proposed in a previous contribution for the urban energy management, a hierarchical strategic decision structure is proposed. More in detail, a two-level decentralized programming model integrates several decision making units (decision panels), each focusing on the energy optimization of a specific urban subsystem. We focus on the presentation of the street lighting decision panel and on its application to the energy management of the public lighting of the city of Bari (Italy), where a smart city program has recently been launched. © 2015 IEEE.}, author_keywords = {Cities and towns; Decision making; Lighting; Optimization; Smart cities}, keywords = {Application programs; Energy management; Factory automation; Lighting; Optimization; Street lighting; Cities and towns; Decision making unit; Energy optimization; Hierarchical decisions; ITS applications; Programming models; Smart cities; Strategic decisions; Decision making}, type = {Conference paper}, publication_stage = {Final}, source = {Scopus}, note = {Cited by: 19} }
2014
- Carli, R. & Dotoli, M. (2014) Energy scheduling of a smart home under nonlinear pricing IN Proceedings of the IEEE Conference on Decision and Control., 5648 – 5653. doi:10.1109/CDC.2014.7040273
[BibTeX] [Abstract] [Download PDF]The paper focuses on the scheduling of energy activities in smart homes equipped with controllable electrical appliances, renewable energy sources, dispatchable energy generators, and energy storage systems. We formulate a mixed integer quadratic programming energy scheduling algorithm for cost minimization under nonlinear pricing. The scheduling technique manages the use of electrical appliances, plans the energy production and supplying, and programs the storage systems charging/discharging. A case study simulated in different scenarios demonstrates that the approach allows full exploitation of the potential of local energy generation and storage to reduce the individual user energy consumption costs, while complying with the customer energy needs. © 2014 IEEE.
@CONFERENCE{Carli20145648, author = {Carli, Raffaele and Dotoli, Mariagrazia}, title = {Energy scheduling of a smart home under nonlinear pricing}, year = {2014}, journal = {Proceedings of the IEEE Conference on Decision and Control}, volume = {2015-February}, number = {February}, pages = {5648 – 5653}, doi = {10.1109/CDC.2014.7040273}, url = {https://www.scopus.com/inward/record.uri?eid=2-s2.0-84961994657&doi=10.1109%2fCDC.2014.7040273&partnerID=40&md5=55539508a30611826d5d2e0f0b11c853}, abstract = {The paper focuses on the scheduling of energy activities in smart homes equipped with controllable electrical appliances, renewable energy sources, dispatchable energy generators, and energy storage systems. We formulate a mixed integer quadratic programming energy scheduling algorithm for cost minimization under nonlinear pricing. The scheduling technique manages the use of electrical appliances, plans the energy production and supplying, and programs the storage systems charging/discharging. A case study simulated in different scenarios demonstrates that the approach allows full exploitation of the potential of local energy generation and storage to reduce the individual user energy consumption costs, while complying with the customer energy needs. © 2014 IEEE.}, keywords = {Automation; Costs; Energy utilization; Integer programming; Intelligent buildings; Quadratic programming; Renewable energy resources; Scheduling; Dispatchable energies; Electrical appliances; Energy productions; Energy storage systems; Mixed integer quadratic programming; Non-linear pricing; Renewable energy source; Scheduling techniques; Scheduling algorithms}, type = {Conference paper}, publication_stage = {Final}, source = {Scopus}, note = {Cited by: 42} }
- Carli, R., Deidda, P., Dotoli, M. & Pellegrino, R. (2014) An urban control center for the energy governance of a smart city IN 19th IEEE International Conference on Emerging Technologies and Factory Automation, ETFA 2014.. doi:10.1109/ETFA.2014.7005155
[BibTeX] [Abstract] [Download PDF]The paper addresses the emerging need of providing urban managers with tools for energy governance of smart cities. We present the architecture of a decision support system called Urban Control Center (UCC). The UCC measures the city energy performance and supports the decision maker in determining the optimal action plan for implementing smartness strategies in the city energy governance. To this aim, the UCC relies on a two-level decentralized programming model that integrates several decision making units (decision panels), each focusing on the energy optimization of a specific urban subsystem. © 2014 IEEE.
@CONFERENCE{Carli2014, author = {Carli, Raffaele and Deidda, Paolo and Dotoli, Mariagrazia and Pellegrino, Roberta}, title = {An urban control center for the energy governance of a smart city}, year = {2014}, journal = {19th IEEE International Conference on Emerging Technologies and Factory Automation, ETFA 2014}, doi = {10.1109/ETFA.2014.7005155}, url = {https://www.scopus.com/inward/record.uri?eid=2-s2.0-84946686880&doi=10.1109%2fETFA.2014.7005155&partnerID=40&md5=7ae375bfea52c5c6212722aca93bc04d}, abstract = {The paper addresses the emerging need of providing urban managers with tools for energy governance of smart cities. We present the architecture of a decision support system called Urban Control Center (UCC). The UCC measures the city energy performance and supports the decision maker in determining the optimal action plan for implementing smartness strategies in the city energy governance. To this aim, the UCC relies on a two-level decentralized programming model that integrates several decision making units (decision panels), each focusing on the energy optimization of a specific urban subsystem. © 2014 IEEE.}, keywords = {Artificial intelligence; Decision support systems; Factory automation; Control center; Decision makers; Decision making unit; Energy optimization; Energy performance; Optimal actions; Programming models; Smart cities; Decision making}, type = {Conference paper}, publication_stage = {Final}, source = {Scopus}, note = {Cited by: 14} }
2013
- Carli, R., Dotoli, M., Pellegrino, R. & Ranieri, L. (2013) Measuring and managing the smartness of cities: A framework for classifying performance indicators IN Proceedings – 2013 IEEE International Conference on Systems, Man, and Cybernetics, SMC 2013., 1288 – 1293. doi:10.1109/SMC.2013.223
[BibTeX] [Abstract] [Download PDF]Due to the continuous increase of the world population living in cities, it is crucial to identify strategic plans and perform associated actions to make cities smarter, i.e., more operationally efficient, socially friendly, and environmentally sustainable, in a cost effective manner. To achieve these goals, emerging smart cities need to be optimally and intelligently measured, monitored, and managed. In this context the paper proposes the development of a framework for classifying performance indicators of a smart city. It is based on two dimensions: The degree of objectivity of observed variables and the level of technological advancement for data collection. The paper shows an application of the presented framework to the case of the Bari municipality (Italy). © 2013 IEEE.
@CONFERENCE{Carli20131288, author = {Carli, Raffaele and Dotoli, Mariagrazia and Pellegrino, Roberta and Ranieri, Luigi}, title = {Measuring and managing the smartness of cities: A framework for classifying performance indicators}, year = {2013}, journal = {Proceedings - 2013 IEEE International Conference on Systems, Man, and Cybernetics, SMC 2013}, pages = {1288 – 1293}, doi = {10.1109/SMC.2013.223}, url = {https://www.scopus.com/inward/record.uri?eid=2-s2.0-84893629343&doi=10.1109%2fSMC.2013.223&partnerID=40&md5=2e7a1d0751ad2b7391780dc51c8b26b0}, abstract = {Due to the continuous increase of the world population living in cities, it is crucial to identify strategic plans and perform associated actions to make cities smarter, i.e., more operationally efficient, socially friendly, and environmentally sustainable, in a cost effective manner. To achieve these goals, emerging smart cities need to be optimally and intelligently measured, monitored, and managed. In this context the paper proposes the development of a framework for classifying performance indicators of a smart city. It is based on two dimensions: The degree of objectivity of observed variables and the level of technological advancement for data collection. The paper shows an application of the presented framework to the case of the Bari municipality (Italy). © 2013 IEEE.}, author_keywords = {Information and communication technologies; Management; Monitoring; Smart cities; Smartness indicators}, keywords = {Cybernetics; Electronic commerce; Information technology; Management; Monitoring; Cost effective; Data collection; Information and Communication Technologies; Performance indicators; Smart cities; Strategic plan; Technological advancement; World population; Benchmarking}, type = {Conference paper}, publication_stage = {Final}, source = {Scopus}, note = {Cited by: 91} }